Online Estimation of Sparse Inverse Covariances
2021 AMERICAN CONTROL CONFERENCE (ACC)(2021)
摘要
Gaussian graphical models have been well studied as a way to represent the relationships between various entities, and numerous algorithms have been proposed to learn the dependencies in such models. However, these algorithms process data in a batch, and may not be suitable for real-time estimation. In this paper, we propose an online sparse inverse covariance algorithm to infer the network structure (i.e., dependencies between nodes) in real-time from time-series data. Our approach is based on an alternating minimization algorithm and allows users to select the number of iterations per data point. We provide theoretical guarantees showing that the online estimates converge to that of the batch mode as the number of data increases and characterize its asymptotic rate of convergence. Finally, we evaluate our online algorithm on synthetic data sets.
更多查看译文
关键词
data processing,alternating minimization algorithm,time series data,network structure,online sparse inverse covariance algorithm,Gaussian graphical models,online estimation
AI 理解论文
溯源树
样例
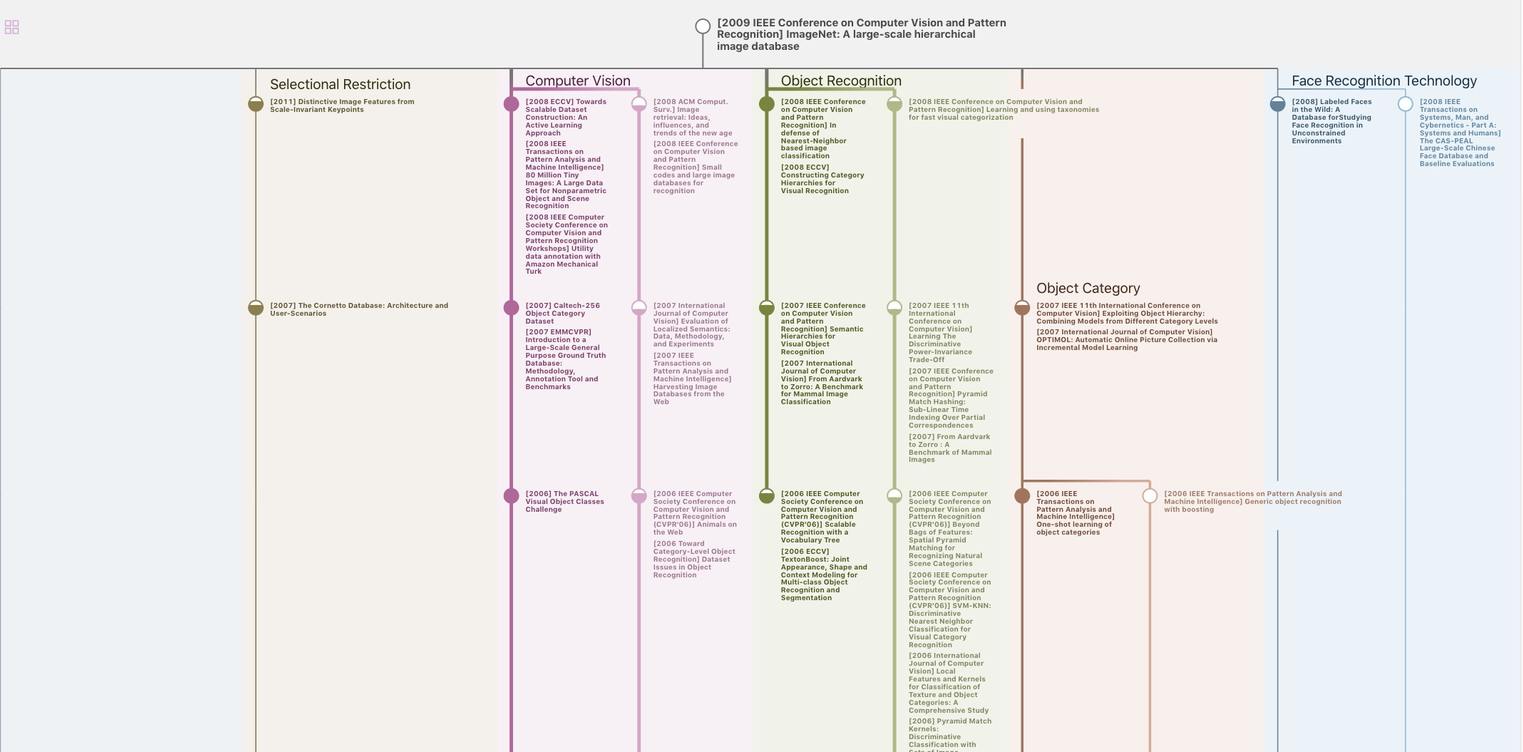
生成溯源树,研究论文发展脉络
Chat Paper
正在生成论文摘要