A Data-Driven Congestion Diffusion Model For Characterizing Traffic In Metrocity Scales
2017 IEEE INTERNATIONAL CONFERENCE ON BIG DATA (BIG DATA)(2017)
摘要
Traffic congestion is a spatio-temporal state of speeds beyond the capacity of road design and congestion may propagate through road networks. Characterizing the diffusion process is of great importance both in congestion relief and traffic condition prediction. Traffic congestion diffusion (TCD) in road networks can be observed, but literature lacks accurate models for characterizing the process. In this paper, we define a concept of Traffic Flow Influence (TFI) as a base for congestion diffusion. A TCD model is designed to characterize not only the traffic flow evolving process in time domain but also the propagation process of TFI through road networks in space domain. The model is for traffic networks in a city, which is divided into grids and each grid is modeled by traffic status of congested or smooth. Different from other diffusion models, the grid status depends on not only its current condition, but also the relative traffic flow from and to its neighbors. We use a gradient descent approach to quantify the traffic flow and TFI intensity of road networks. To the best of our knowledge, this should be the first model for a metro-city scale. The TCD model with TFI is able to predict grid status with an accuracy as high as 89%. Experimental results based on real-world taxi trajectory data in a metro-city show that the TCD approach performs best in comparison with its competitors.
更多查看译文
关键词
Traffic Flow Influence, TFI Intensity, Traffic Congestion Diffusion, Data-driven Approach
AI 理解论文
溯源树
样例
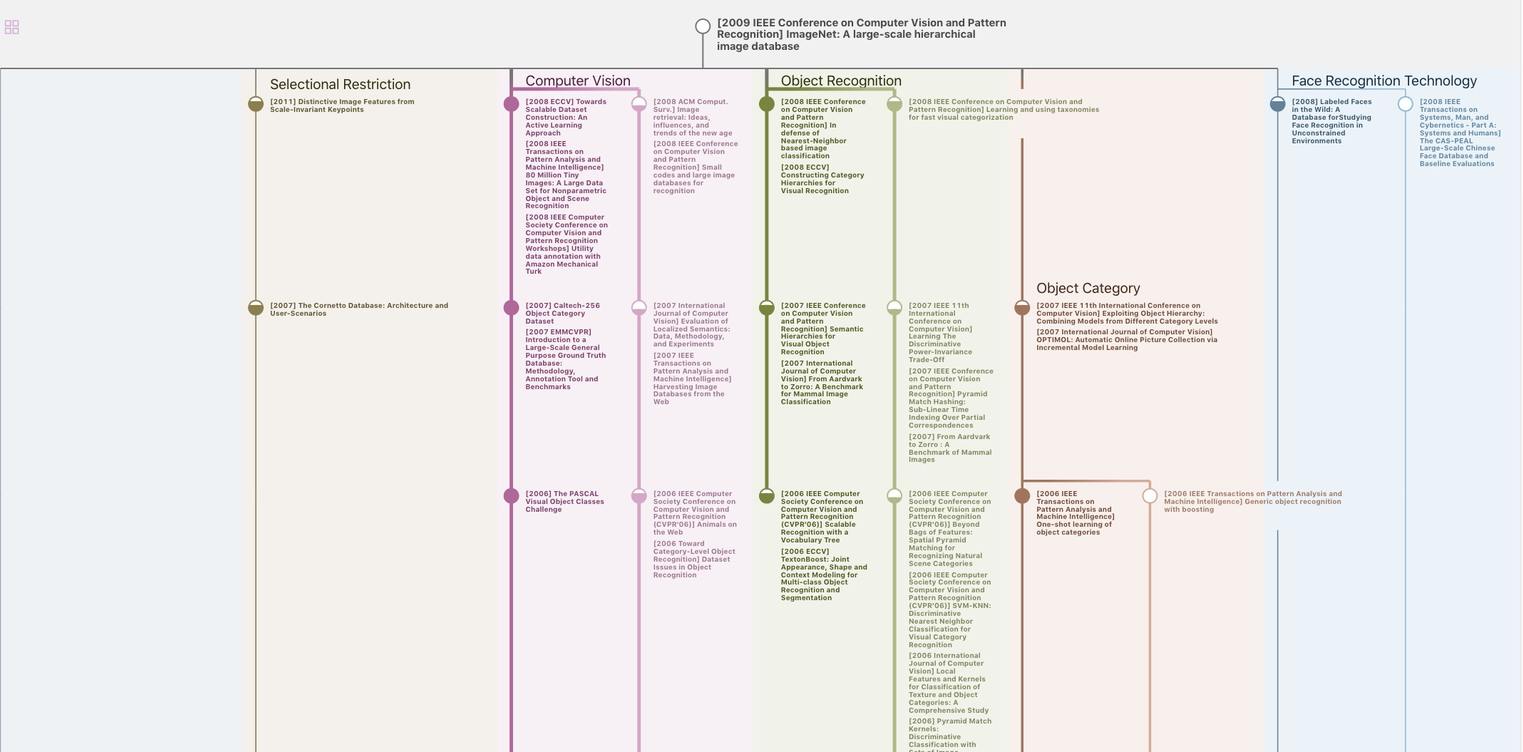
生成溯源树,研究论文发展脉络
Chat Paper
正在生成论文摘要