Cloud Network Trace Anonymization
user-60ab1d9b4c775e04970067d6(2021)
摘要
Cloud traffic is estimated to represent 95% of the global traffic [1]. Grand challenges in designing the vast ecosystem of cloud systems and infrastructures, ranging from topology design to programmability and congestion management, have been the focus of numerous research projects in recent years due to their intellectual appeal and the potential to make a significant impact on large-scale deployed systems. Studying the characteristics of datacenter traffic is crucial for understanding and extending today’s cloud systems, designing new ones, and reproducing existing research. To address this need, a few datasets and samples of datacenter traces have been released in recent years [5, 4, 2]. While a powerful tool for many projects, these datasets share some major limitations that act as obstacles for conducting foundational research on datacenters: (a) They exclude some of the key metrics for evaluating today’s cloud systems and applications such as recent failure and attack statistics, the evolution of such statistics over time, and highresolution timing characteristics of packet traces (e.g., inter-packet gaps). (b) They are created by coarse-grained packet sampling techniques (e.g., with a 1:30,000 packet sampling rate [5]) and suffer from sampling biases such as its impact on observed flow sizes. (c) With the exception of a few (and relatively old) public packet traces, e.g., for a few university datacenters [2] (showing markedly different traffic characteristics compared to the recent datacenter measurements), they are from a few datacenters (mostly one social network, in recent years [5, 4]) with one particular set of applications and workloads that are not necessarily representative of the entire cloud ecosystem. (d) Even for reporting high-level and aggregate statistics, various key metrics are normalized using ad hoc techniques (see, for instance, Swift’s loss, latency, and throughput statistics [3]). The effectiveness and necessity of these techniques for preserving confidentiality is less known. The technical challenges for resolving some of these limitations via accurate measurements have been the focus of many recent telemetry research and deployed measurement projects such as data collection frameworks that enable polling switch statistics at a microsecond granularity [5] and high-resolution latency measurements at the edge of datacenter [3]. Consequently, today’s datacenter fabrics have powerful capabilities for measuring and storing high-resolution traces. Still, few data sets collected from these measurements are available to the community. We believe a major remaining barrier is the less studied concerns surrounding the confidentiality risks of data sharing. Addressing these concerns requires a systemic investigation of anonimization metrics and techniques to preserve confidentiality while preserving the utility for systems research. The current scarcity of publicly available datasets with sufficiently rich sets of data types and attributes implies that a large space of intellectually stimulating problems with potentially high impact is not accessible to the broader research community and is restricted to a handful of groups with internal access. It also implies that the research published by these groups cannot be independently reproduced, verified, and extended by the community. To work around this limitation, a plethora of recent datacenter research projects have focused on important, but narrow, niches such as traffic engineering and telemetry—for which the (partially synthetic) available datasets are deemed adequate. Alas, this does not reflect the diversity of users and providers, the plurality of systems and designs, and the scale and far-reaching impact of cloud computing. Plus, even for these subsets of problems, these projects tend to be optimized for a few—and conceivably non-representative—types of datacenters with public traces. To have a better understanding of the broader cloud landscape, access to larger and more diverse datasets is key. We believe a promising approach to facilitate this is to understand and reduce the perceived risks of sharing measurement data for network providers via systematically (a) studying the concerns of network providers and their users regarding sharing the data, (b) identifying the measurements and data sets with the potential to usher in innovative ideas to tackle critical and big challenges in cloud computing (such as data-driven attack-resilient and fault-tolerant designs) that can be of value to providers, (c) developing anonymization metrics to evaluate the level of anonymity and the richness of the data sets for various types of systems research, (d) developing efficient anonymization techniques to preserve the utility of the measurements for systems research while preventing information leakage, and designing techniques to minimize the risk of de-anonymization via cross-referencing with other available sources and the concerns of de-anonymization over time. This can be a key enabler for motivating a more diverse set of cloud providers to share their measurements who may have little incentive and/or internal resources to develop trustworthy anonymization techniques.
更多查看译文
AI 理解论文
溯源树
样例
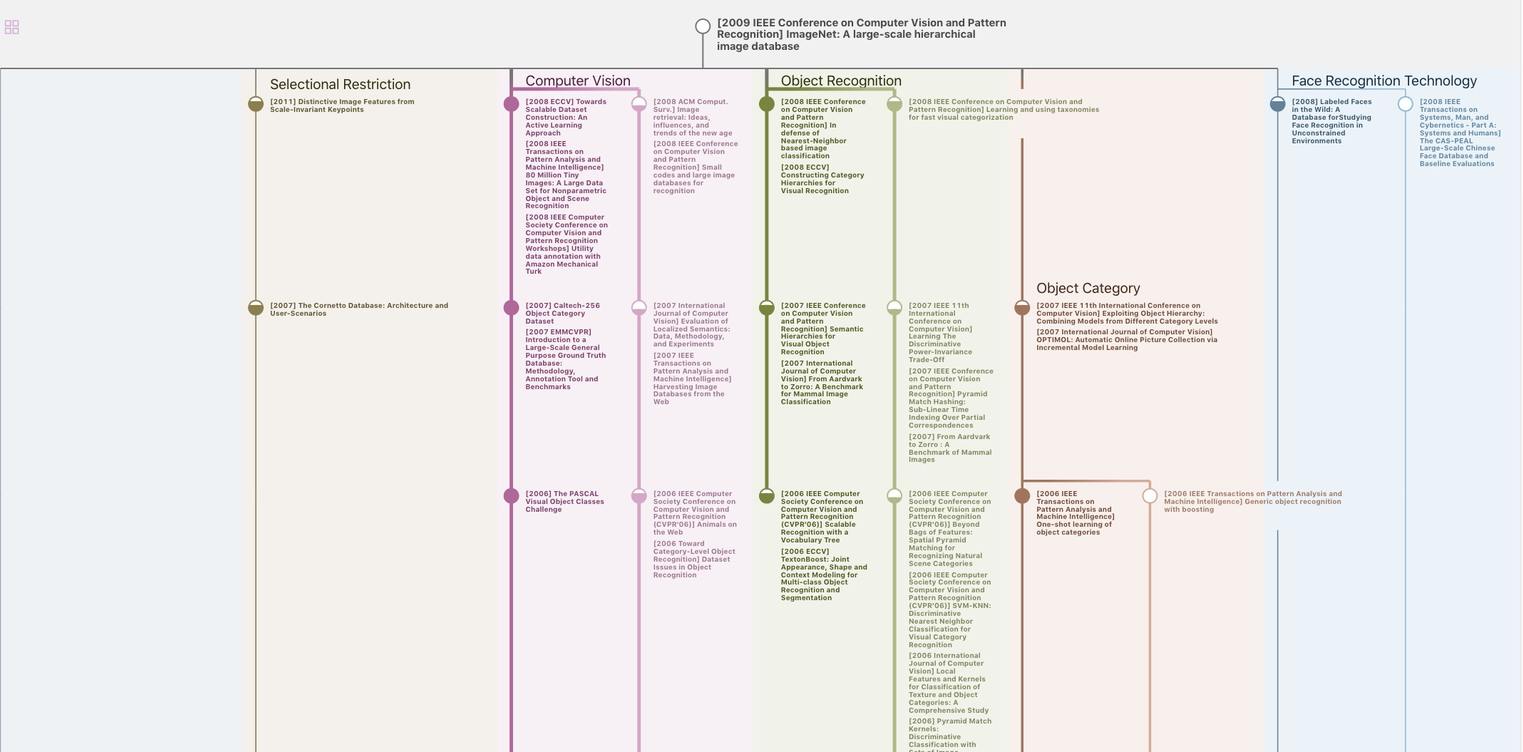
生成溯源树,研究论文发展脉络
Chat Paper
正在生成论文摘要