Block-based Learned Image Coding with Convolutional Autoencoder and Intra-Prediction Aided Entropy Coding
2021 Picture Coding Symposium (PCS)(2021)
摘要
Recent works on learned image coding using autoencoder models have achieved promising results in rate-distortion performance. Typically, an autoencoder is used to transform an image into a latent tensor, which is then quantized and entropy coded. Based on a work by Ballé et al., we adapted the autoencoder with a hyperprior model to code images in a block-based approach. When the autoencoder model is directly applied to code small image blocks, spatial redundancy in the larger image cannot be fully utilized, resulting in a decrease in ratedistortion performance. We propose a method to utilize border information in the entropy coding of latent and hyper-latent tensors, which has achieved promising results. We show that using intra-prediction to help entropy coding is more effective than applying a convolutional autoencoder with hyper priors to intra-prediction residual blocks.
更多查看译文
关键词
Block-based coding,deep learning,intraprediction,learned image compression
AI 理解论文
溯源树
样例
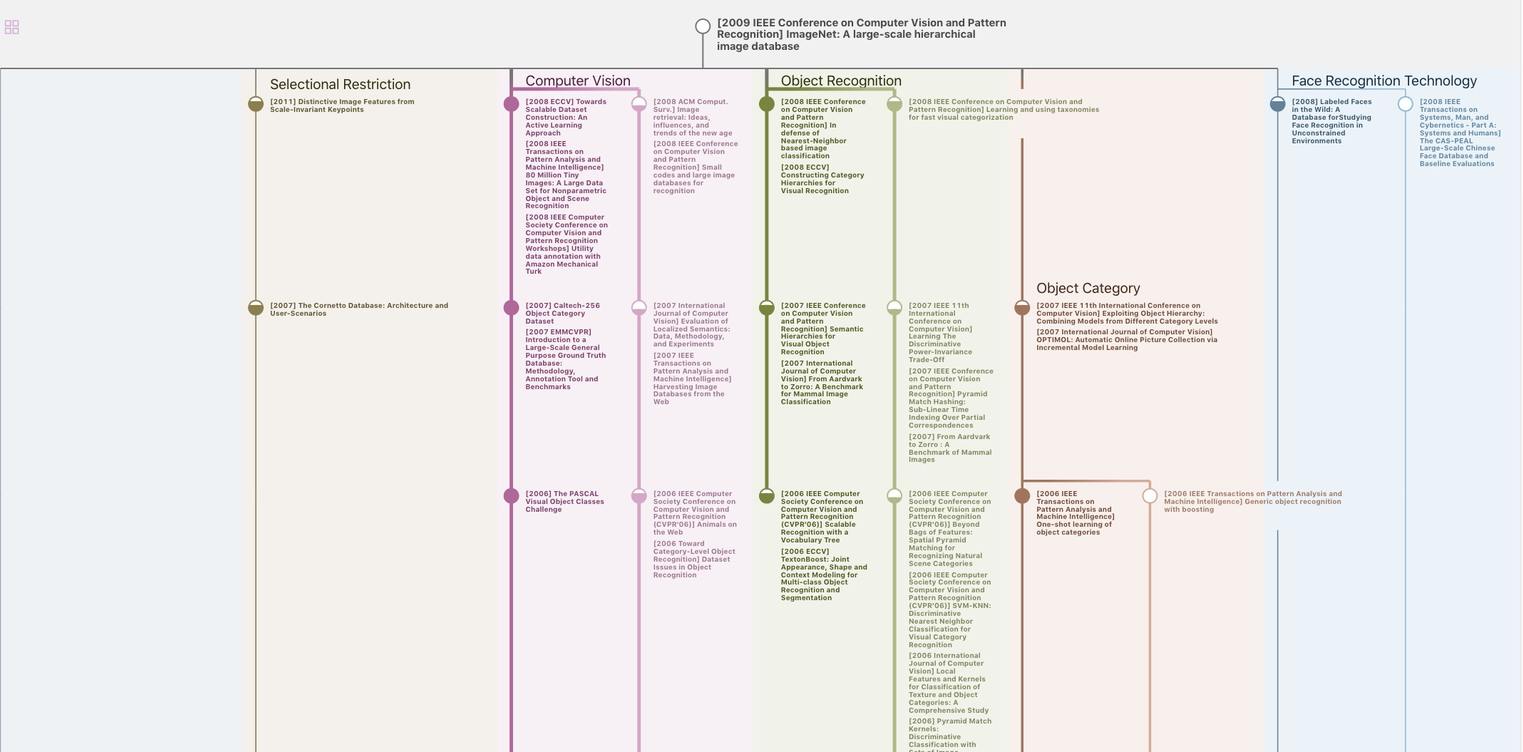
生成溯源树,研究论文发展脉络
Chat Paper
正在生成论文摘要