Correlation Clustering Reconstruction in Semi-Adversarial Models
arxiv(2021)
摘要
Correlation Clustering is an important clustering problem with many applications. We study the reconstruction version of this problem in which one is seeking to reconstruct a latent clustering that has been corrupted by random noise and adversarial modifications. Concerning the latter, we study a standard "post-adversarial" model, in which adversarial modifications come after the noise, and also introduce and analyze a "pre-adversarial" model in which adversarial modifications come before the noise. Given an input coming from such a semi-adversarial generative model, the goal is to reconstruct almost perfectly and with high probability the latent clustering. We focus on the case where the hidden clusters have equal size and show the following. In the pre-adversarial setting, spectral algorithms are optimal, in the sense that they reconstruct all the way to the information-theoretic threshold beyond which no reconstruction is possible. In contrast, in the post-adversarial setting their ability to restore the hidden clusters stops before the threshold, but the gap is optimally filled by SDP-based algorithms.
更多查看译文
关键词
correlation clustering reconstruction,models,semi-adversarial
AI 理解论文
溯源树
样例
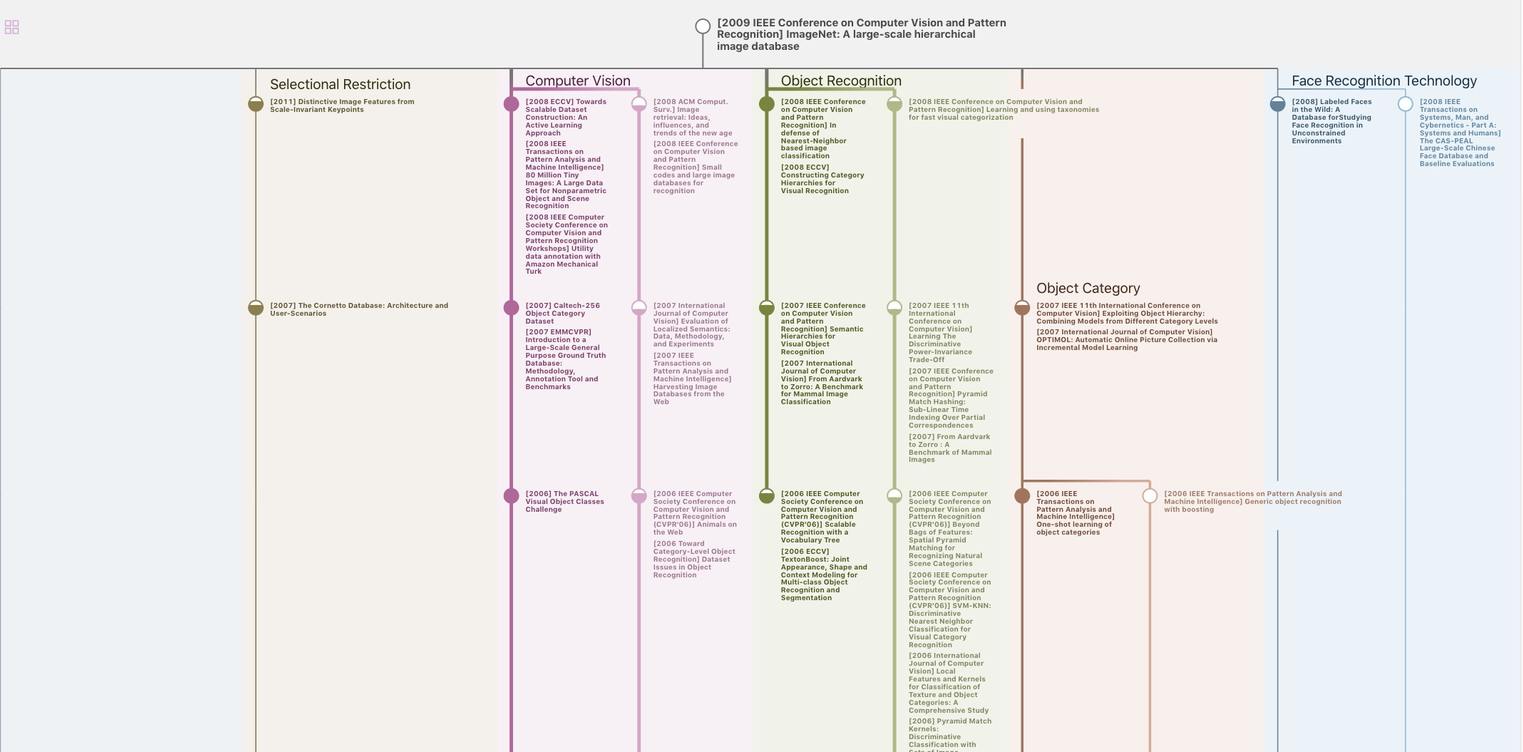
生成溯源树,研究论文发展脉络
Chat Paper
正在生成论文摘要