Browsing weighted interactome models using GeneDive
BCB(2021)
Abstract
ABSTRACTInteractome models are key to understanding metabolic functions and mechanisms of medical interventions. There are two problems that need to be solved to deliver and use high-quality interactome models: (1) network curation at scale; and (2) bringing large-scale interactomes to human-scale understanding. To address these problems we present GeneDive, a computational systems biology interactome browser that makes large gene, disease, and drug interactions networks easily accessible and usable. GeneDive can be accessed at: https://www.genedive.net/ Expert curation of interaction networks is considered the gold standard for quality, but is limited in scope due to prohibitive costs. Automated molecular interaction network labeling is relatively inexpensive and can perform at scale, but lacks the quality of manual curation. Hybrid approaches that leverage the scalability of automated methods and the quality of expert curation processes have the potential to substantially expand our understanding of interactome models. GeneDive web-application is designed to facilitate such hybrid curation efforts. Machine learning (ML) based approaches have been shown to assign high confidence scores to interactions with strong evidence in scientific literature, while assigning lower scores to interactions with less evidence. Subnetworks containing only high confidence scores have been shown to match strongly with expert curated networks. This suggests that interactions with mid-ranged scores may be yet undiscovered or not as well-researched. Edges in interaction networks can be weighted by these confidence scores to represent interactions found in literature and the abundance of relevant support from said literature. A weighted interactome browser, like GeneDive, can recall such understudied subnetworks and their surrounding context to prioritize curation efforts. Once these subnetworks are curated, the following process with a positive feedback loop can be applied: (1) on the ML algorithm (which can be retrained with the latest curations, improving accuracy); and (2) on the curation effort to identify the next "low-hanging fruit" discoveries within the browser. An iterative application of this process can rapidly grow the amount of high-quality interactome models available to the biomedical community. In addition to improving the underlying data quality and scale for weighted interactome models, GeneDive also helps biomedical researchers use said models for biomedical discoveries. In previously published work, we outlined eight use cases where GeneDive could be used to help clinicians and researchers gain a better understanding through search and visualization of the interactome model. GeneDive provides the following key features to facilitate discoveries in weighted interactome browsing: multiple search modalities, Cytoscape network visualization, a tabular view of interactions with supporting evidence and confidence scores, highlighting, free-text filtering for all fields, and filtering by confidence scores. Latest improvements to the GeneDive web-application include the ability to import user-provided data using an extensible semi-structured schema (previously, only fixed-schema user-provided data could be imported), and the ability to add the extensible fields to the tabular view via a plugin architecture. The ability to consolidate multiple datasets is supported without compromising data ownership and privacy. GeneDive promotes longitudinal and exploratory investigations that can be reproduced to enable branched hypothesis testing.
MoreTranslated text
Key words
interactome models
AI Read Science
Must-Reading Tree
Example
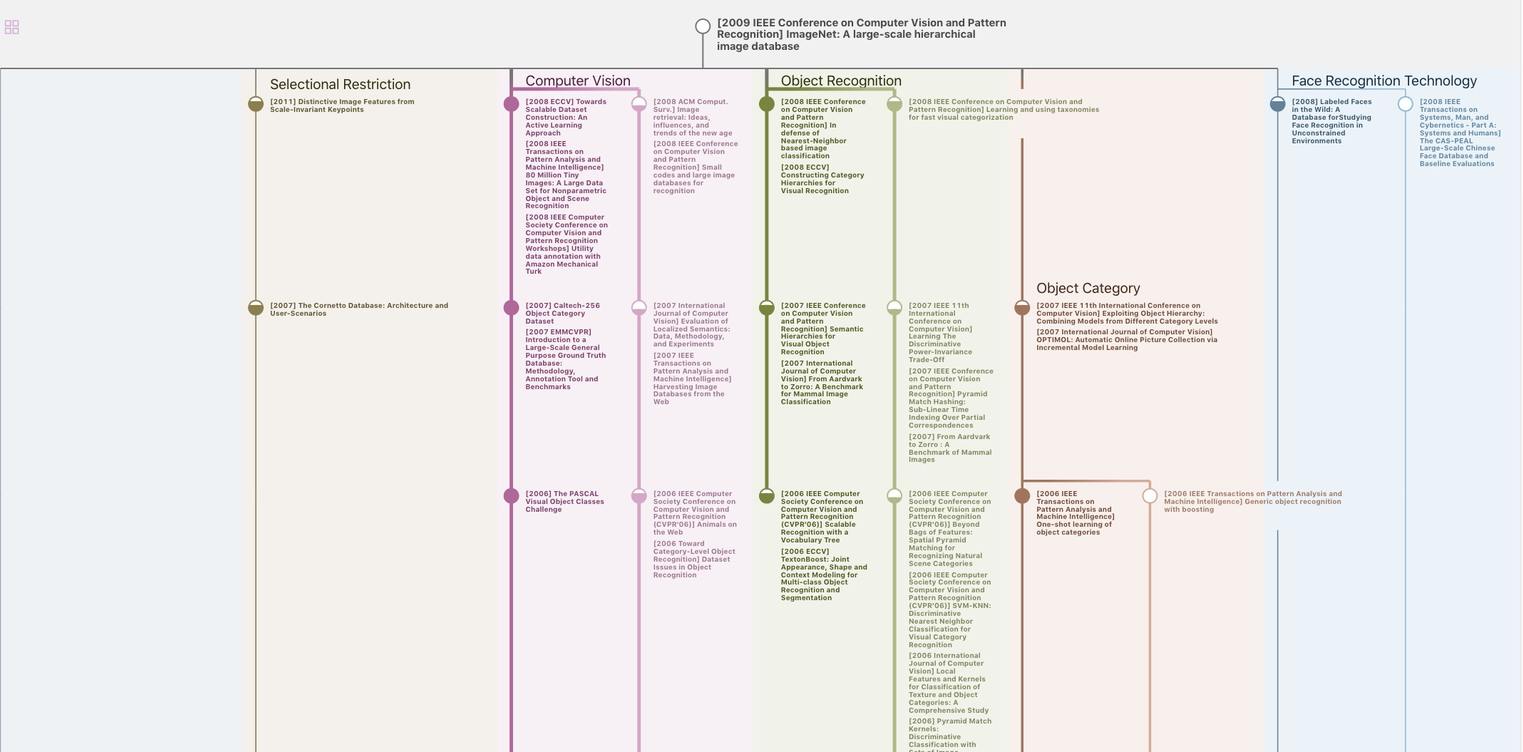
Generate MRT to find the research sequence of this paper
Chat Paper
Summary is being generated by the instructions you defined