HAC Explore: Accelerating Exploration with Hierarchical Reinforcement Learning
arxiv(2021)
摘要
Sparse rewards and long time horizons remain challenging for reinforcement learning algorithms. Exploration bonuses can help in sparse reward settings by encouraging agents to explore the state space, while hierarchical approaches can assist with long-horizon tasks by decomposing lengthy tasks into shorter subtasks. We propose HAC Explore (HACx), a new method that combines these approaches by integrating the exploration bonus method Random Network Distillation (RND) into the hierarchical approach Hierarchical Actor-Critic (HAC). HACx outperforms either component method on its own, as well as an existing approach to combining hierarchy and exploration, in a set of difficult simulated robotics tasks. HACx is the first RL method to solve a sparse reward, continuous-control task that requires over 1,000 actions.
更多查看译文
关键词
hierarchical reinforcement learning,exploration,reinforcement learning
AI 理解论文
溯源树
样例
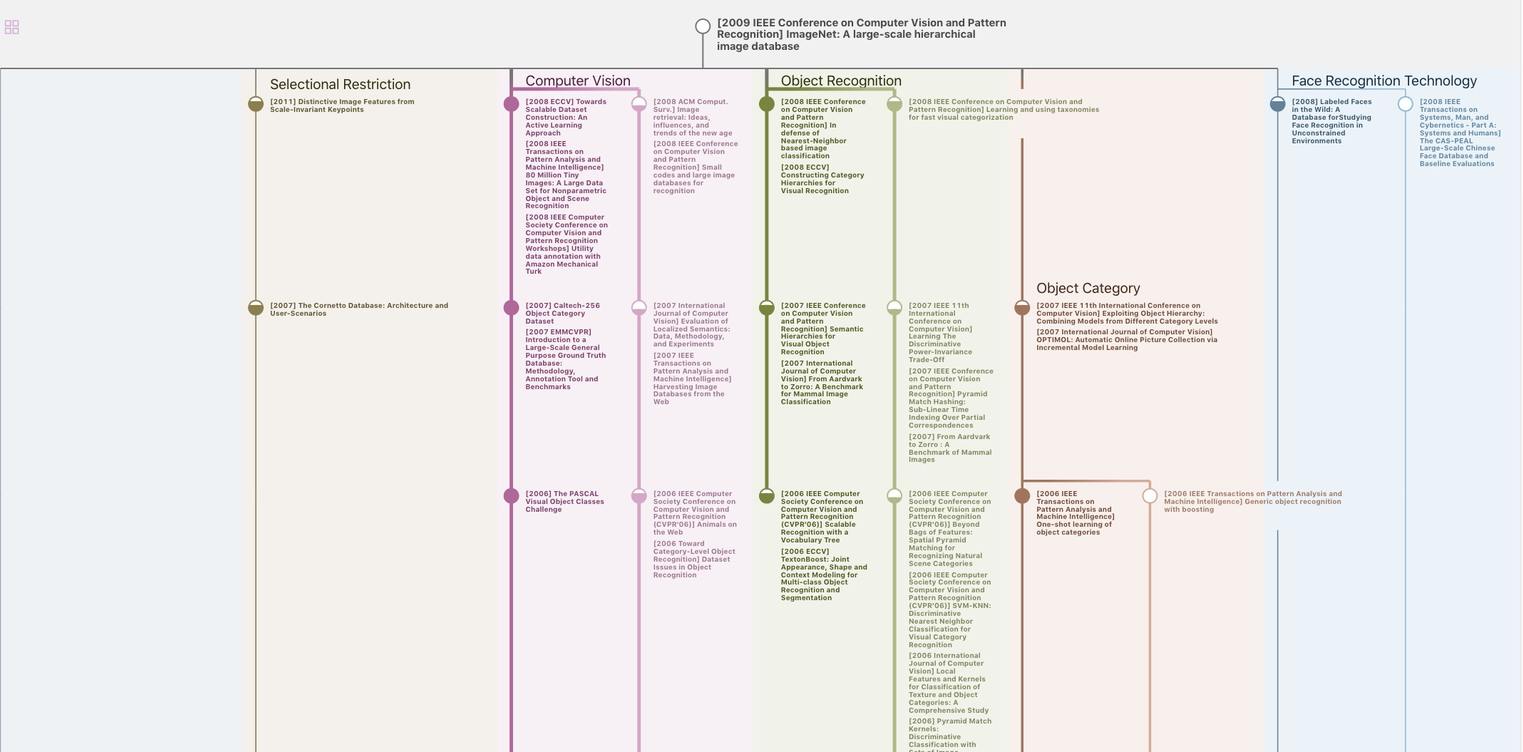
生成溯源树,研究论文发展脉络
Chat Paper
正在生成论文摘要