A systematic review of automatic text summarization for biomedical literature and EHRs
JOURNAL OF THE AMERICAN MEDICAL INFORMATICS ASSOCIATION(2021)
摘要
Objective: Biomedical text summarization helps biomedical information seekers avoid information overload by reducing the length of a document while preserving the contents' essence. Our systematic review investigates the most recent biomedical text summarization researches on biomedical literature and electronic health records by analyzing their techniques, areas of application, and evaluation methods. We identify gaps and propose potential directions for future research. Materials and Methods: This review followed the PRISMA methodology and replicated the approaches adopted by the previous systematic review published on the same topic. We searched 4 databases (PubMed, ACM Digital Library, Scopus, and Web of Science) from January 1, 2013 to April 8, 2021. Two reviewers independently screened title, abstract, and full-text for all retrieved articles. The conflicts were resolved by the third reviewer. The data extraction of the included articles was in 5 dimensions: input, purpose, output, method, and evaluation. Results: Fifty-eight out of 7235 retrieved articles met the inclusion criteria. Thirty-nine systems used single-document biomedical research literature as their input, 17 systems were explicitly designed for clinical support, 47 systems generated extractive summaries, and 53 systems adopted hybrid methods combining computational linguistics, machine learning, and statistical approaches. As for the assessment, 51 studies conducted an intrinsic evaluation using predefined metrics. Discussion and Conclusion: This study found that current biomedical text summarization systems have achieved good performance using hybrid methods. Studies on electronic health records summarization have been increasing compared to a previous survey. However, the majority of the works still focus on summarizing literature.
更多查看译文
关键词
automatic text summarization, machine learning, computational linguistics, biomedical and health sciences literature, electronic health records
AI 理解论文
溯源树
样例
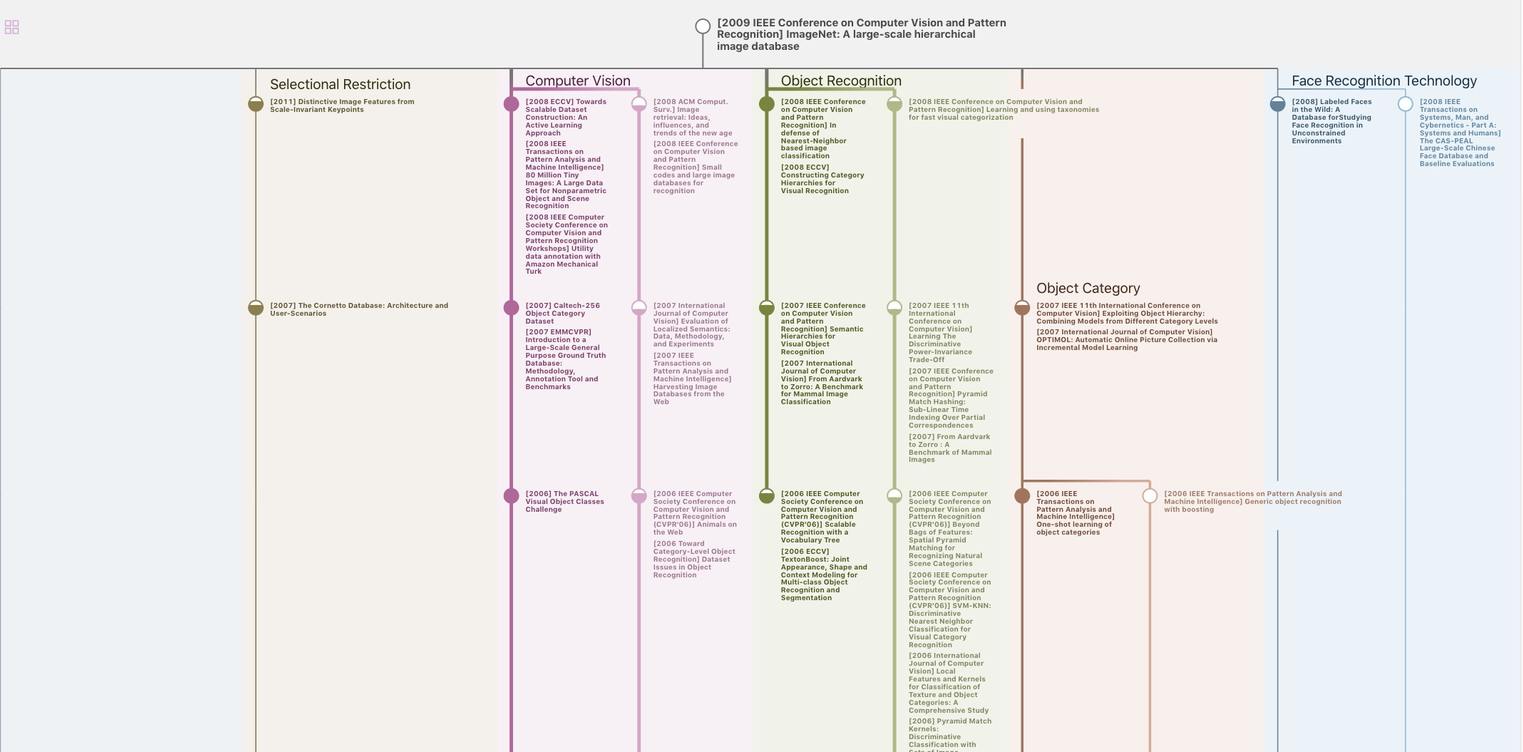
生成溯源树,研究论文发展脉络
Chat Paper
正在生成论文摘要