Psychometric properties of the Chinese version of the Courtauld Emotional Control Scale in women newly diagnosed with breast cancer
Quality of Life Research(2021)
Abstract
Purpose Emotional control, the attempt to suppress the expression of negative effects, is an essential factor in the prevalence of psychological distress in women with breast cancer. The Courtauld Emotional Control Scale (CECS) is a commonly used self-report tool for assessing emotional suppression in both clinical and general groups. This study aimed to validate the Chinese version of the Courtauld Emotional Control Scale (CECS) in women newly diagnosed with breast cancer. Methods The study involved 680 women newly diagnosed with breast cancer aged 25 to 76 (mean age = 48.19, standard deviation (SD) = 8.57) from Changsha (China). Data analysis included Cronbach's alpha coefficients, the intraclass correlation coefficient (ICC), Pearson's correlations, Independent-Samples T test, confirmatory factor analysis (CFA) and exploratory structural equation modeling (ESEM) were conducted to determine the optimal model. For the best fitting model stability was assessed with tests for invariance across age, educational level, and employment status. Results Internal consistency ( α = 0.987) and test–retest reliability (ICC = 0.715) of the CECS were presented. Results confirm the structure of the Chinese version of the CECS with 21 items divided into three dimensions, anger suppression (CECS_AG), depression suppression (CECS_MD), and anxiety suppression (CECS_AX). Convergent and known-groups validity were acceptable. Additionally, this model remained invariant across age, educational levels, and employment status. Conclusions The Chinese version of the CECS has good psychometric properties in terms of reliability and validity, remaining invariant across age, educational levels, and employment status in women newly diagnosed with breast cancer.
MoreTranslated text
Key words
Emotional suppression, Breast cancer, ESEM, Measurement invariance
AI Read Science
Must-Reading Tree
Example
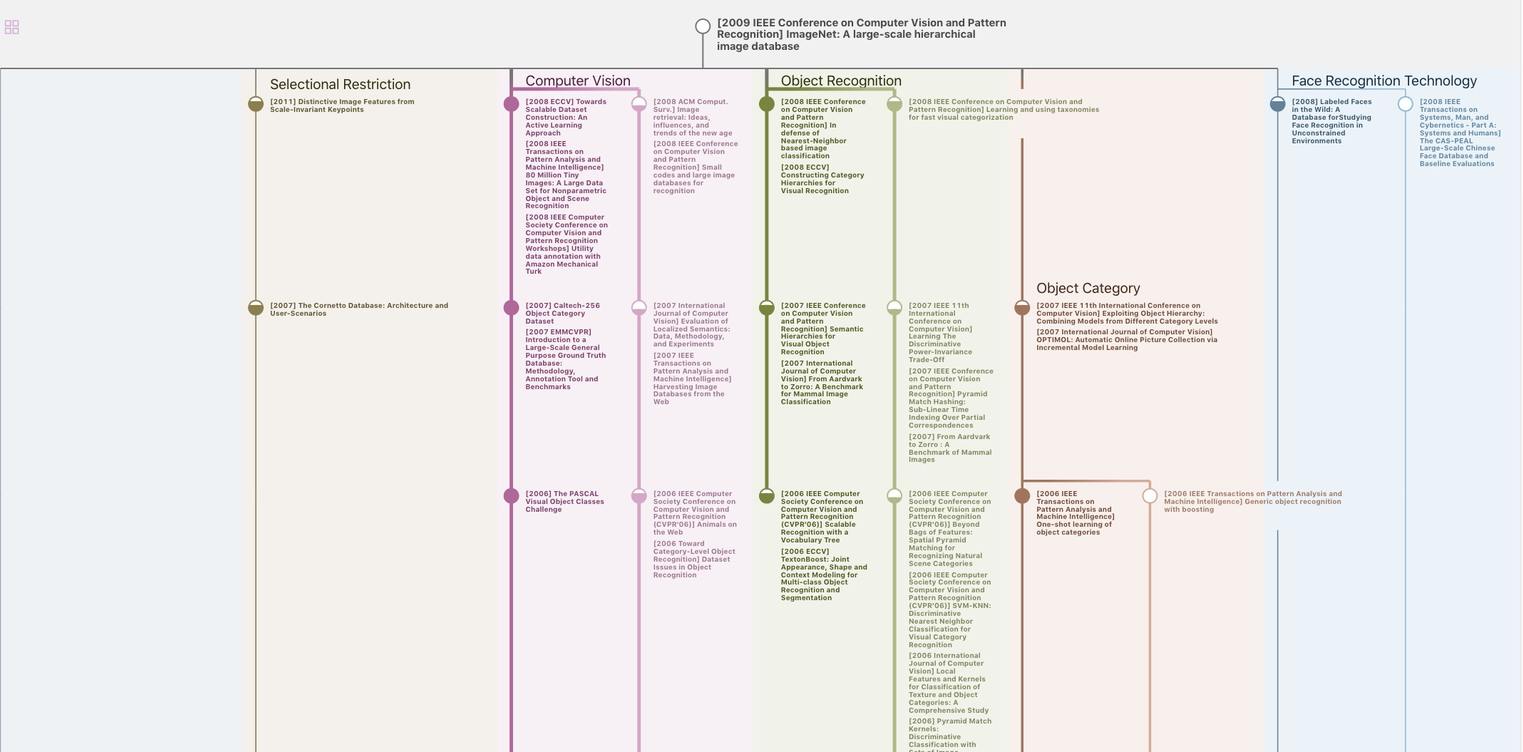
Generate MRT to find the research sequence of this paper
Chat Paper
Summary is being generated by the instructions you defined