Generating Personalized Dialogue via Multi-Task Meta-Learning
ArXiv(2021)
摘要
Conventional approaches to personalized dialogue generation typically require a large corpus, as well as predefined persona information. However, in a real-world setting, neither a large corpus of training data nor persona information are readily available. To address these practical limitations, we propose a novel multi-task meta-learning approach which involves training a model to adapt to new personas without relying on a large corpus, or on any predefined persona information. Instead, the model is tasked with generating personalized responses based on only the dialogue context. Unlike prior work, our approach leverages on the provided persona information only during training via the introduction of an auxiliary persona reconstruction task. In this paper, we introduce 2 frameworks that adopt the proposed multi-task meta-learning approach: the Multi-Task Meta-Learning (MTML) framework, and the Alternating Multi-Task Meta-Learning (AMTML) framework. Experimental results show that utilizing MTML and AMTML results in dialogue responses with greater persona consistency.
更多查看译文
关键词
personalized dialogue,multi-task,meta-learning
AI 理解论文
溯源树
样例
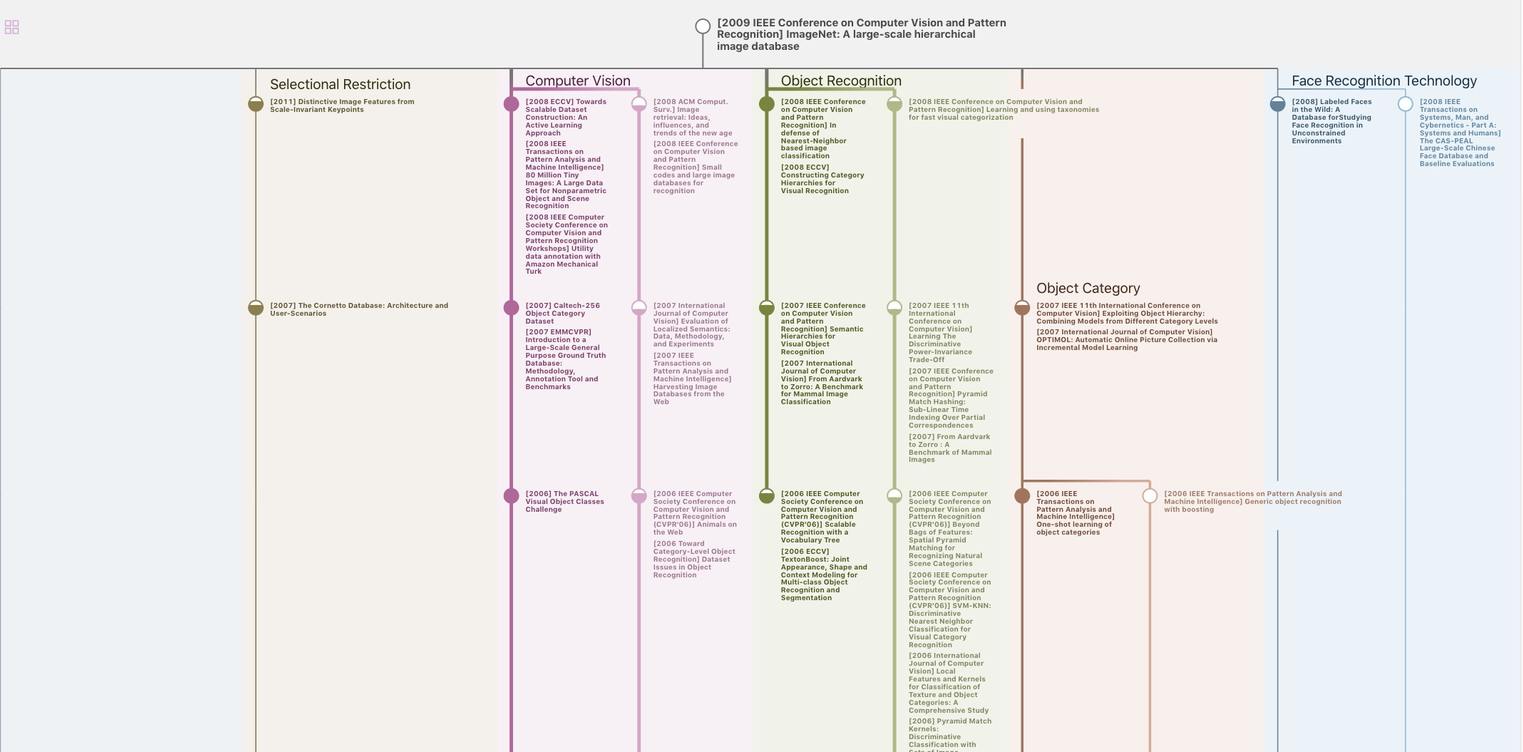
生成溯源树,研究论文发展脉络
Chat Paper
正在生成论文摘要