What Matters for Adversarial Imitation Learning?
Annual Conference on Neural Information Processing Systems(2021)
Abstract
Adversarial imitation learning has become a popular framework for imitation in continuous control. Over the years, several variations of its components were proposed to enhance the performance of the learned policies as well as the sample complexity of the algorithm. In practice, these choices are rarely tested all together in rigorous empirical studies.It is therefore difficult to discuss and understand what choices, among the high-level algorithmic options as well as low-level implementation details, matter. To tackle this issue, we implement more than 50 of these choices in a generic adversarial imitation learning frameworkand investigate their impacts in a large-scale study (>500k trained agents) with both synthetic and human-generated demonstrations. We analyze the key results and highlight the most surprising findings.
MoreTranslated text
Key words
Imitation,Empirical research,Adversarial system,Data science,Computer science,Control (linguistics),Imitation learning,Sample complexity
AI Read Science
Must-Reading Tree
Example
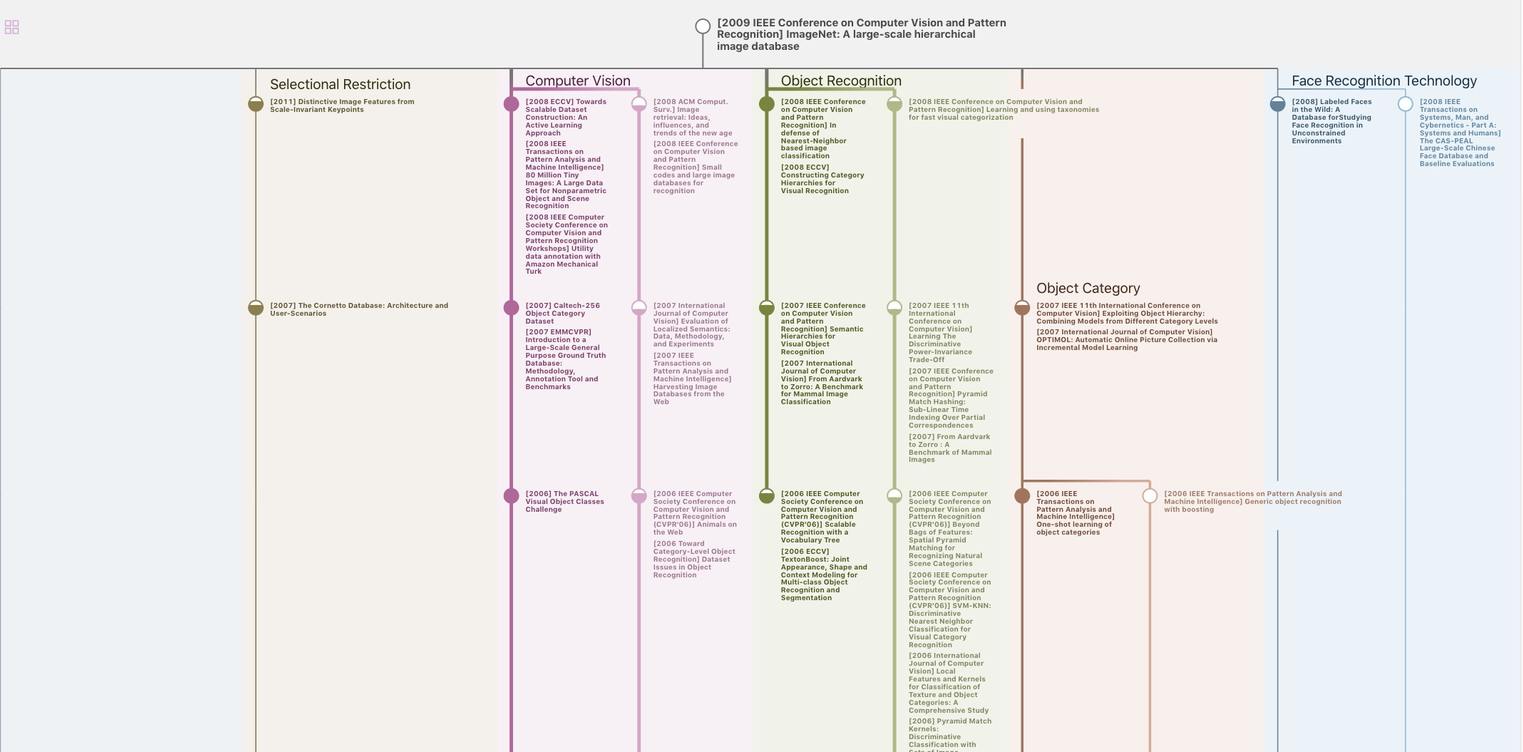
Generate MRT to find the research sequence of this paper
Chat Paper
Summary is being generated by the instructions you defined