Forecasting Migration Intention Using Multivariate Time Series.
ICVISP(2020)
摘要
This paper aims to analyze international migrations in western African countries using irregular multivariate monthly time series containing a few values. Existing methods of filling in missing values have limitations because there are not enough values to infer them. In this study, we explore two approaches to solve this problem. One approach is to aggregate the values annually to eliminate missing values. The other is to use the Random Forest (RF) based approach to fill in the missing values. Then, we predict the international migration intentions using deep learning approaches and time series dataset. We demonstrate that a RF-based imputation outperforms a zero filling approach (used as the baseline) with Long Short-Term Memory (LSTM) method. Moreover, we show that analyzing the monthly subregion-based time series provides better insights than the yearly country-based time series.
更多查看译文
AI 理解论文
溯源树
样例
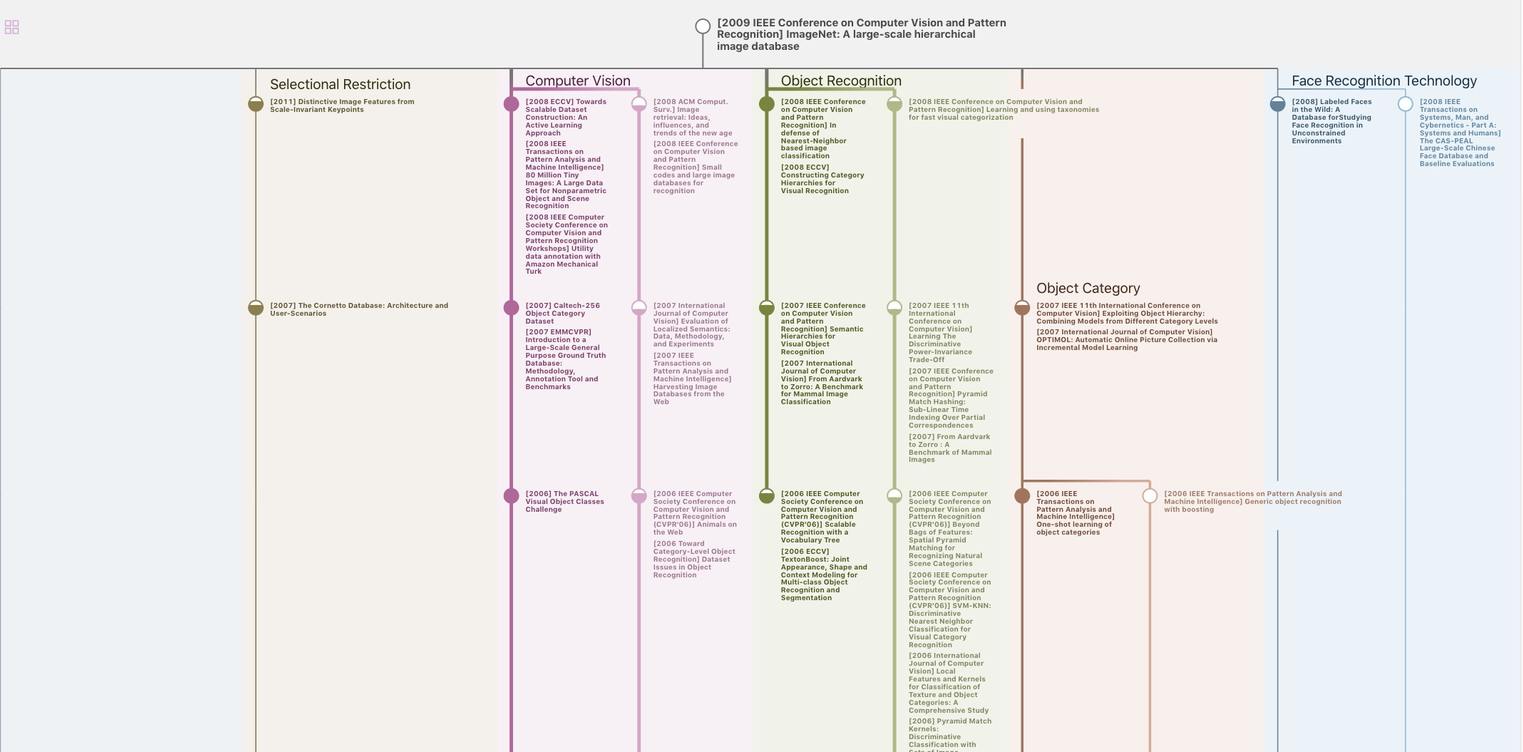
生成溯源树,研究论文发展脉络
Chat Paper
正在生成论文摘要