An Encoder-Decoder Based Audio Captioning System with Transfer and Reinforcement Learning.
DCASE(2021)
摘要
Automated audio captioning aims to use natural language to describe the content of audio data. This paper presents an audio captioning system with an encoder-decoder architecture, where the decoder predicts words based on audio features extracted by the encoder. To improve the proposed system, transfer learning from either an upstream audio-related task or a large in-domain dataset is introduced to mitigate the problem induced by data scarcity. Besides, evaluation metrics are incorporated into the optimization of the model with reinforcement learning, which helps address the problem of ``exposure bias'' induced by ``teacher forcing'' training strategy and the mismatch between the evaluation metrics and the loss function. The resulting system was ranked 3rd in DCASE 2021 Task 6. Ablation studies are carried out to investigate how much each element in the proposed system can contribute to final performance. The results show that the proposed techniques significantly improve the scores of the evaluation metrics, however, reinforcement learning may impact adversely on the quality of the generated captions.
更多查看译文
关键词
reinforcement learning,encoder-decoder
AI 理解论文
溯源树
样例
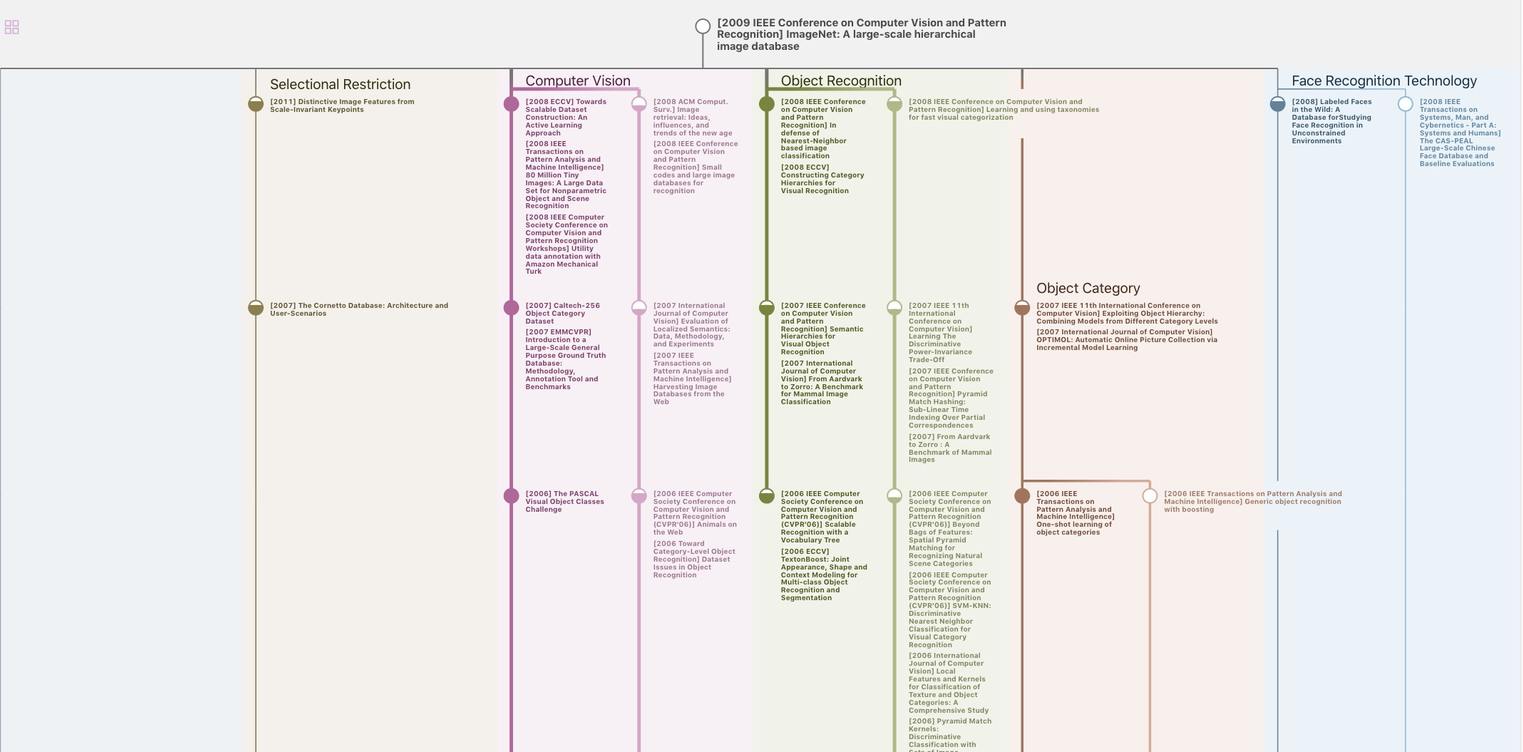
生成溯源树,研究论文发展脉络
Chat Paper
正在生成论文摘要