Enhancing Neural Machine Translation With Dual-Side Multimodal Awareness
IEEE TRANSACTIONS ON MULTIMEDIA(2022)
摘要
Multimodal machine translation (MMT) aims to translate a sentence in the source language into the target language with the context of an associated image. According to where the visual information is employed, previous approaches can be categorized into two types: directly injecting the visual information at the input side or exploiting it as a visual constraint at the objective side. In this work, we propose an IO-MMT model which exploits the visual assistance in dual sides to fully exploit the visual information for multimodal machine translation. It contains a relation-aware multimodal transformer to simultaneously exploit the objects and their spatial relationships in the image with a graph at the input-side and a novel visual assistance structure to further improve visual consistency of the translation at the objective-side. Experimental results under both normal setting and input degradation settings on the Multi30k benchmark dataset show that combining the visual assistance in dual sides consistently outperforms single-side MMT models and achieves the state-of-the-art results on EN-DE and EN-FR translation tasks. We will release the codes and models at
https://github.com/syuqings/MMT
.
更多查看译文
关键词
Visualization, Machine translation, Task analysis, Training, Decoding, Semantics, Degradation, Multimodal machine translation, graph, relationship, multimodal rewards, reinforcement learning
AI 理解论文
溯源树
样例
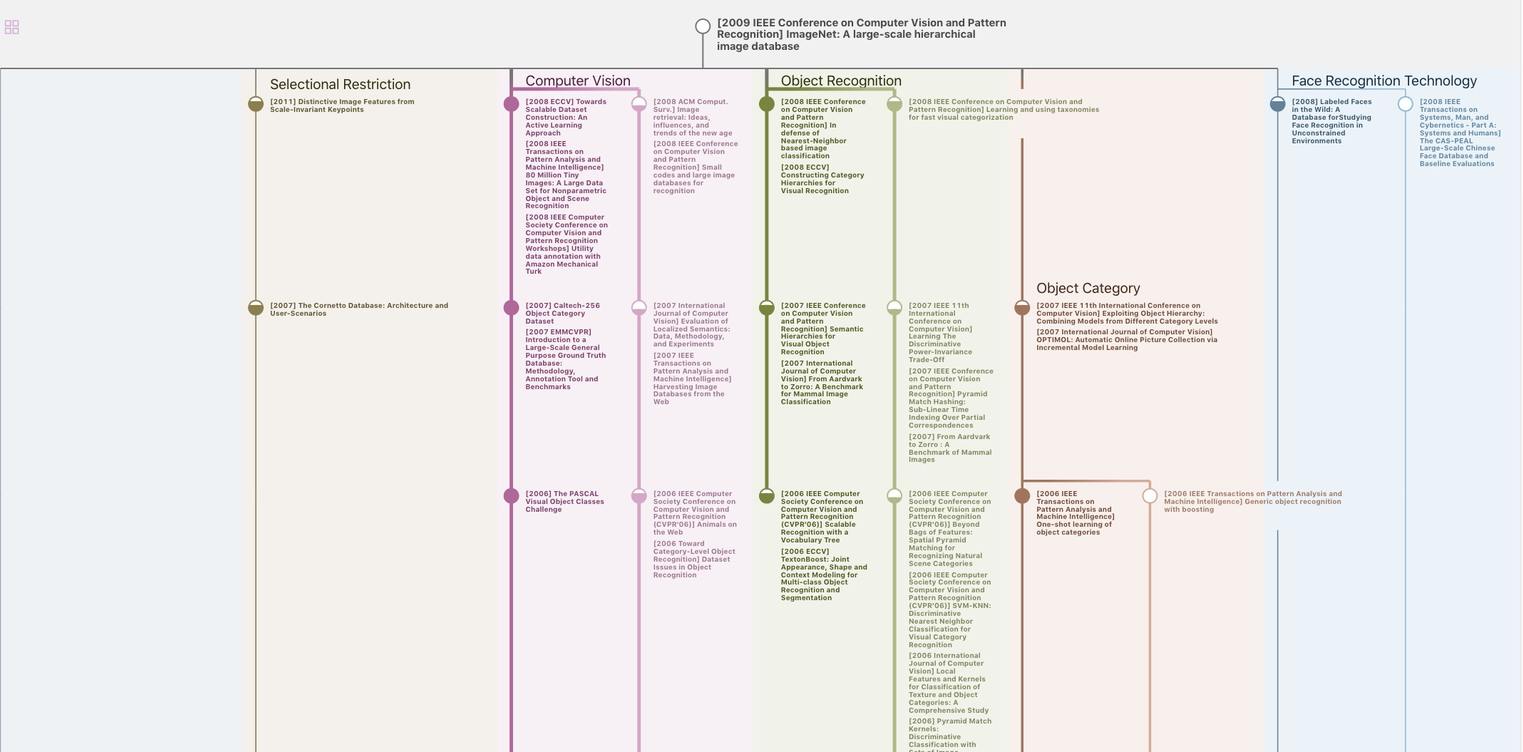
生成溯源树,研究论文发展脉络
Chat Paper
正在生成论文摘要