Towards Better Uncertainty: Iterative Training of Efficient Networks for Multitask Emotion Recognition
arxiv(2021)
摘要
When recognizing emotions, subtle nuances of emotion displays often cause ambiguity or uncertainty in emotion perception. Unfortunately, the ambiguity or uncertainty cannot be reflected in hard emotion labels. Emotion predictions with uncertainty can be useful for risk controlling, but they are relatively scarce in current deep models for emotion recognition. To address this issue, we propose to apply the multi-generational self-distillation algorithm to emotion recognition task towards better uncertainty estimation performance. We firstly use deep ensembles to capture uncertainty, as an approximation to Bayesian methods. Secondly, the deep ensemble provides soft labels to its student models, while the student models can learn from the uncertainty embedded in those soft labels. Thirdly, we iteratively train deep ensembles to further improve the performance of emotion recognition and uncertainty estimation. In the end, our algorithm results in a single student model that can estimate in-domain uncertainty and a student ensemble that can detect out-of-domain samples. We trained our Efficient Multitask Emotion Networks (EMENet) on the Aff-wild2 dataset, and conducted extensive experiments on emotion recognition and uncertainty estimation. Our algorithm gives more reliable uncertainty estimates than Temperature Scaling and Monte Carol Dropout.
更多查看译文
关键词
multitask emotion recognition,iterative training,networks
AI 理解论文
溯源树
样例
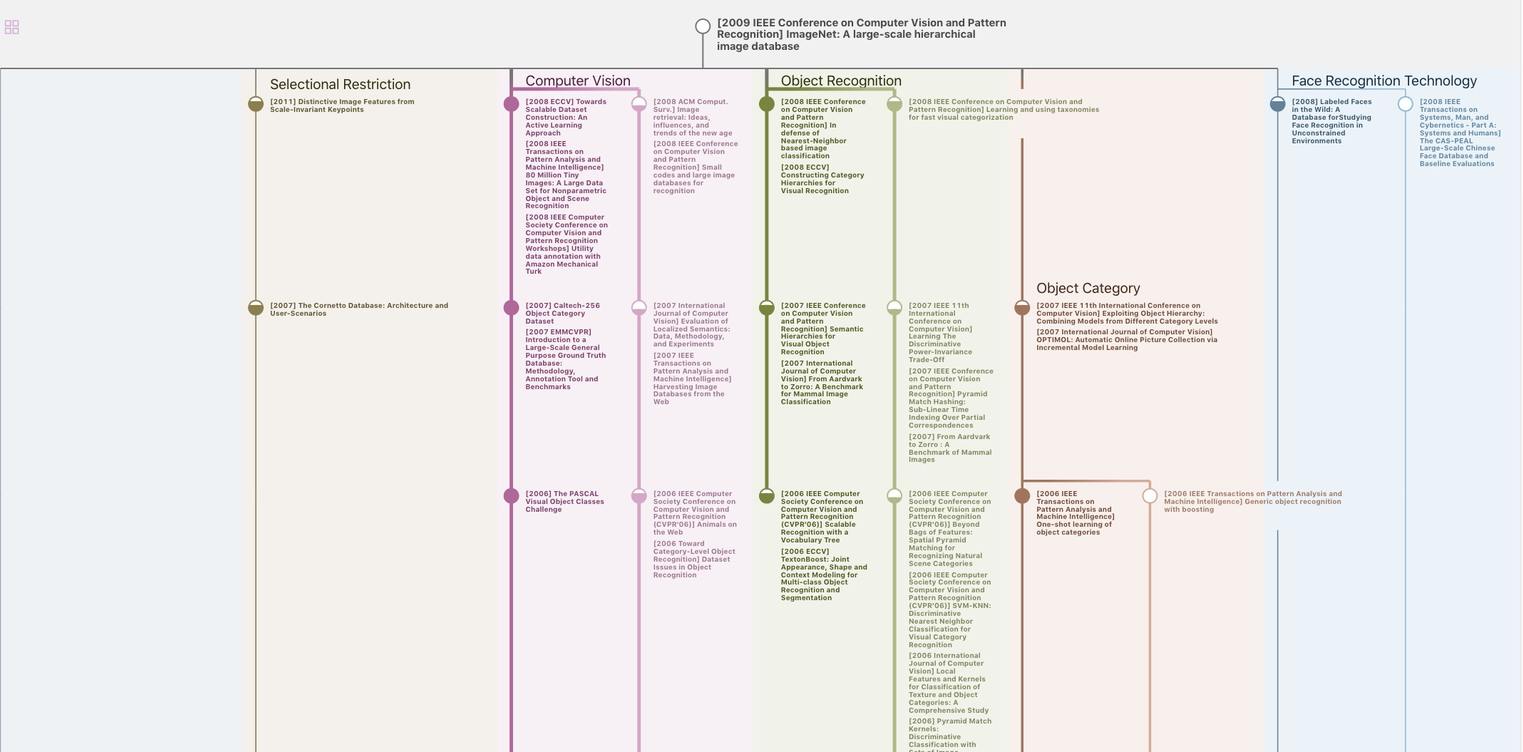
生成溯源树,研究论文发展脉络
Chat Paper
正在生成论文摘要