On the Robustness of Domain Adaption to Adversarial Attacks
CoRR(2021)
Abstract
State-of-the-art deep neural networks (DNNs) have been proved to have excellent performance on unsupervised domain adaption (UDA). However, recent work shows that DNNs perform poorly when being attacked by adversarial samples, where these attacks are implemented by simply adding small disturbances to the original images. Although plenty of work has focused on this, as far as we know, there is no systematic research on the robustness of unsupervised domain adaption model. Hence, we discuss the robustness of unsupervised domain adaption against adversarial attacking for the first time. We benchmark various settings of adversarial attack and defense in domain adaption, and propose a cross domain attack method based on pseudo label. Most importantly, we analyze the impact of different datasets, models, attack methods and defense methods. Directly, our work proves the limited robustness of unsupervised domain adaptation model, and we hope our work may facilitate the community to pay more attention to improve the robustness of the model against attacking.
MoreTranslated text
Key words
adversarial attacks,robustness,domain adaption
AI Read Science
Must-Reading Tree
Example
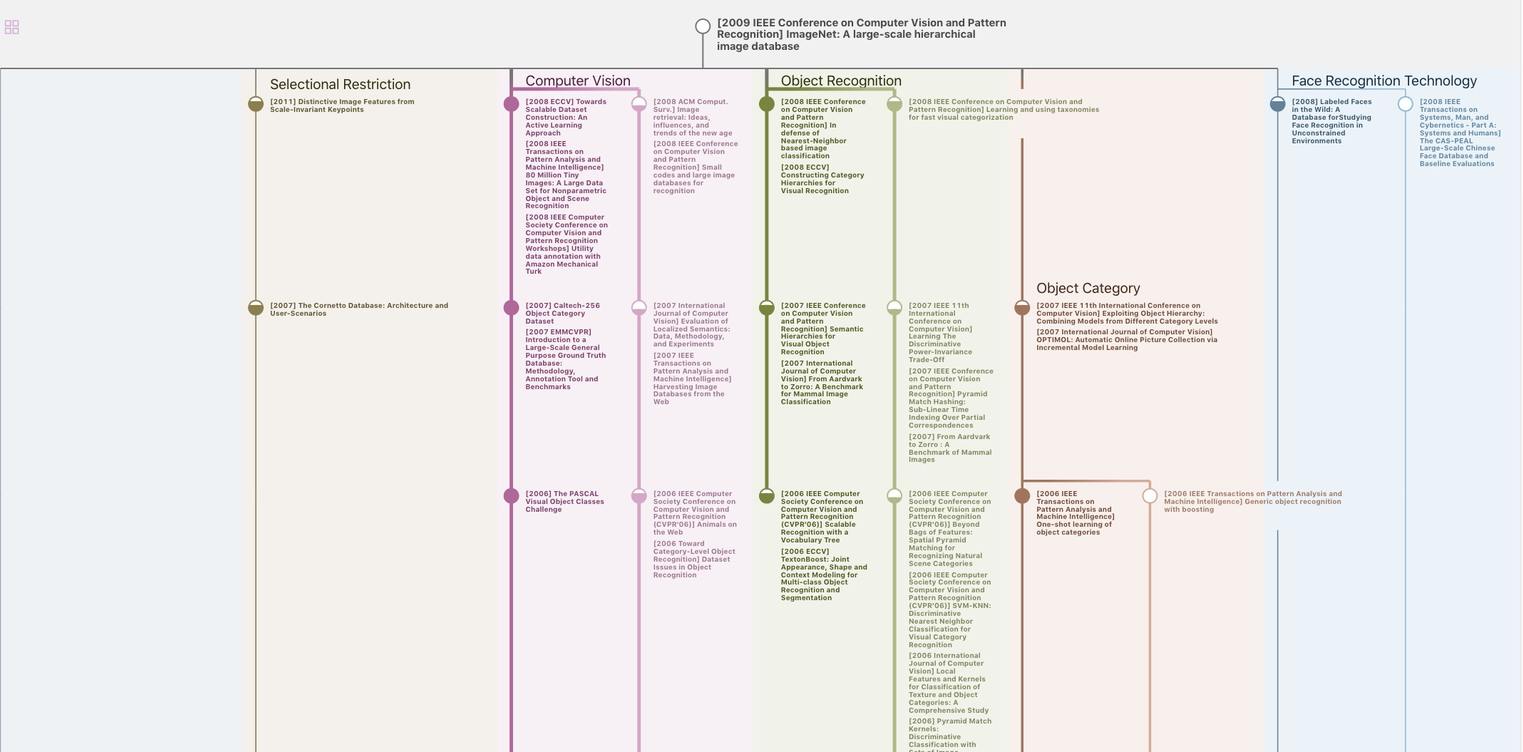
Generate MRT to find the research sequence of this paper
Chat Paper
Summary is being generated by the instructions you defined