Virtual Drive-Tests: A Case for Predicting QoE in Adaptive Video Streaming
arxiv(2021)
摘要
Intelligent and autonomous troubleshooting is a crucial enabler for the current 5G and future 6G networks. In this work, we develop a flexible architecture for detecting anomalies in adaptive video streaming comprising three main components: i) A pattern recognizer that learns a typical pattern for video quality from the client-side application traces of a specific reference video, ii) A predictor for mapping Radio Frequency (RF) performance indicators collected on the network-side using user-based traces to a video quality measure, iii) An anomaly detector for comparing the predicted video quality pattern with the typical pattern to identify anomalies. We use real network traces (i.e., on-device measurements) collected in different geographical locations and at various times of day to train our machine learning models. We perform extensive numerical analysis to demonstrate key parameters impacting correct video quality prediction and anomaly detection. In particular, we have shown that the video playback time is the most crucial parameter determining the video quality since buffering continues during the playback and resulting in better video quality further into the playback. However, we also reveal that RF performance indicators characterizing the quality of the cellular connectivity are required to correctly predict QoE in anomalous cases. Then, we have exhibited that the mean maximum F1-score of our method is 77%, verifying the efficacy of our models. Our architecture is flexible and autonomous, so one can apply it to -- and operate with -- other user applications as long as the relevant user-based traces are available.
更多查看译文
关键词
qoe,adaptive video streaming,drive-tests
AI 理解论文
溯源树
样例
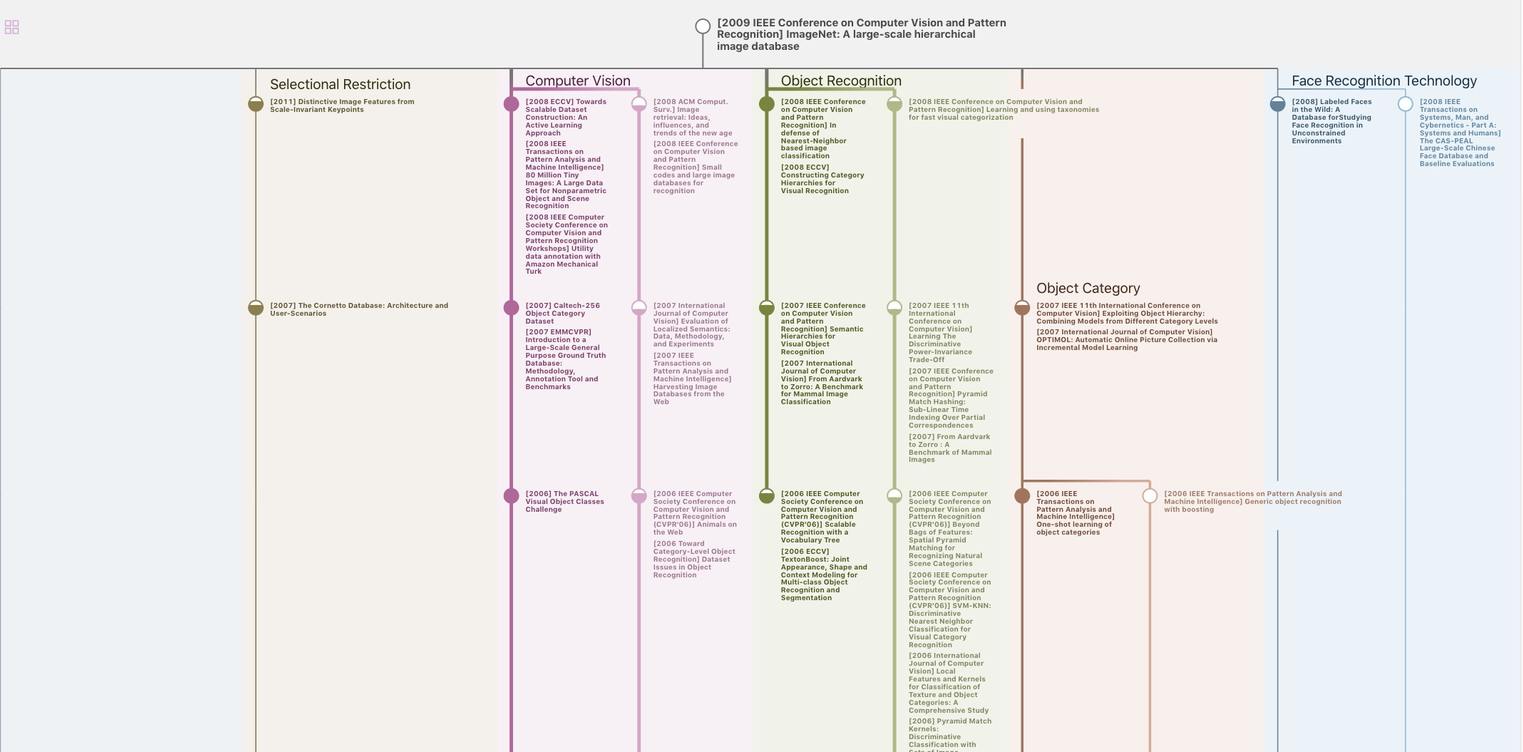
生成溯源树,研究论文发展脉络
Chat Paper
正在生成论文摘要