Temporal Convolutional Network Based Regression Approach for Estimation of Remaining Useful Life
2021 IEEE International Conference on Prognostics and Health Management (ICPHM)(2021)
摘要
Remaining Useful Life (RUL) is an essential factor in the Prognostics and Health Management (PHM) field. A reliable and accurate RUL estimation of the condition monitoring data could maximize system performance and reduce maintenance costs. Recently, with a surge of interest in deep learning (DL) and the rise of computational power, many state-of-the-art neural networks have been introduced in the PHM field. However, the previously proposed networks have drawbacks in handling sequential tasks. For example, the widely-used Recurrent Neural Network (RNN) and Long Short-term Neural Network (LSTM) have long-term dependency problems and gradient vanishing problems. In this paper, we adopt the Temporal Convolutional Network (TCN), which excels in sequential data processing and avoid potential problems shared by the aforementioned models. We have leveraged TCN on the C-MAPSS Dataset from NASA to examine its performance in RUL estimation. Our experiments result shows that TCN outperforms all the previous proposed neural networks for RUL estimation, which indicates the potential of TCN applications in the PHM field.
更多查看译文
关键词
Alternative to RNN,Prognostics Health Management,Remaining Useful Life,Temporal Convolutional Network.
AI 理解论文
溯源树
样例
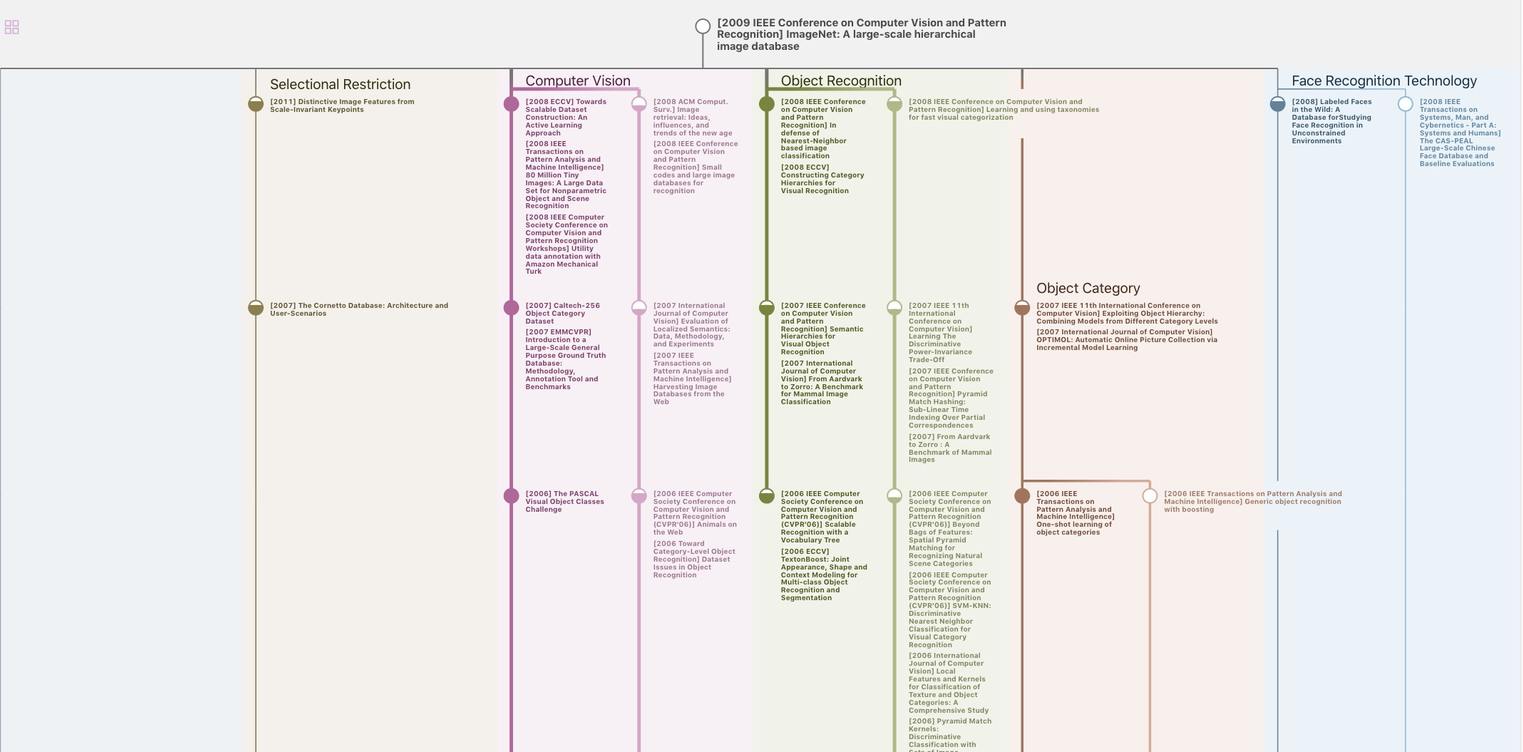
生成溯源树,研究论文发展脉络
Chat Paper
正在生成论文摘要