Regression Optimization for System-level Production Control
2021 AMERICAN CONTROL CONFERENCE (ACC)(2021)
Abstract
We propose a novel generalized prediction-optimization framework to optimize set point controls for a network of processes in a production plant, wherein a regression model is used to capture the physical representation of each process's behavior and the relationship between its inputs and outputs. We introduce a nonlinear optimization problem to model the optimal set-point problem. For piece-wise linear regressors, we reformulate the problem into a mixed-integer linear program. For highly nonlinear models such as deep neural networks, we propose a decomposition primal-dual algorithm for solving it. Using a real-world use case of oil sands processing, we show the benefit of our approach by the ability to efficiently identify a set of feasible control variables, while giving a high production output.
MoreTranslated text
Key words
regression optimization,system-level production control,set point controls,production plant,regression model,physical representation,nonlinear optimization problem,optimal set-point problem,piece-wise linear regressors,mixed-integer linear program,highly nonlinear models,deep neural networks,decomposition primal-dual algorithm,oil sands processing,feasible control variables,high production output,generalized prediction-optimization framework
AI Read Science
Must-Reading Tree
Example
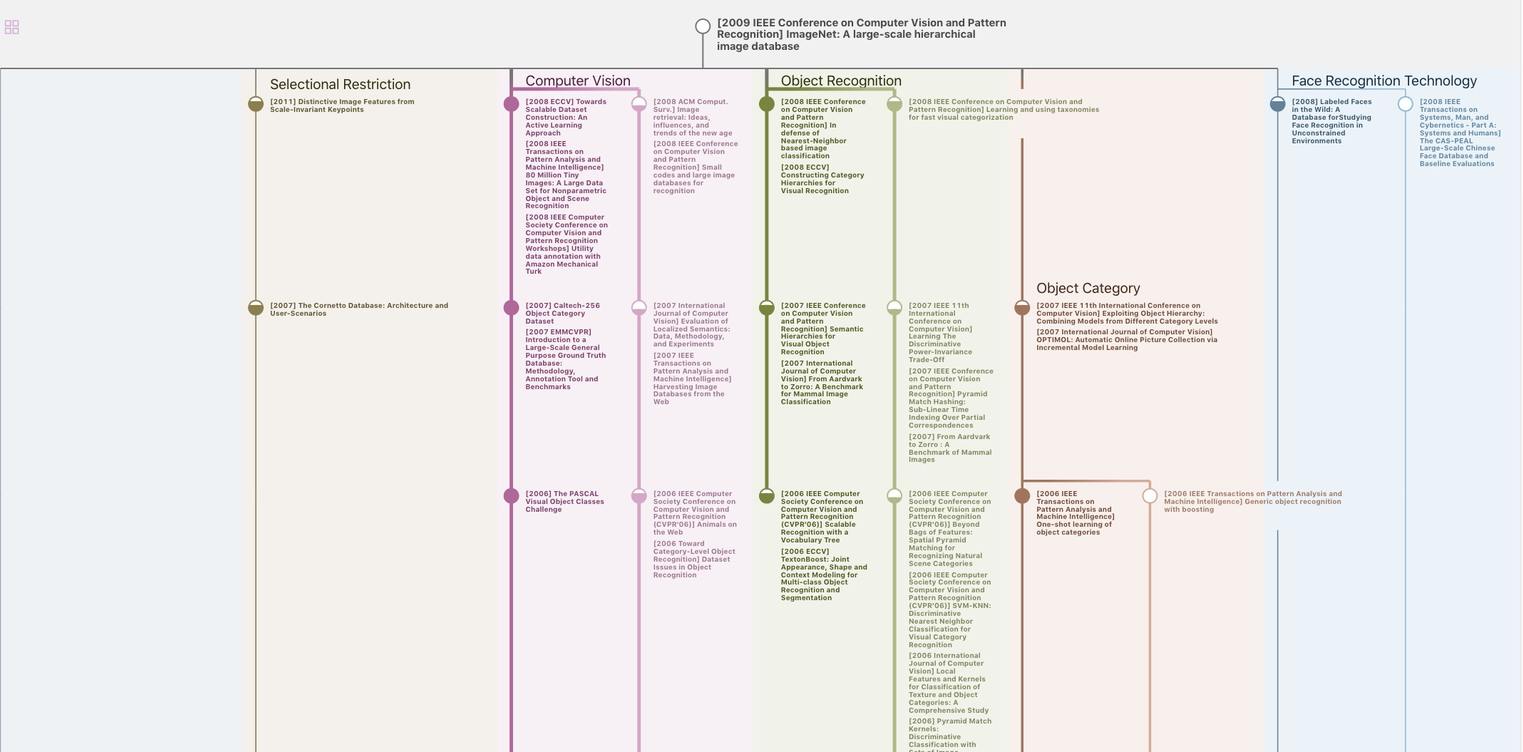
Generate MRT to find the research sequence of this paper
Chat Paper
Summary is being generated by the instructions you defined