A Method For Quantifying The Impacts Of Human Activities On Net Primary Production Of Grasslands In Northwest China
REMOTE SENSING(2021)
Abstract
Accurately assessing the impact of human activities on net primary productivity (NPP) of vegetation is of great significance to the achievement of sustainable development. However, it is difficult to disentangle the effects of climate conditions and human activities on NPP, and bridging this knowledge gap largely depends on the calculation of the NPP under natural conditions. Here, we propose a method for calculating natural vegetation NPP (NNPP) based on non-human influence grids, which are obtained according to the consistent rate of climate and actual NPP (ANPP) temporal changes. We selected Northwest China as study area, and we used a light use efficiency (LUE) model to estimate ANPP and used the random forest algorithm (RF) to estimate the NNPP. The results show that NNPP is very close to ANPP, and the human activities on NPP (HNPP) based on NNPP is close to the actual situation of human activities on NPP. From 2001 to 2017, the positive HNPP accounts for 40.28% of the total grassland area, with an average value of 28.65 gC center dot m(-2)center dot yr(-1), while the negative HNPP accounts for 59.72% of the total area, with an average value of -31.19 gC center dot m(-2)center dot yr(-1). The grassland NPP shows an increasing trend, which is dominated by climate factors. Human activity is the dominant factor for the grassland degradation, accounting for 42.78% of the degraded area, but promoting grassland growth in 11.4% of the restored area. This study provides a new method to estimate the impacts of human activities on vegetation, and the results can be used to evaluate the effectiveness of ecological environmental governance, providing a quantitative basis for scientifically building the harmonious relationship between human and nature.
MoreTranslated text
Key words
nature net primary productivity, human activities, light use efficiency model, random forest, Northwest China
AI Read Science
Must-Reading Tree
Example
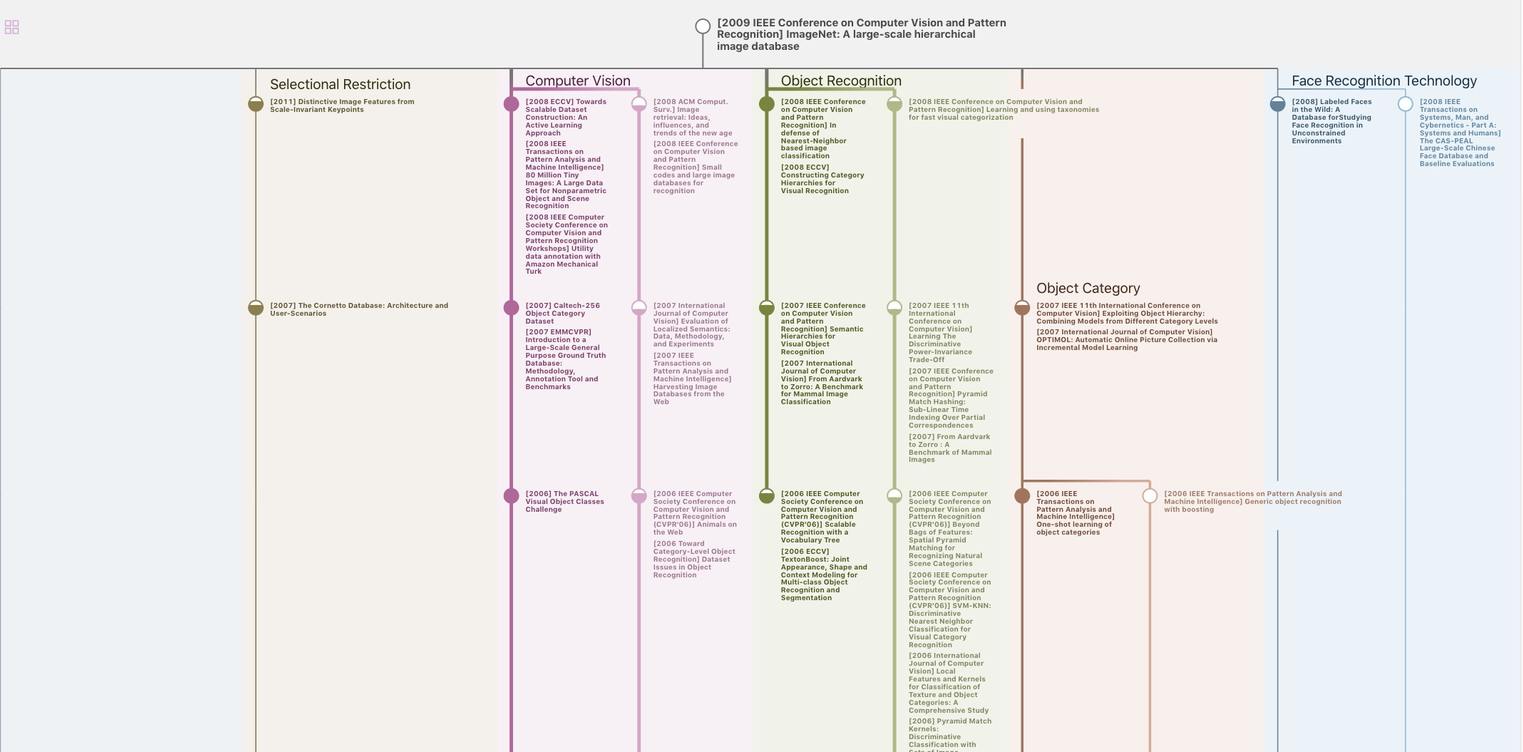
Generate MRT to find the research sequence of this paper
Chat Paper
Summary is being generated by the instructions you defined