A Machine Learning Approach For Prioritizing Groundwater Testing For Per-And Polyfluoroalkyl Substances (Pfas)
JOURNAL OF ENVIRONMENTAL MANAGEMENT(2021)
摘要
Regulatory agencies are beginning to recognize and regulate per-and polyfluoroalkyl substances (PFAS) as concerning environmental contaminants. In groundwater management, testing and mitigation strategies are desirable, but can be time and cost-intensive processes. As a result, only a fraction of all groundwater wells has been tested for PFAS levels, resulting in potentially extended drinking water exposure to PFAS in the meantime. In this study, we build a series of machine learning models (including linear and random forest regressors) to predict PFAS based on a groundwater dataset from California. These models are used to compare the relative predictive ability of co-contaminant fingerprints, hydrological properties, soil parameters, proximity of airports/ military bases, and geospatial data. Additionally, a random forest machine learning model that combines all data types can quantitatively predict the maximum PFAS compound concentration in a well with a Spearman correlation of 0.64 and can discern wells containing concerningly high concentrations of PFAS with an accuracy of 91 % (AUC of 0.90). This approach may have widespread utility for other hazardous anthropogenic compounds in groundwater. Future investigations should evaluate the practicability of using machine learning to prospectively prioritize contaminant testing in groundwater wells.
更多查看译文
关键词
Machine learning, Groundwater, Chemical fingerprints, PFAS, Open data
AI 理解论文
溯源树
样例
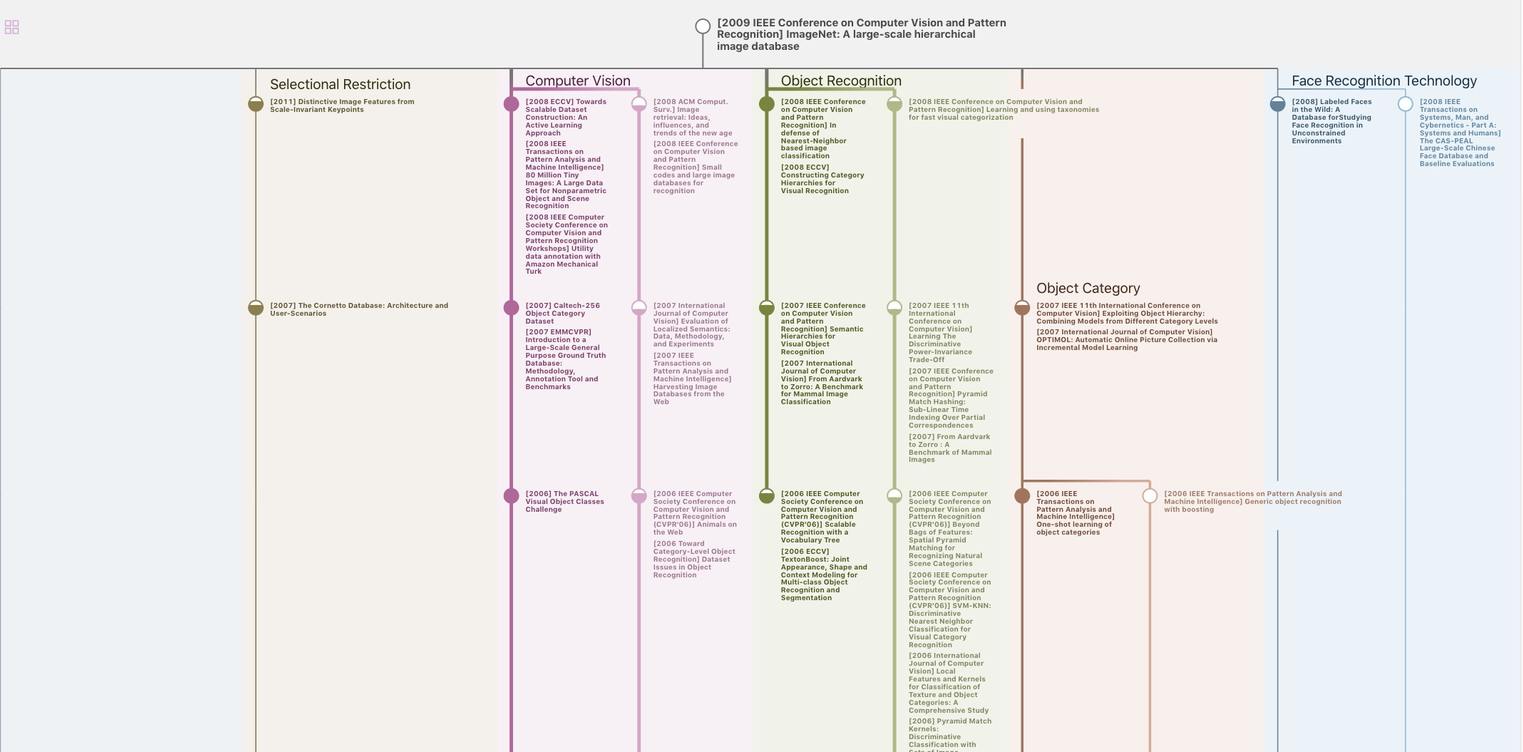
生成溯源树,研究论文发展脉络
Chat Paper
正在生成论文摘要