Analog In-memory Computing in FeFET-based 1T1R Array for Edge AI Applications
2021 Symposium on VLSI Technology(2021)
摘要
Deep neural network (DNN) inference for edge AI requires low-power operation, which can be achieved by implementing massively parallel matrix-vector multiplications (MVM) in the analog domain on a highly resistive memory array. We propose a 1T1R compute cell (1T1R-cell) using a ferroelectric hafnium oxide-based FET (FeFET) and TiN/SiO2 tunneling junction of MΩ resistor (MOR) for analog in-memory computing (AiMC). The MOR exhibited a tunneling current behavior and MΩ resistance. A 1T1R-cell array-level evaluation was also performed. A random access for writing with low write disturbance scheme was confirmed from the summation-DC-current output, and binaries were successfully classified into "T" and "L." Based on the experimental results of our proposed 1T1R-cell, we obtained a state-of-the-art energy efficiency of 13700 TOPS/W including the periphery. Furthermore, we confirmed that a high inference accuracy can be obtained with our low-resistance-variability 1T1R-cell with a properly trained model.
更多查看译文
关键词
1t1r array,edge ai applications,in-memory,fefet-based
AI 理解论文
溯源树
样例
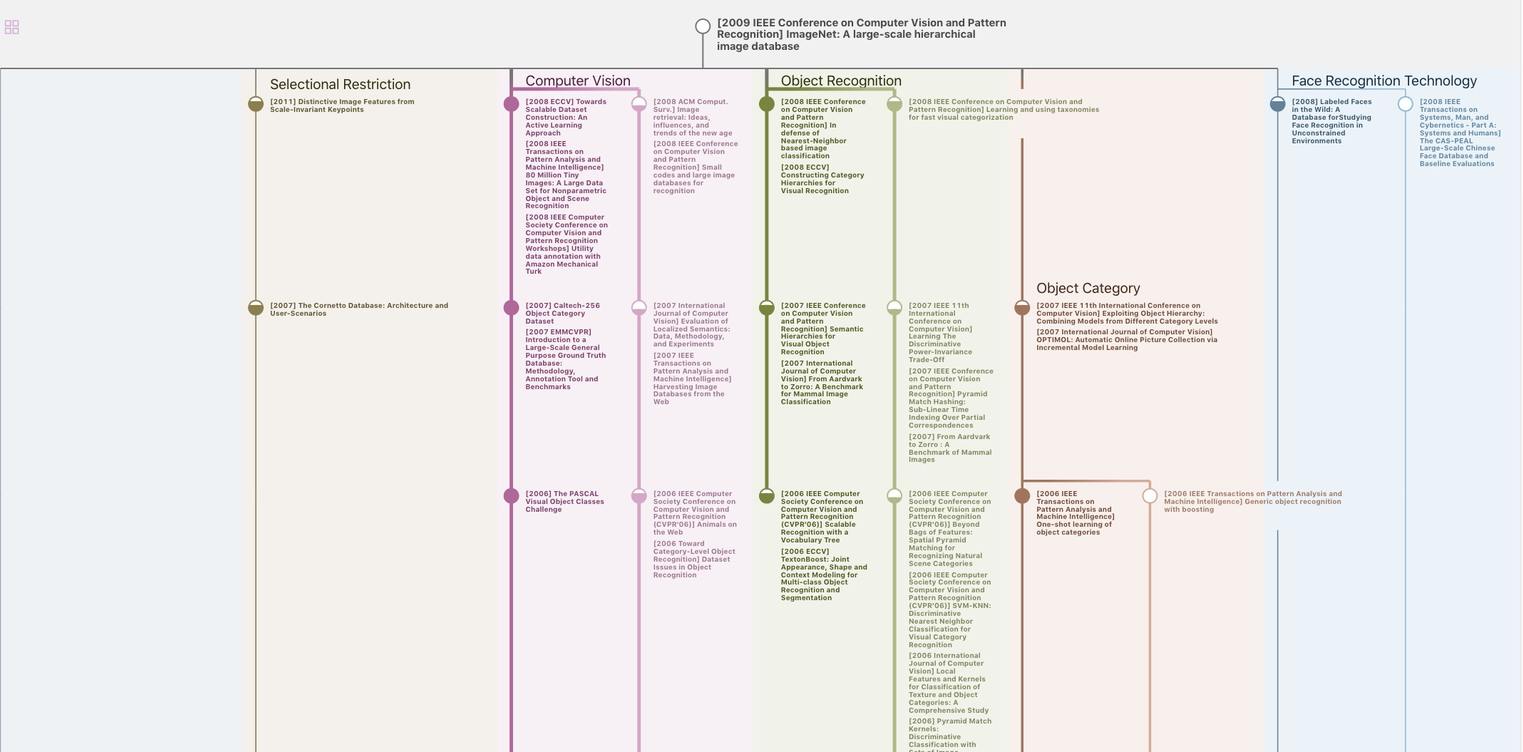
生成溯源树,研究论文发展脉络
Chat Paper
正在生成论文摘要