Missingness Augmentation: A General Approach for Improving Generative Imputation Models
arxiv(2023)
摘要
Missing data imputation is a fundamental problem in data analysis, and many studies have been conducted to improve its performance by exploring model structures and learning procedures. However, data augmentation, as a simple yet effective method, has not received enough attention in this area. In this paper, we propose a novel data augmentation method called Missingness Augmentation (MisA) for generative imputation models. Our approach dynamically produces incomplete samples at each epoch by utilizing the generator's output, constraining the augmented samples using a simple reconstruction loss, and combining this loss with the original loss to form the final optimization objective. As a general augmentation technique, MisA can be easily integrated into generative imputation frameworks, providing a simple yet effective way to enhance their performance. Experimental results demonstrate that MisA significantly improves the performance of many recently proposed generative imputation models on a variety of tabular and image datasets. The code is available at \url{https://github.com/WYu-Feng/Missingness-Augmentation}.
更多查看译文
关键词
imputation models
AI 理解论文
溯源树
样例
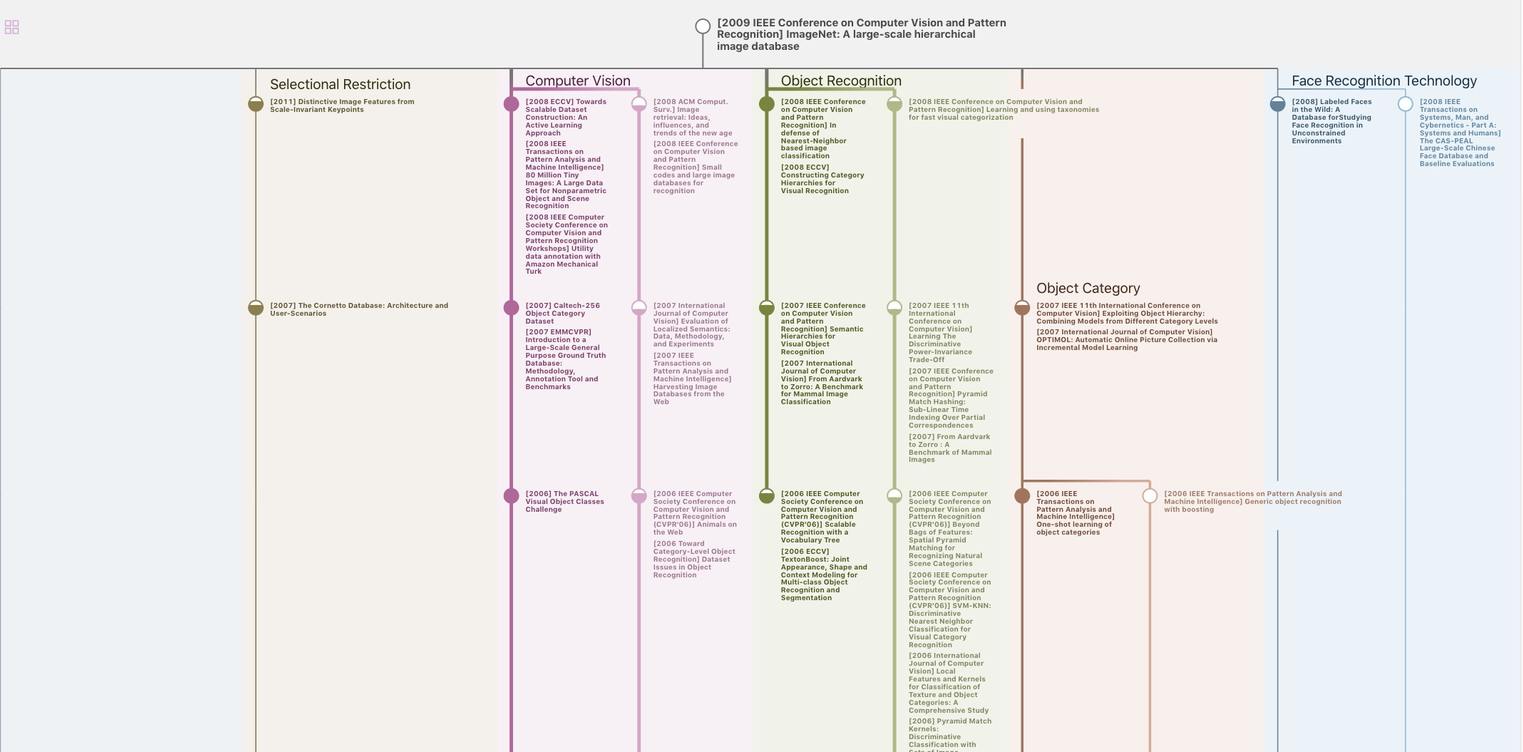
生成溯源树,研究论文发展脉络
Chat Paper
正在生成论文摘要