To Pay Or Not To Pay Attention: Classifying And Interpreting Visual Selective Attention Frequency Features
EXPLAINABLE AND TRANSPARENT AI AND MULTI-AGENT SYSTEMS, EXTRAAMAS 2021(2021)
摘要
Selective attention is the ability to promote the processing of objects important for the accomplishment of our behavioral goals (target objects) over the objects not important to those goals (distractor objects). Previous investigations have shown that the mechanisms of selective attention contribute to enhancing perception in both simple daily tasks and more complex activities requiring learning new information.Recently, it has been verified that selective attention to target objects and distractor objects is separable in the frequency domain, using Logistic Regression (LR) and Support Vector Machines (SVMs) classification. However, discerning dynamics of target and distractor objects in the context of selective attention has not been accomplished yet.This paper extends the investigations on the possible classification and interpretation of distraction and intention solely relying on neural activity (frequency features). In particular, this paper (i) classifies distractor objects vs. target object replicating the LR classification of prior studies, extending the analysis by (ii) interpreting the coefficient weights relating to all features with a focus on N2PC features, and (iii) retrains an LR classifier with the features deemed important by the interpretation analysis.As a result of the interpretation methods, we have successfully decreased the feature size to 7.3% of total features - i.e., from 19,072 to 1,386 features - while recording only a 0.04 loss in performance accuracy score-i.e., from 0.65 to 0.61. Additionally, the interpretation of the classifiers' coefficient weights unveiled new evidence regarding frequency which has been discussed along with the paper.
更多查看译文
关键词
EEG, Selective attention, Machine learning
AI 理解论文
溯源树
样例
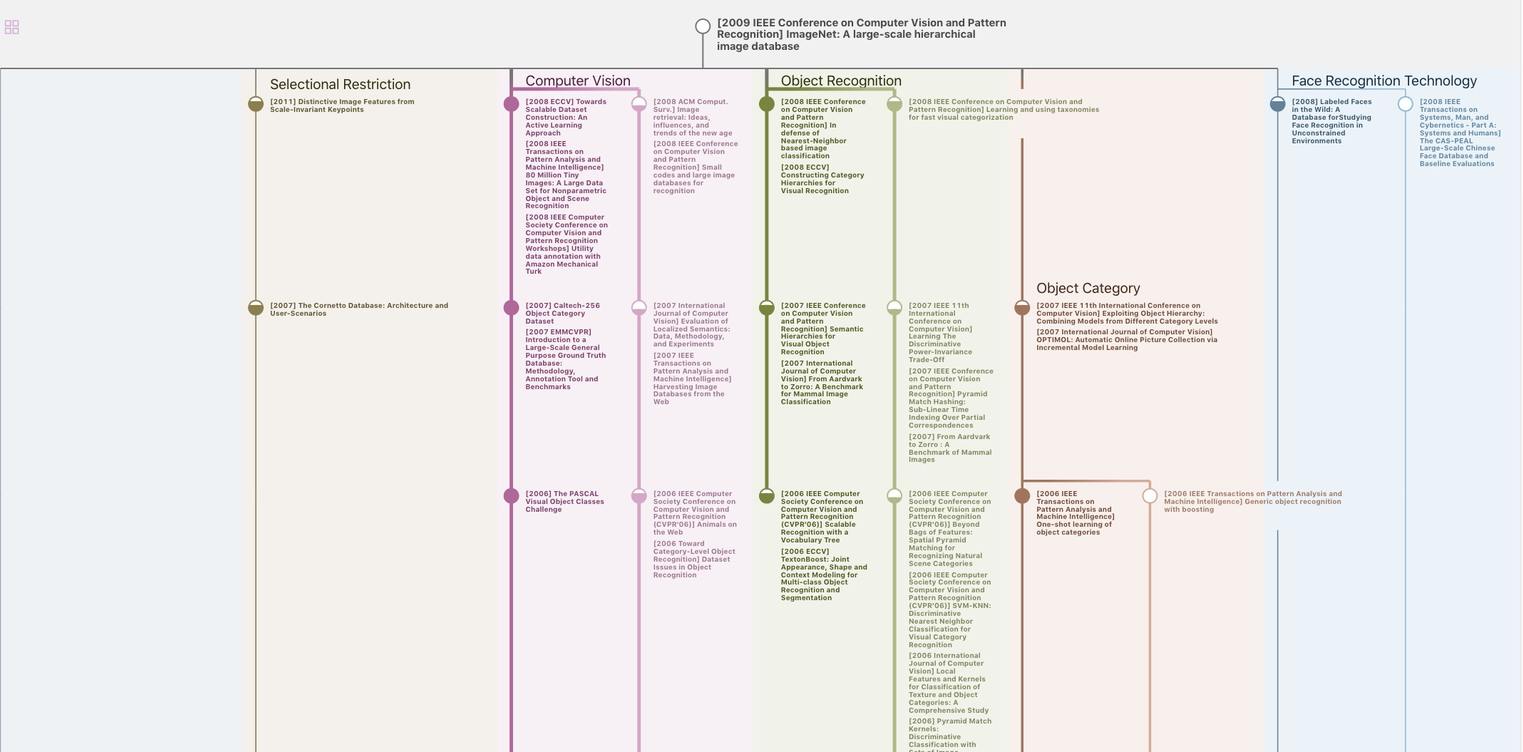
生成溯源树,研究论文发展脉络
Chat Paper
正在生成论文摘要