Automatic Segmentation Of Brain Metastases Using T1 Magnetic Resonance And Computed Tomography Images
PHYSICS IN MEDICINE AND BIOLOGY(2021)
Abstract
An increasing number of patients with multiple brain metastases are being treated with stereotactic radiosurgery (SRS). Manually identifying and contouring all metastatic lesions is difficult and time-consuming, and a potential source of variability. Hence, we developed a 3D deep learning approach for segmenting brain metastases on MR and CT images. Five-hundred eleven patients treated with SRS were retrospectively identified for this study. Prior to radiotherapy, the patients were imaged with 3D T1 spoiled-gradient MR post-Gd (T1 + C) and contrast-enhanced CT (CECT), which were co-registered by a treatment planner. The gross tumor volume contours, authored by the attending radiation oncologist, were taken as the ground truth. There were 3 +/- 4 metastases per patient, with volume up to 57 ml. We produced a multi-stage model that automatically performs brain extraction, followed by detection and segmentation of brain metastases using co-registered T1 + C and CECT. Augmented data from 80% of these patients were used to train modified 3D V-Net convolutional neural networks for this task. We combined a normalized boundary loss function with soft Dice loss to improve the model optimization, and employed gradient accumulation to stabilize the training. The average Dice similarity coefficient (DSC) for brain extraction was 0.975 +/- 0.002 (95% CI). The detection sensitivity per metastasis was 90% (329/367), with moderate dependence on metastasis size. Averaged across 102 test patients, our approach had metastasis detection sensitivity 95 +/- 3%, 2.4 +/- 0.5 false positives, DSC of 0.76 +/- 0.03, and 95th-percentile Hausdorff distance of 2.5 +/- 0.3 mm (95% CIs). The volumes of automatic and manual segmentations were strongly correlated for metastases of volume up to 20 ml ( r=0.97, p<0.001 More
Translated text
Key words
deep learning, brain metastases, skull stripping, MRI, CECT, convolutional neural network, boundary loss
AI Read Science
Must-Reading Tree
Example
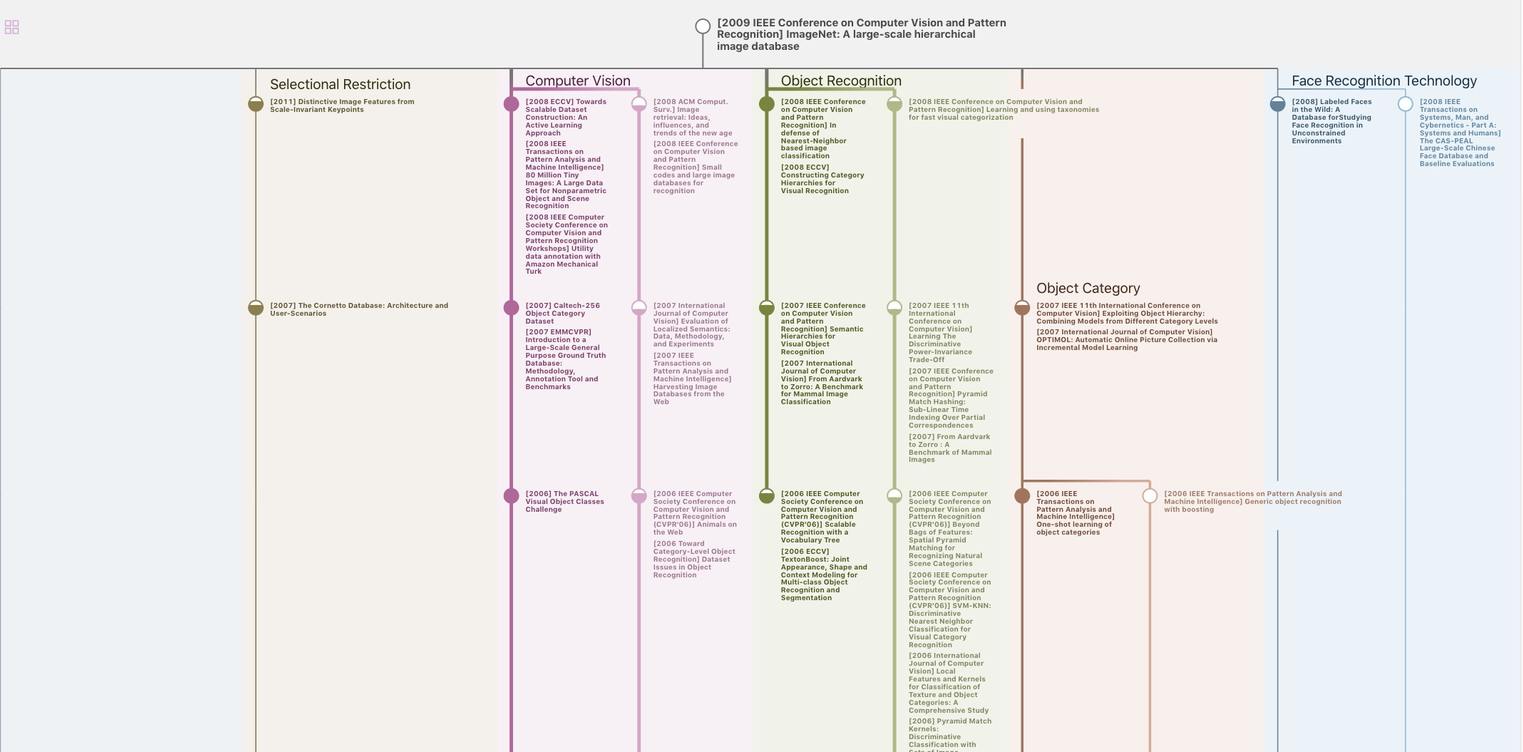
Generate MRT to find the research sequence of this paper
Chat Paper
Summary is being generated by the instructions you defined