From Sampling to Optimization on Discrete Domains with Applications to Determinant Maximization.
user-5d4bc4a8530c70a9b361c870(2022)
摘要
We establish a connection between sampling and optimization on discrete domains. For a family of distributions $\mu$ defined on size $k$ subsets of a ground set of elements, that is closed under external fields, we show that rapid mixing of natural local random walks implies the existence of simple approximation algorithms to find $\max \mu(\cdot)$. More precisely, we show that if $t$-step down-up random walks have spectral gap at least inverse polynomially large, then $t$-step local search finds $\max \mu(\cdot)$ within a factor of $k^{O(k)}$. As the main application of our result, we show that $2$-step local search achieves a nearly-optimal $k^{O(k)}$-factor approximation for MAP inference on nonsymmetric $k$-DPPs. This is the first nontrivial multiplicative approximation algorithm for this problem. In our main technical result, we show that an exchange inequality, a concept rooted in discrete convex analysis, can be derived from fast mixing of local random walks. We further advance the state of the art on the mixing of random walks for nonsymmetric DPPs and more generally sector-stable distributions, by obtaining the tightest possible bound on the step size needed for polynomial-time mixing of random walks. We bring the step size down by a factor of $2$ compared to prior works, and consequently get a quadratic improvement on the runtime of local search steps; this improvement is potentially of independent interest in sampling applications.
更多查看译文
关键词
Inverse,Random walk,Connection (algebraic framework),Approximation algorithm,Minor (linear algebra),Convex analysis,Spectral gap,Square (algebra),Combinatorics,Computer science
AI 理解论文
溯源树
样例
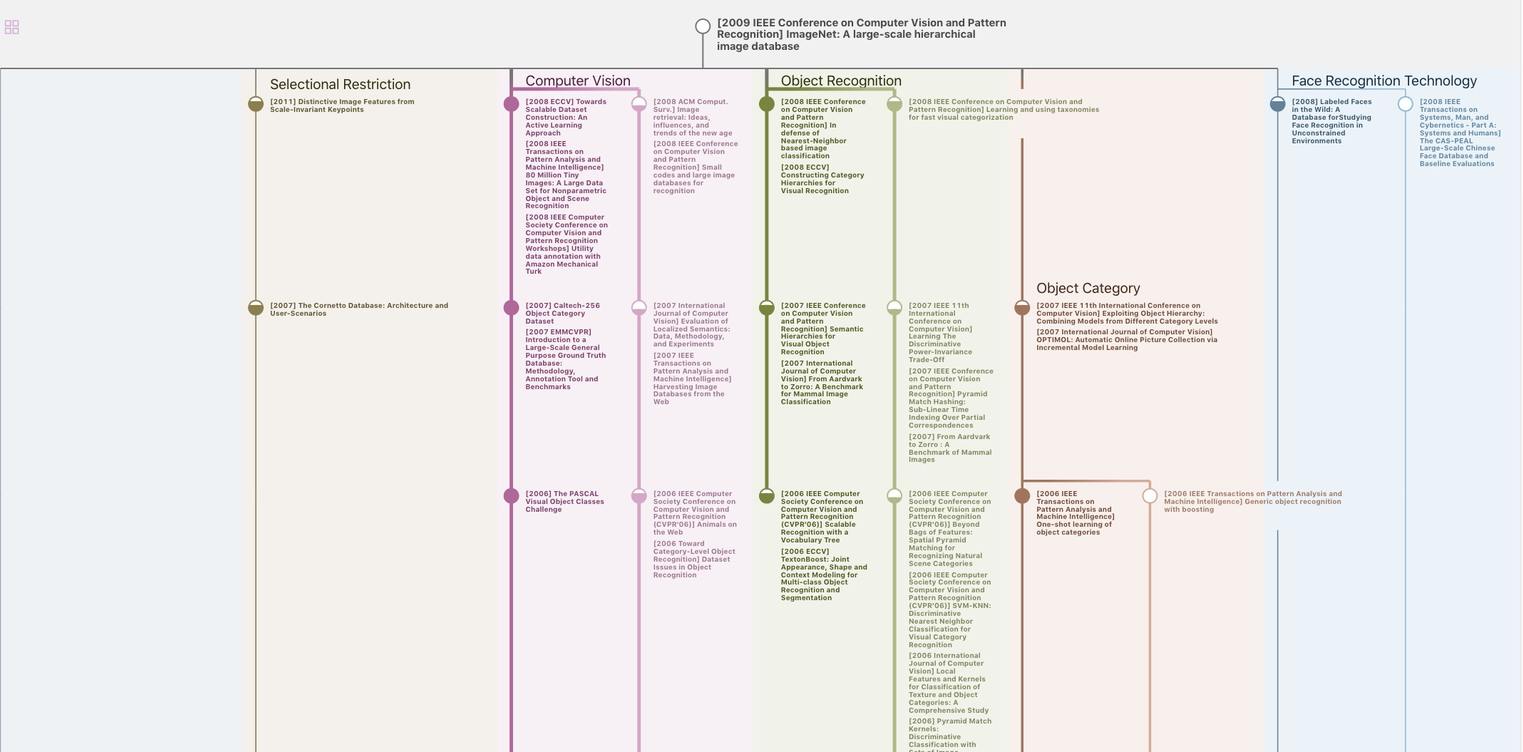
生成溯源树,研究论文发展脉络
Chat Paper
正在生成论文摘要