Predicting Drug Resistance in M. Tuberculosis Using a Long-Term Recurrent Convolutional Network
12TH ACM CONFERENCE ON BIOINFORMATICS, COMPUTATIONAL BIOLOGY, AND HEALTH INFORMATICS (ACM-BCB 2021)(2021)
摘要
Motivation: Drug resistance in Mycobacterium tuberculosis (MTB) is a growing threat to human health worldwide. One way to mitigate the risk of drug resistance is to enable clinicians to prescribe the right antibiotic drugs to each patient through methods that predict drug resistance in MTB using whole-genome sequencing (WGS) data. Existing machine learning methods for this task typically convert the WGS data from a given bacterial isolate into features corresponding to single-nucleotide polymorphisms (SNPs) or short sequence segments of a fixed length K (K-mers). Here, we introduce a gene burden-based method for predicting drug resistance in TB. We define one numerical feature per gene corresponding to the number of mutations in that gene in a given isolate. This representation greatly reduces the number of model parameters. We further propose a model architecture that considers both gene order and locality structure through a Long-term Recurrent Convolutional Network (LRCN) architecture, which combines convolutional and recurrent layers. Results: We find that using these strategies yields a substantial, statistically significant improvement over state-of-the-art methods on a large dataset of M. tuberculosis isolates, and suggest that this improvement is driven by our method's ability to account for the order of the genes in the genome and their organization into operons. Availability: The implementations of our feature preprocessing pipeline1 and our LRCN model2 are publicly available, as is our complete dataset3. Supplementary information: Additional data are available in the Supplementary Materials document4.
更多查看译文
关键词
Mycobacterium tuberculosis
AI 理解论文
溯源树
样例
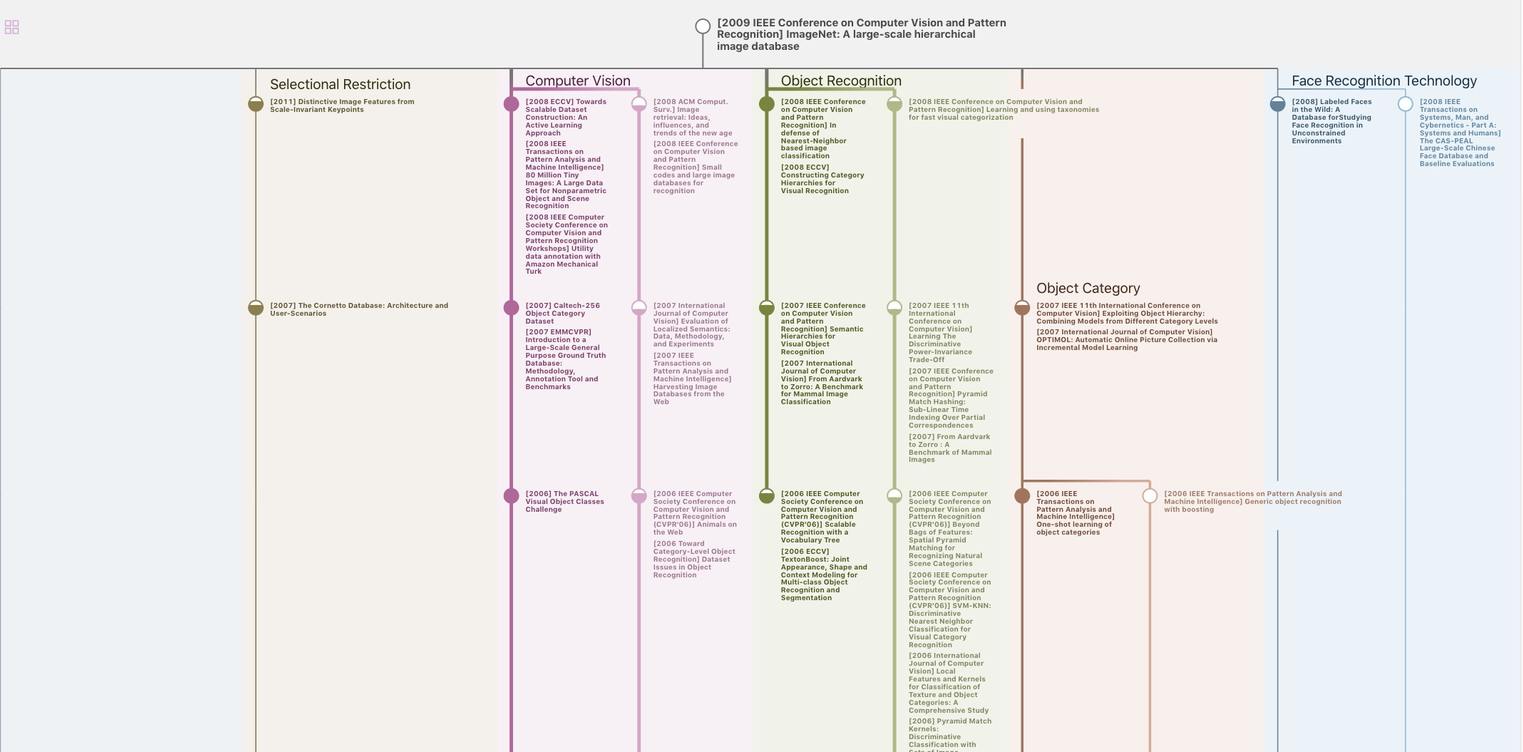
生成溯源树,研究论文发展脉络
Chat Paper
正在生成论文摘要