Evaluation of Audio-Visual Alignments in Visually Grounded Speech Models.
Interspeech(2021)
摘要
Systems that can find correspondences between multiple modalities, such as between speech and images, have great potential to solve different recognition and data analysis tasks in an unsupervised manner. This work studies multimodal learning in the context of visually grounded speech (VGS) models, and focuses on their recently demonstrated capability to extract spatiotemporal alignments between spoken words and the corresponding visual objects without ever been explicitly trained for object localization or word recognition. As the main contributions, we formalize the alignment problem in terms of an audiovisual alignment tensor that is based on earlier VGS work, introduce systematic metrics for evaluating model performance in aligning visual objects and spoken words, and propose a new VGS model variant for the alignment task utilizing cross-modal attention layer. We test our model and a previously proposed model in the alignment task using SPEECH-COCO captions coupled with MSCOCO images. We compare the alignment performance using our proposed evaluation metrics to the semantic retrieval task commonly used to evaluate VGS models. We show that cross-modal attention layer not only helps the model to achieve higher semantic cross-modal retrieval performance, but also leads to substantial improvements in the alignment performance between image object and spoken words.
更多查看译文
关键词
cross-modal learning,audio-visual alignment,visual object localization,word segmentation
AI 理解论文
溯源树
样例
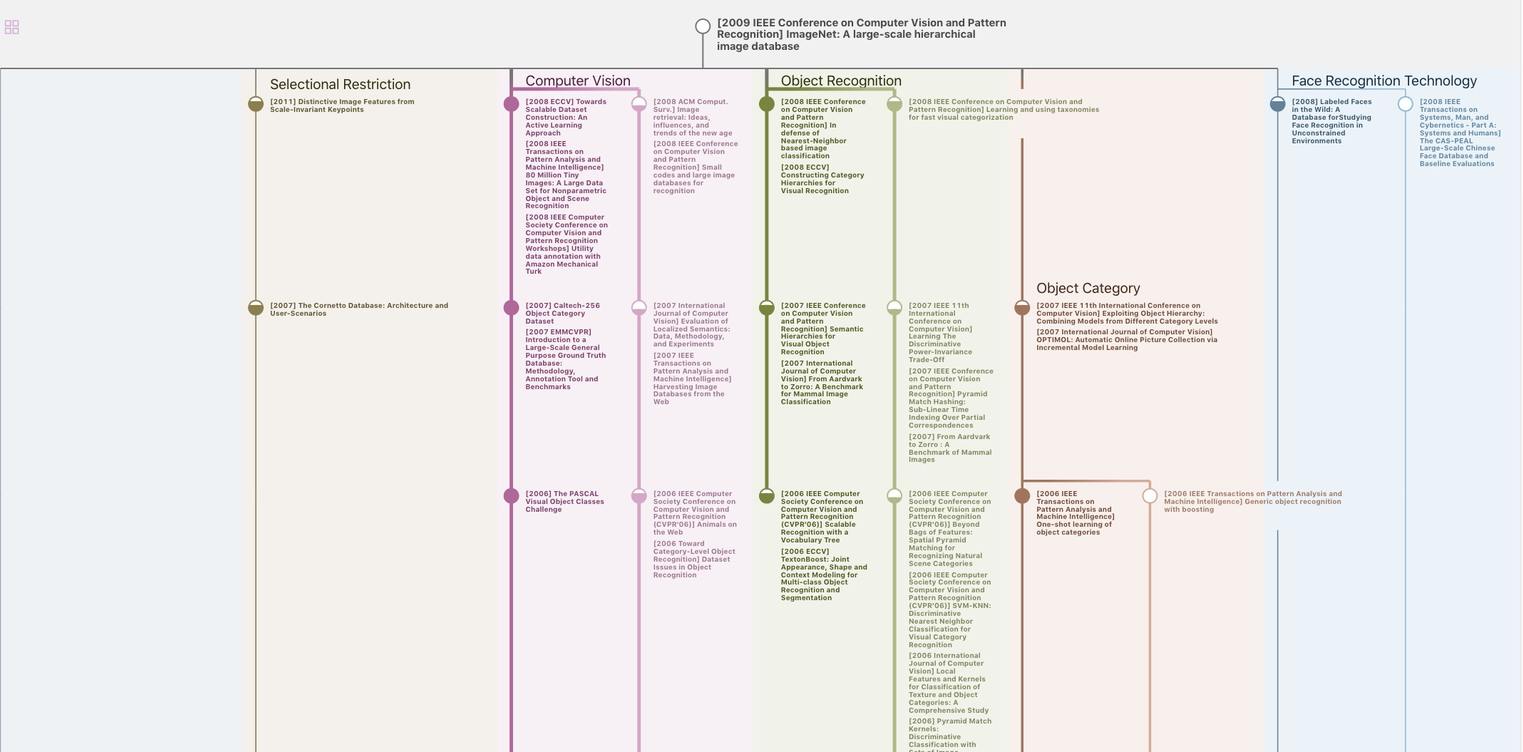
生成溯源树,研究论文发展脉络
Chat Paper
正在生成论文摘要