Combining Reward and Rank Signals for Slate Recommendation
arxiv(2021)
摘要
We consider the problem of slate recommendation, where the recommender system presents a user with a collection or slate composed of K recommended items at once. If the user finds the recommended items appealing then the user may click and the recommender system receives some feedback. Two pieces of information are available to the recommender system: was the slate clicked? (the reward), and if the slate was clicked, which item was clicked? (rank). In this paper, we formulate several Bayesian models that incorporate the reward signal (Reward model), the rank signal (Rank model), or both (Full model), for non-personalized slate recommendation. In our experiments, we analyze performance gains of the Full model and show that it achieves significantly lower error as the number of products in the catalog grows or as the slate size increases.
更多查看译文
关键词
slate recommendation,rank signals
AI 理解论文
溯源树
样例
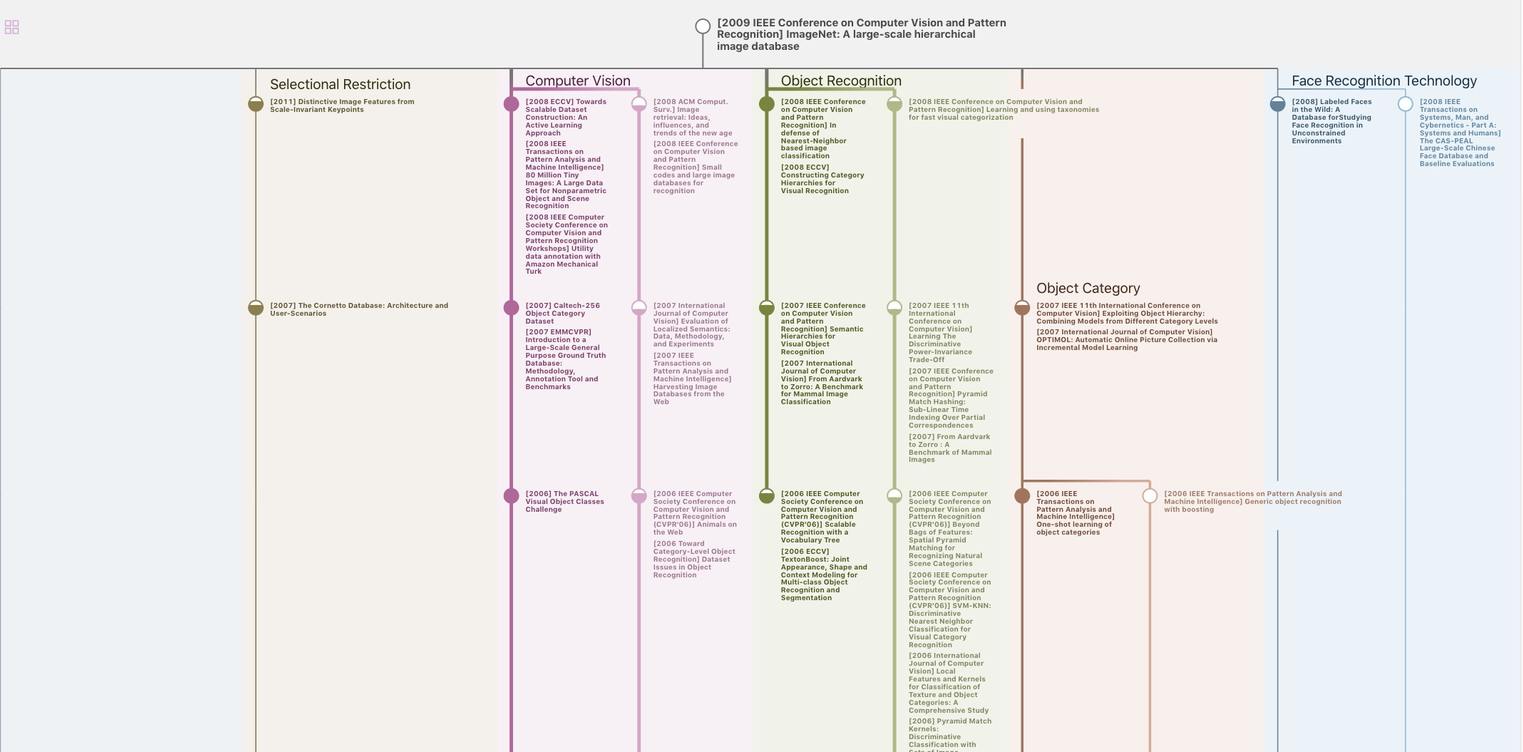
生成溯源树,研究论文发展脉络
Chat Paper
正在生成论文摘要