Explainable AI and Adoption of Financial Algorithmic Advisors: An Experimental Study
AIES '21: PROCEEDINGS OF THE 2021 AAAI/ACM CONFERENCE ON AI, ETHICS, AND SOCIETY(2021)
摘要
We study whether receiving advice from either a human or algorithmic advisor, accompanied by five types of Local and Global explanation labelings, has an effect on the readiness to adopt, willingness to pay, and trust in a financial AI consultant. We compare the differences over time and in various key situations using a unique experimental framework where participants play a web-based game with real monetary consequences. We observed that accuracy-based explanations of the model in initial phases leads to higher adoption rates. When the performance of the model is immaculate, there is less importance associated with the kind of explanation for adoption. Using more elaborate feature-based or accuracy-based explanations helps substantially in reducing the adoption drop upon model failure. Furthermore, using an autopilot increases adoption significantly. Participants assigned to the AI-labeled advice with explanations were willing to pay more for the advice than the AI-labeled advice with "No-explanation" alternative. These results add to the literature on the importance of XAI for algorithmic adoption and trust.
更多查看译文
关键词
HCI, Explainable AI, Financial Advice, Trust, Algorithm Adoption, Experiment
AI 理解论文
溯源树
样例
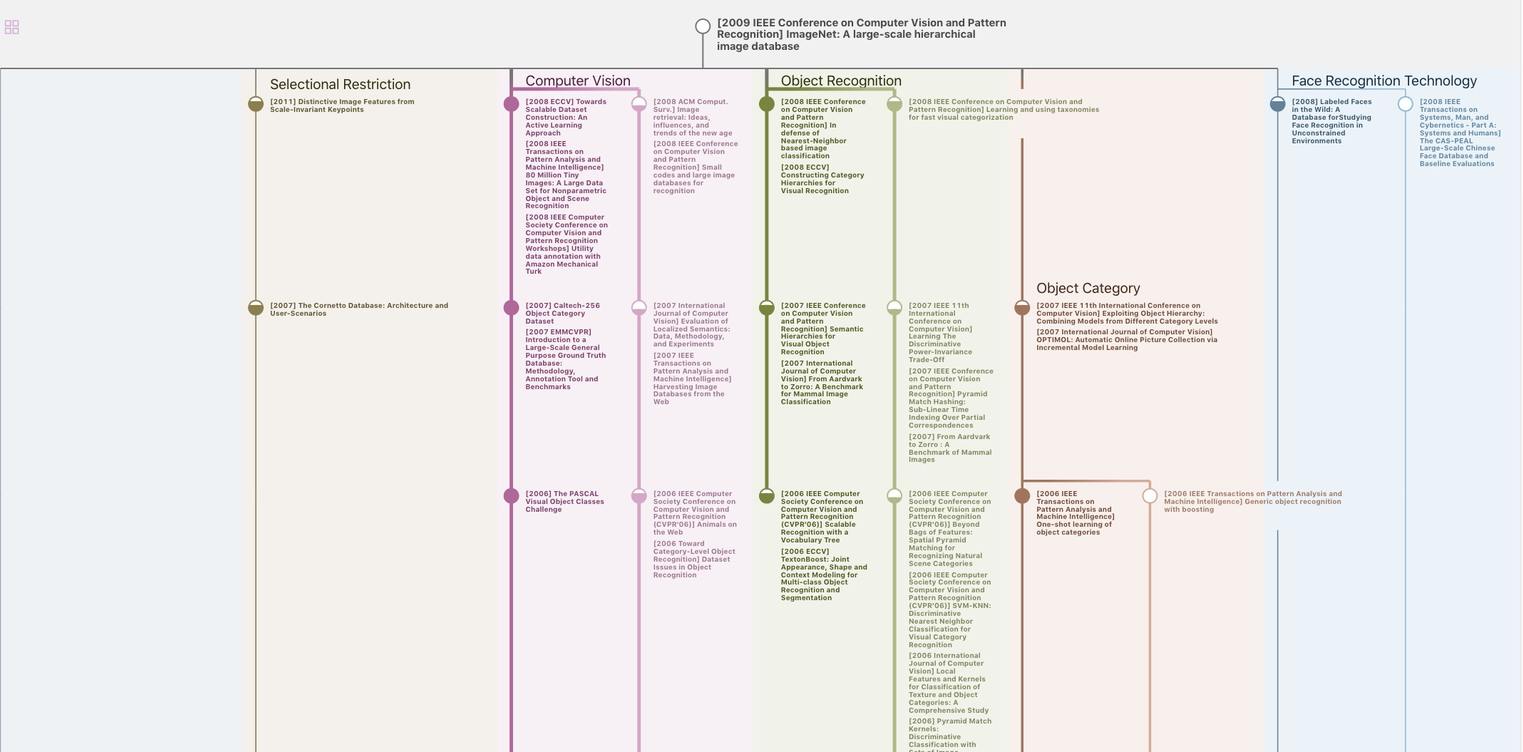
生成溯源树,研究论文发展脉络
Chat Paper
正在生成论文摘要