Winning the NIST Contest: A scalable and general approach to differentially private synthetic data.
J. Priv. Confidentiality(2021)
摘要
We propose a general approach for differentially private synthetic data generation, that consists of three steps: (1) select a collection of low-dimensional marginals, (2) measure those marginals with a noise addition mechanism, and (3) generate synthetic data that preserves the measured marginals well. Central to this approach is Private-PGM, a post-processing method that is used to estimate a high-dimensional data distribution from noisy measurements of its marginals. We present two mechanisms, NIST-MST and MST, that are instances of this general approach. NIST-MST was the winning mechanism in the 2018 NIST differential privacy synthetic data competition, and MST is a new mechanism that can work in more general settings, while still performing comparably to NIST-MST. We believe our general approach should be of broad interest, and can be adopted in future mechanisms for synthetic data generation.
更多查看译文
关键词
differential privacy,synthetic data,graphical models
AI 理解论文
溯源树
样例
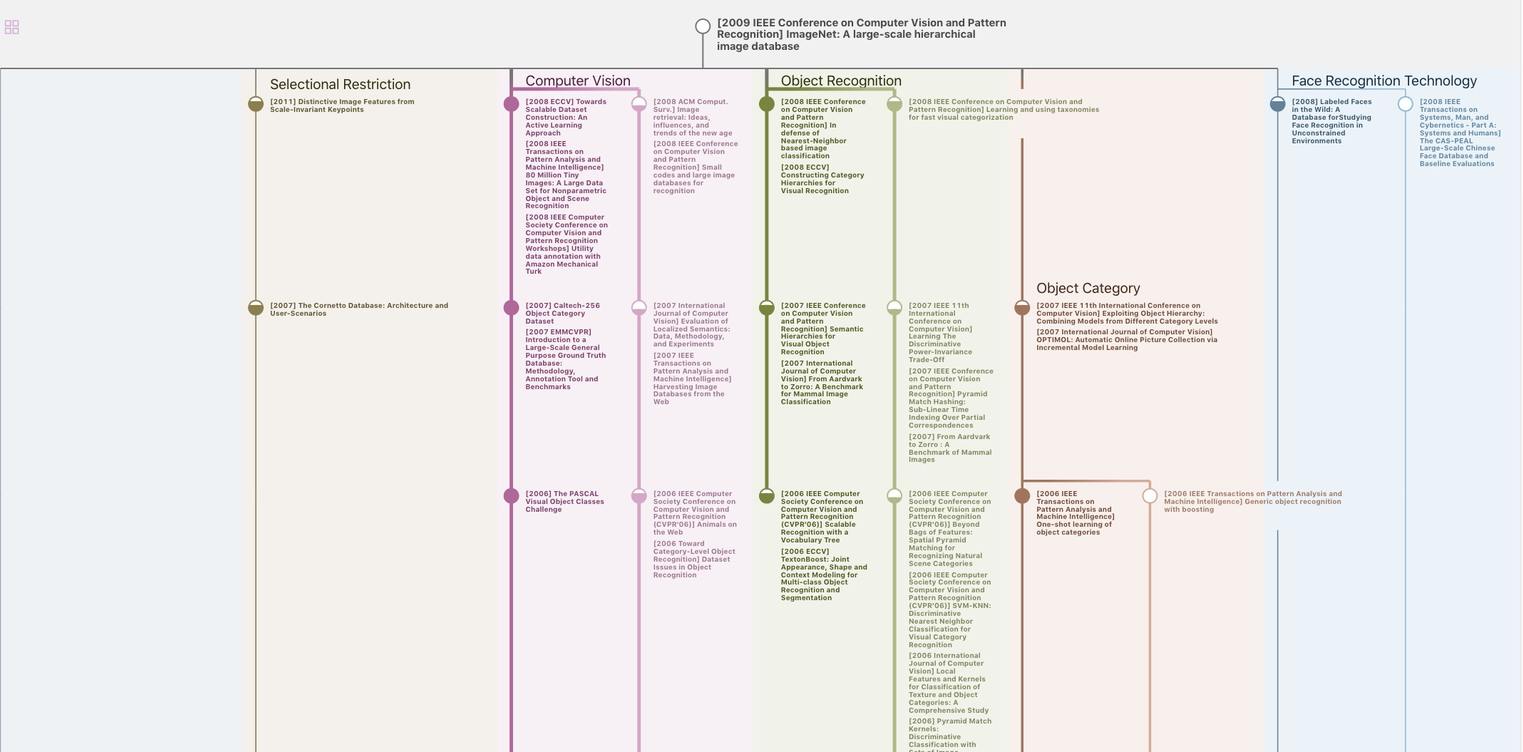
生成溯源树,研究论文发展脉络
Chat Paper
正在生成论文摘要