Estimation Of Patient Eye-Lens Dose During Neuro-Interventional Procedures Using A Dense Neural Network (Dnn)
MEDICAL IMAGING 2021: PHYSICS OF MEDICAL IMAGING(2021)
摘要
The patient's eye-lens dose changes for each projection view during fluoroscopically-guided neuro-interventional procedures. Monte-Carlo (MC) simulation can be done to estimate lens dose but MC cannot be done in real-time to give feedback to the interventionalist. Deep learning (DL) models were investigated to estimate patient-lens dose for given exposure conditions to give real-time updates. MC simulations were done using a Zubal computational phantom to create a dataset of eye-lens dose values for training the DL models. Six geometric parameters (entrance-field size, LAO gantry angulation, patient x, y, z head position relative to the beam isocenter, and whether patient's right or left eye) were varied for the simulations. The dose for each combination of parameters was expressed as lens dose per entrance air kerma (mGy/Gy). Geometric parameter combinations associated with high-dose values were sampled more finely to generate more high-dose values for training purposes. Additionally, dose at intermediate parameter values was calculated by MC in order to validate the interpolation capabilities of DL. Data was split into training, validation and testing sets. Stacked models and median algorithms were implemented to create more robust models. Model performance was evaluated using mean absolute percentage error (MADE). The goal for this DL model is that it be implemented into the Dose Tracking System (DTS) developed by our group. This would allow the DTS to infer the patient's eye-lens dose for real-time feedback and eliminate the need for a large database of pre-calculated values with interpolation capabilities.
更多查看译文
关键词
DNN, Eye-Lens Dose, Neuro-Interventional Procedures, DTS
AI 理解论文
溯源树
样例
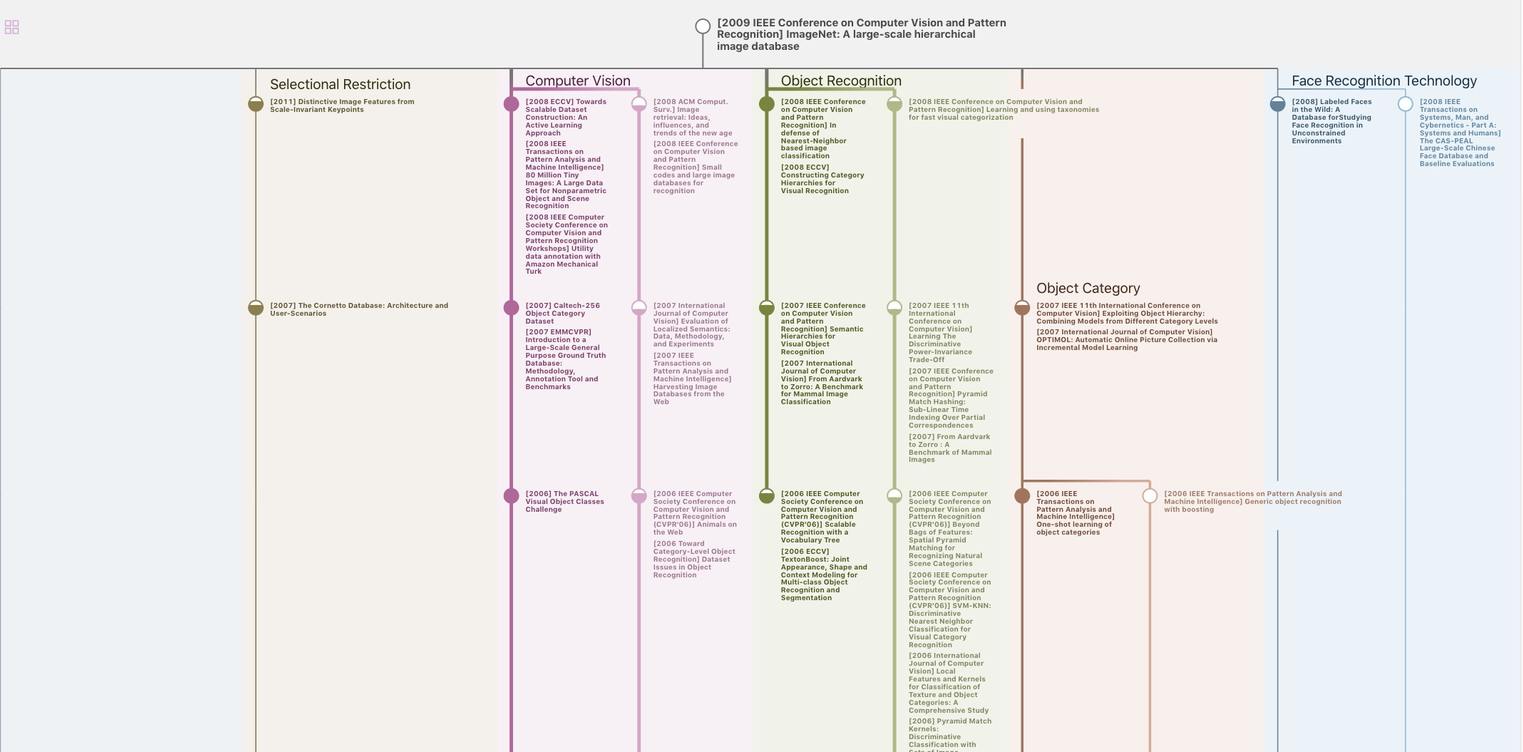
生成溯源树,研究论文发展脉络
Chat Paper
正在生成论文摘要