Review and Comparative Analysis of Machine Learning-based Predic-tors for Predicting and Analyzing Anti-angiogenic Peptides
CURRENT MEDICINAL CHEMISTRY(2022)
摘要
Cancer is one of the leading causes of death worldwide and the underlying angiogenesis represents one of the hallmarks of cancer. Efforts are already under way for the discovery of anti-angiogenic peptides (AAPs) as a promising therapeutic route, which tackle the formation of new blood vessels. As such, the identification of AAPs constitutes a viable path for understanding their mechanistic properties pertinent for the discovery of new anti-cancer drugs. In spite of the abundance of peptide sequences in public databases, experimental efforts in the identification of anti-angiogenic peptides have progressed very slowly owing to high expenditures and laborious nature. Owing to its inherent ability to make sense of large volumes of data, machine learning (ML) represents a lucrative technique that can be harnessed for peptide-based drug discovery. In this review, we conducted a comprehensive and comparative analysis of ML-based AAP predictors in terms of their employed feature descriptors, ML algorithms, cross-validation methods and prediction performance. Moreover, the common framework of these AAP predictors and their inherent weaknesses are also discussed. Particularly, we explore future perspectives for improving the prediction accuracy and model interpretability, which represent an interesting avenue for overcoming some of the inherent weaknesses of existing AAP predictors. We anticipate that this review would assist researchers in the rapid screening and identification of promising AAPs for clinical use.
更多查看译文
关键词
Anti-angiogenic peptides, therapeutic peptides, classification, machine learning, feature representation, feature selection
AI 理解论文
溯源树
样例
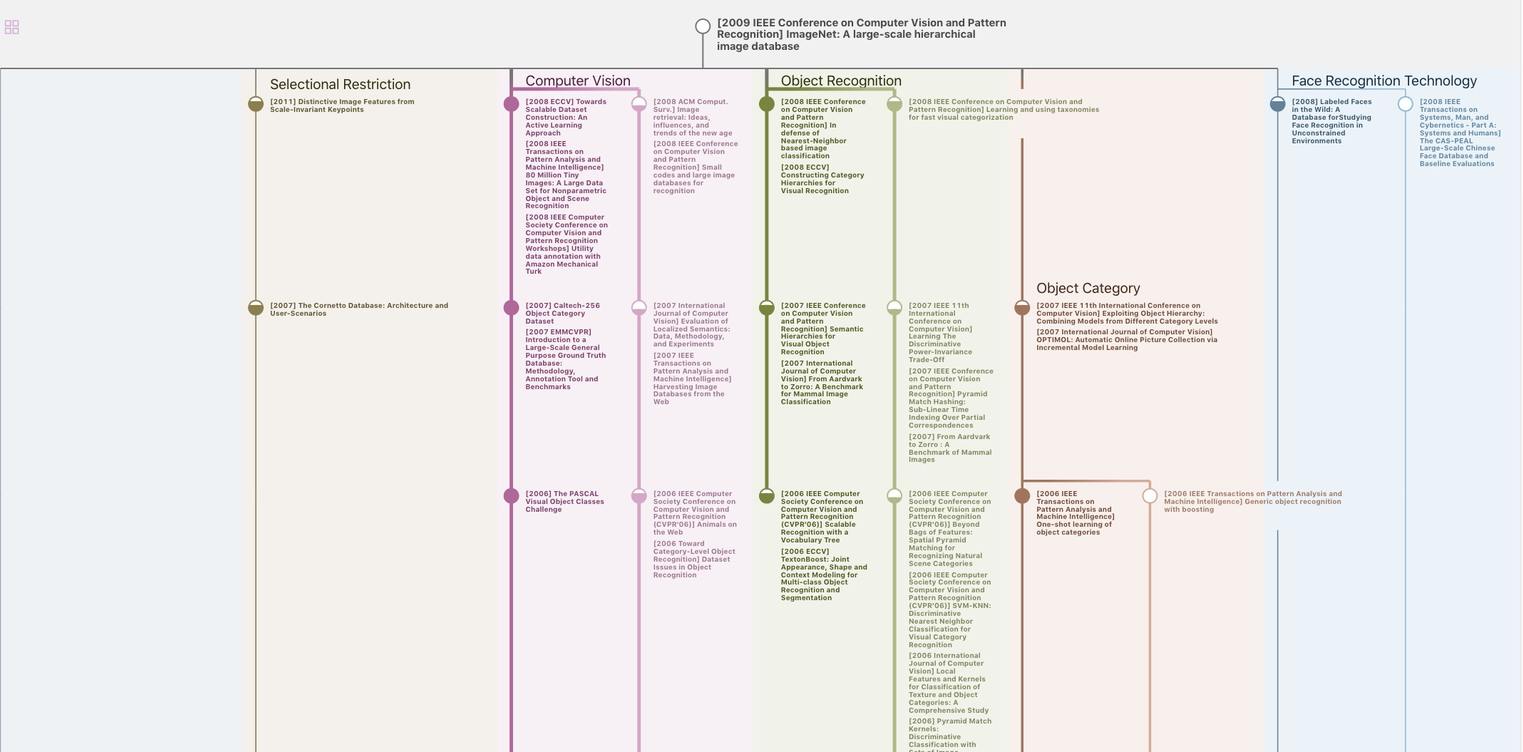
生成溯源树,研究论文发展脉络
Chat Paper
正在生成论文摘要