Novel pricing strategies for revenue maximization and demand learning using an exploration–exploitation framework
SOFT COMPUTING(2021)
摘要
The price demand relation is a fundamental concept that models how price affects the sale of a product. It is critical to have an accurate estimate of its parameters, as it will impact the company’s revenue. The learning has to be performed very efficiently using a small window of a few test points, because of the rapid changes in price demand parameters due to seasonality and fluctuations. However, there are conflicting goals when seeking the two objectives of revenue maximization and demand learning, known as the learn/earn trade-off. This is akin to the exploration/exploitation trade-off that we encounter in machine learning and optimization algorithms. In this paper, we consider the problem of price demand function estimation, taking into account its exploration–exploitation characteristic. We design a new objective function that combines both aspects. This objective function is essentially the revenue minus a term that measures the error in parameter estimates. Recursive algorithms that optimize this objective function are derived. The proposed method outperforms other existing approaches.
更多查看译文
关键词
Revenue management,Dynamic pricing,Demand learning,Exploration–exploitation trade-off,Price experimentation,Sequential decision problems
AI 理解论文
溯源树
样例
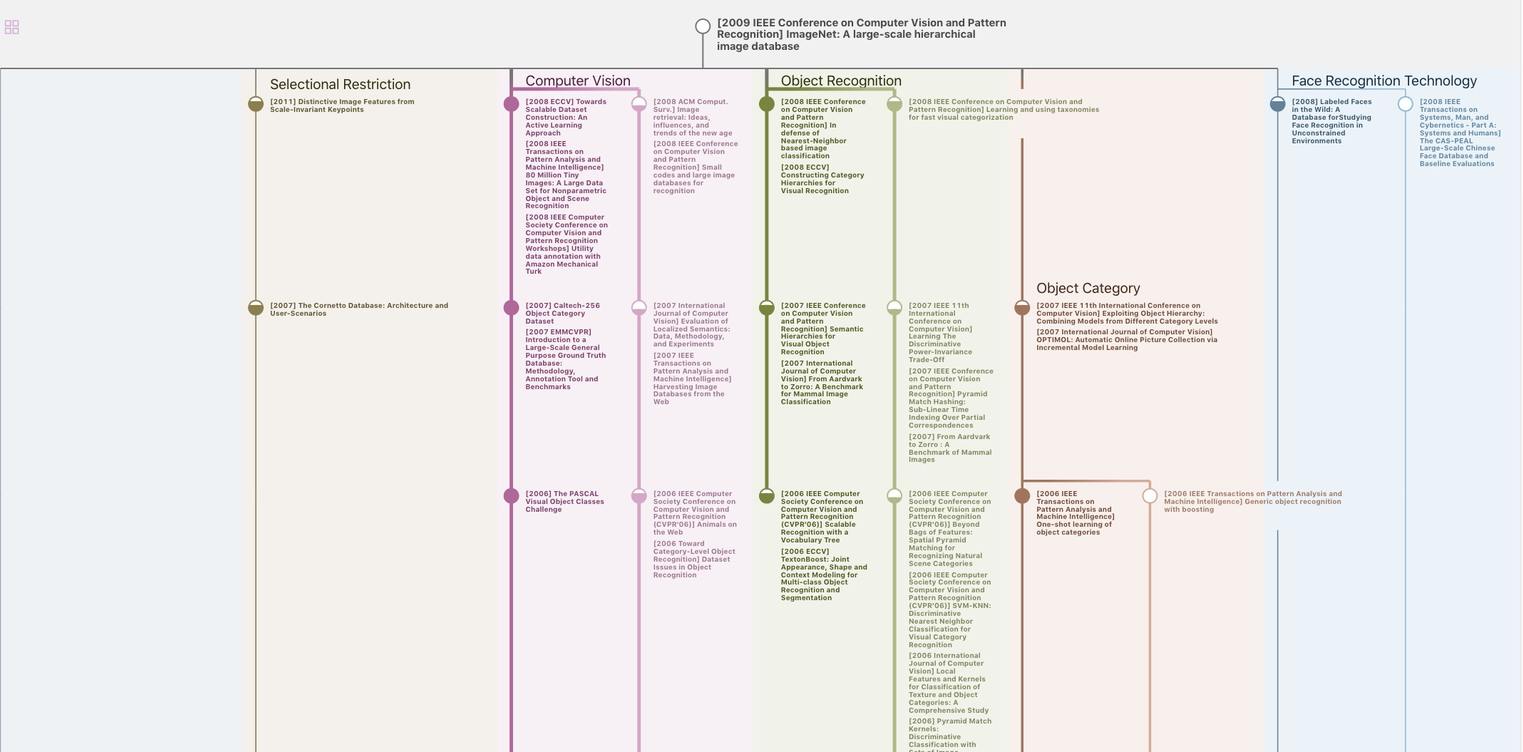
生成溯源树,研究论文发展脉络
Chat Paper
正在生成论文摘要