Development Of A Random Forest Model To Classify Sarcoidosis And Tuberculosis
AMERICAN JOURNAL OF TRANSLATIONAL RESEARCH(2021)
Abstract
Objective: To identify significant diagnostic factors and establish a predictive model for diagnosis of sarcoidosis and tuberculosis. Methods: This study included 252 patients (123 cases of lung sarcoidosis and 129 cases of lung tuberculosis) who underwent laboratory evaluation, including routine hematologic testing, serum immunology, blood coagulation, angiotensin-converting enzyme, and T lymphocyte subset. The factors that statistically different between the two groups were identified by an independent sample t test first, and then processed by the random forest model to distinguish two diseases with the classification function. Moreover, the diagnostic performance of the predictive random forest model was evaluated through the identification of individual contribution of various diagnostic factors conducted by the model. Results: The random forest model revealed a classification error rate of 24.9%. Among all of the statistically significant diagnostic factors, the individual factors with the greatest and second contribution were angiotensin-converting enzyme and prothrombin time, respectively. The area under the receiver operating characteristic (ROC) curve of the random forest prediction model was 0.915. Conclusion: The random forest model can be used to distinguish between sarcoidosis and tuberculosis by incorporating statistically significant diagnostic factors, which is of potential clinical application value.
MoreTranslated text
Key words
Random forest model, sarcoidosis, tuberculosis, differential diagnosis, ACE
AI Read Science
Must-Reading Tree
Example
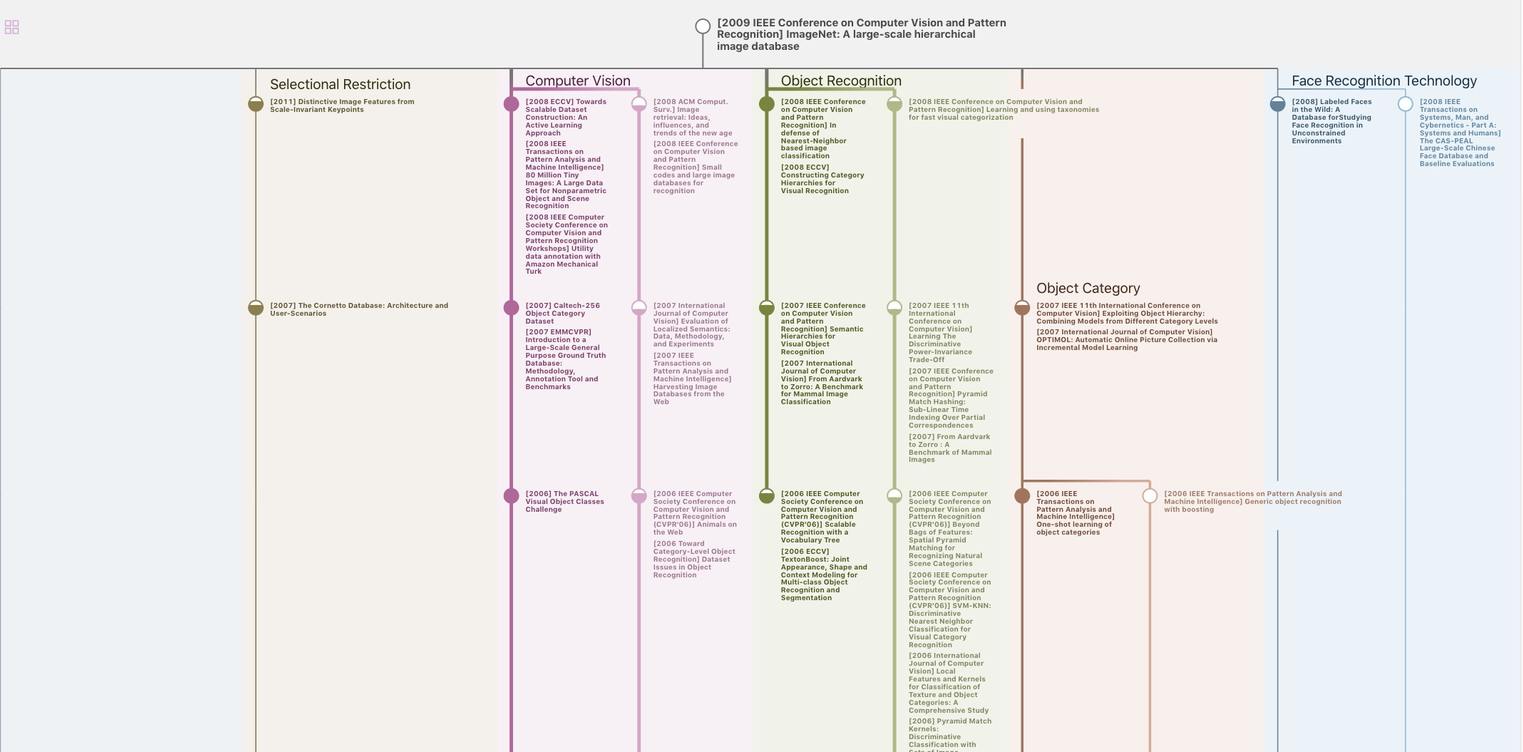
Generate MRT to find the research sequence of this paper
Chat Paper
Summary is being generated by the instructions you defined