Representation Learning for Remote Sensing: An Unsupervised Sensor Fusion Approach
arxiv(2021)
摘要
In the application of machine learning to remote sensing, labeled data is often scarce or expensive, which impedes the training of powerful models like deep convolutional neural networks. Although unlabeled data is abundant, recent self-supervised learning approaches are ill-suited to the remote sensing domain. In addition, most remote sensing applications currently use only a small subset of the multi-sensor, multi-channel information available, motivating the need for fused multi-sensor representations. We propose a new self-supervised training objective, Contrastive Sensor Fusion, which exploits coterminous data from multiple sources to learn useful representations of every possible combination of those sources. This method uses information common across multiple sensors and bands by training a single model to produce a representation that remains similar when any subset of its input channels is used. Using a dataset of 47 million unlabeled coterminous image triplets, we train an encoder to produce semantically meaningful representations from any possible combination of channels from the input sensors. These representations outperform fully supervised ImageNet weights on a remote sensing classification task and improve as more sensors are fused. Our code is available at https://storage.cloud.google.com/public-published-datasets/csf_code.zip.
更多查看译文
关键词
remote sensing,unsupervised sensor
AI 理解论文
溯源树
样例
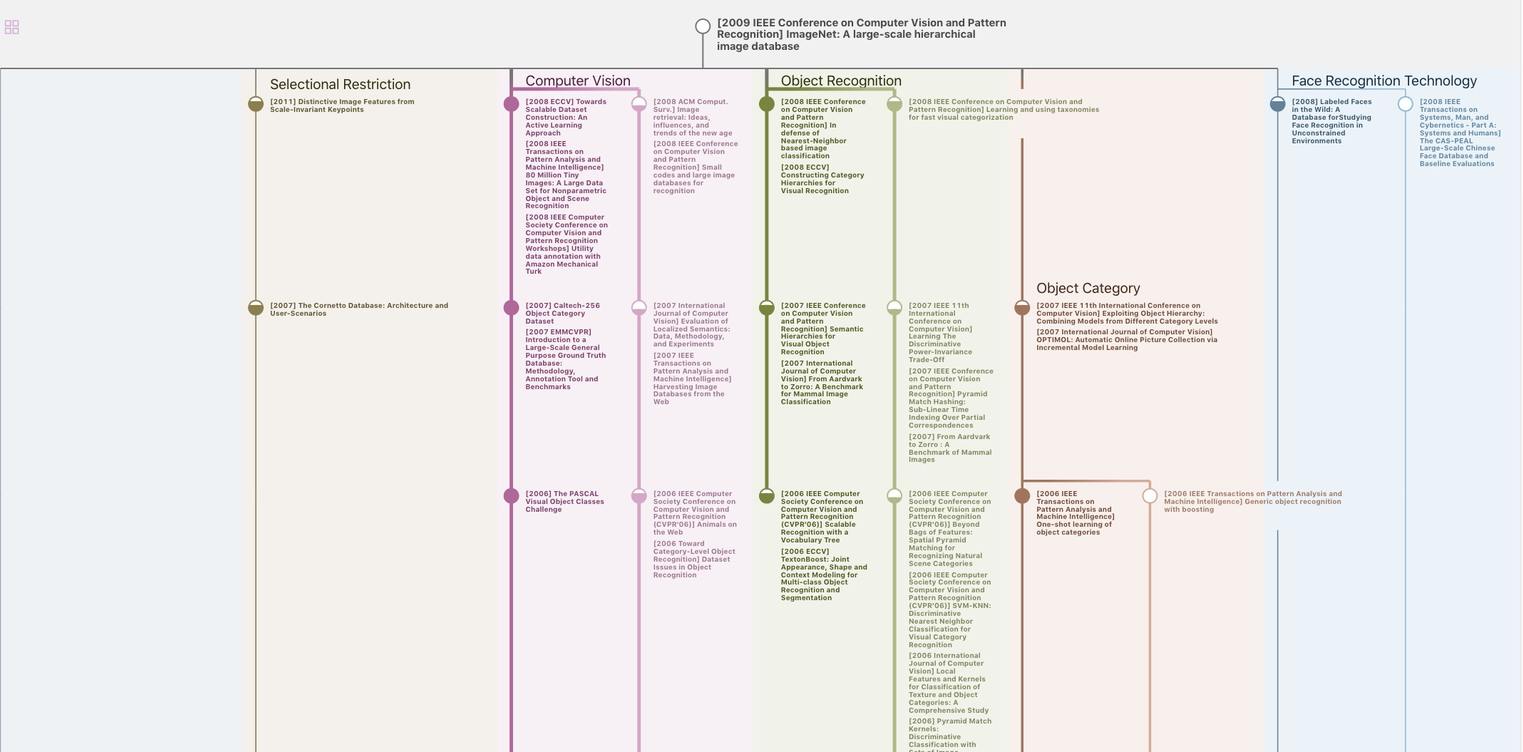
生成溯源树,研究论文发展脉络
Chat Paper
正在生成论文摘要