Machine Learning-based Slice Management in 5G Networks for Emergency Scenarios
2021 Joint European Conference on Networks and Communications & 6G Summit (EuCNC/6G Summit)(2021)
摘要
This study proposes a two-step ML-based multislice radio resource allocation framework for 5G networks, specifically for emergency scenarios and featuring a good tradeoff between complexity and performance. In the first step, call-level resource demands are predicted using supervised ML, which are then aggregated to predict slice-specific resource demands. An innovative method is included in this step to ensure the collection of representative training data for the supervised ML. In the second step, a contextual multi-armed bandit reinforcement learning model is applied to derive the resource allocation among the slices based on the slice-specific resource demand predictions. The simulation results show that the proposed framework outperforms alternative solutions in the defined utility values for priority emergency traffic at the cost of modest performance sacrifice of the background traffic.
更多查看译文
关键词
5G,network slicing,slice management,machine learning,emergency scenarios
AI 理解论文
溯源树
样例
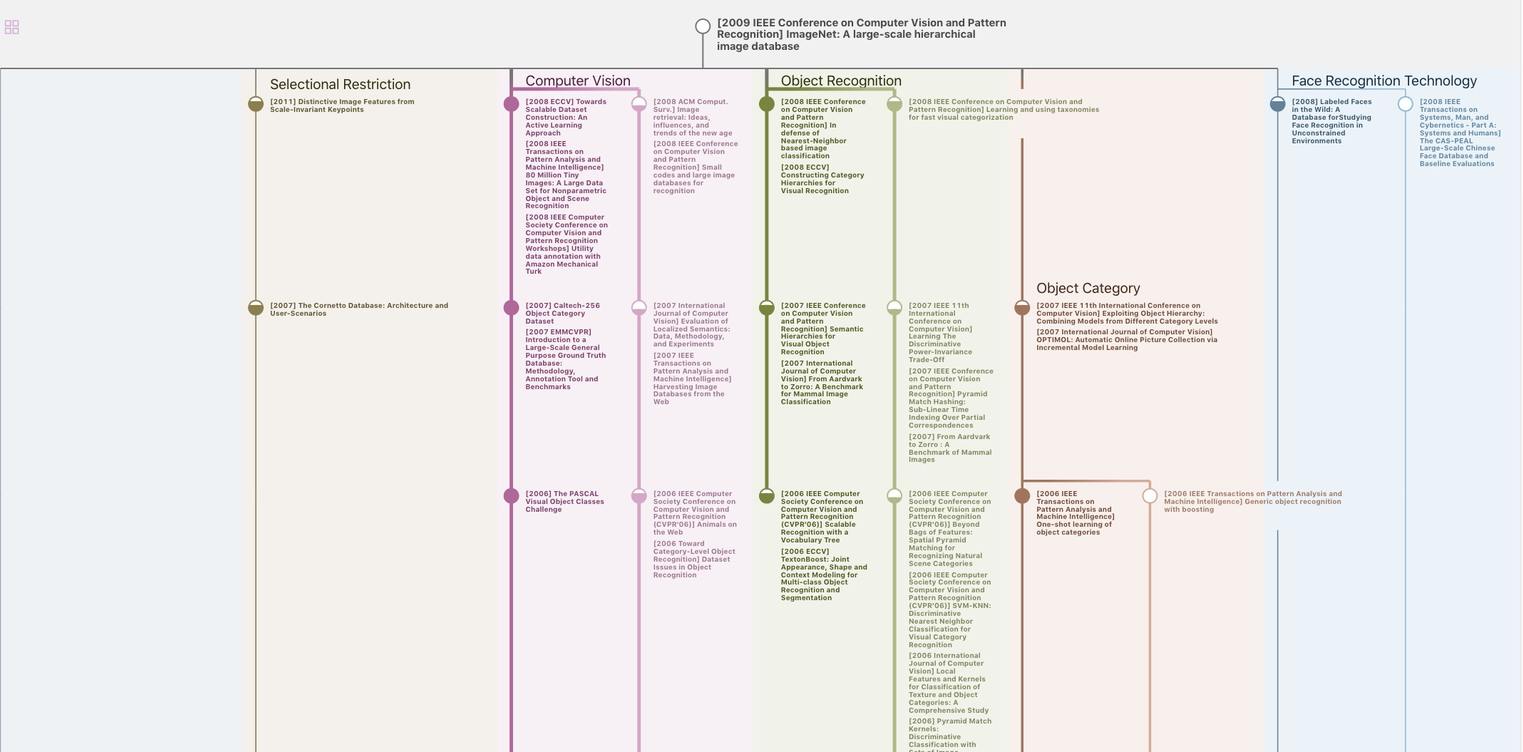
生成溯源树,研究论文发展脉络
Chat Paper
正在生成论文摘要