Scalable Bayesian Transport Maps for High-Dimensional Non-Gaussian Spatial Fields
JOURNAL OF THE AMERICAN STATISTICAL ASSOCIATION(2023)
摘要
A multivariate distribution can be described by a triangular transport map from the target distribution to a simple reference distribution. We propose Bayesian nonparametric inference on the transport map by modeling its components using Gaussian processes. This enables regularization and uncertainty quantification of the map estimation, while resulting in a closed-form and invertible posterior map. We then focus on inferring the distribution of a nonstationary spatial field from a small number of replicates. We develop specific transport-map priors that are highly flexible and are motivated by the behavior of a large class of stochastic processes. Our approach is scalable to high-dimensional distributions due to data-dependent sparsity and parallel computations. We also discuss extensions, including Dirichlet process mixtures for flexible marginals. We present numerical results to demonstrate the accuracy, scalability, and usefulness of our methods, including statistical emulation of non-Gaussian climate-model output. for this article are available online.
更多查看译文
关键词
scalable bayesian transport maps,fields,high-dimensional,non-gaussian
AI 理解论文
溯源树
样例
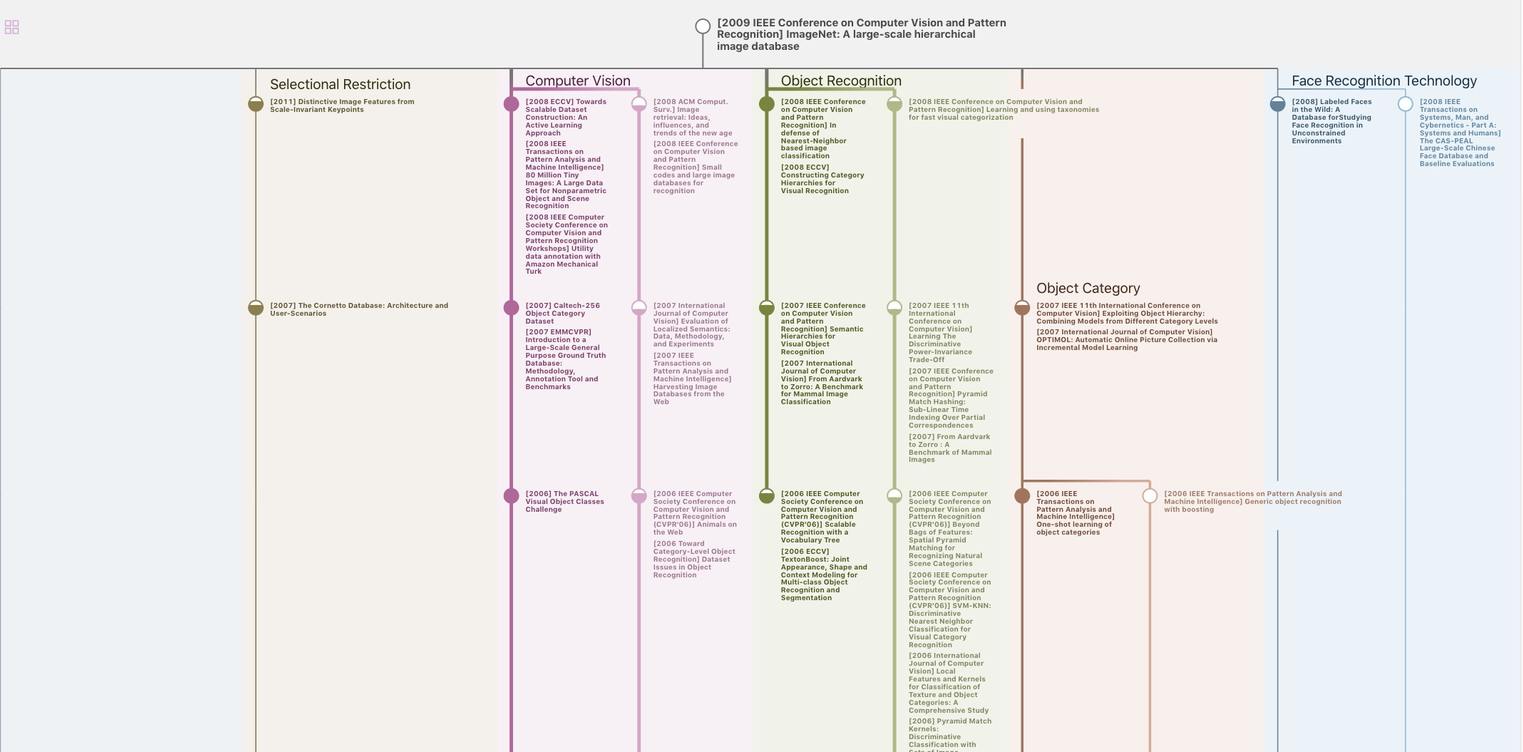
生成溯源树,研究论文发展脉络
Chat Paper
正在生成论文摘要