Content-driven music recommendation: Evolution, state of the art, and challenges
COMPUTER SCIENCE REVIEW(2024)
摘要
The music domain is among the most important ones for adopting recommender systems technology. In contrast to most other recommendation domains, which predominantly rely on collaborative filtering (CF) techniques, music recommenders have traditionally embraced content-based (CB) approaches. In the past years, music recommendation models that leverage collaborative and content data - which we refer to as content-driven models - have been replacing pure CF or CB models. In this survey, we review 55 articles on content-driven music recommendation. Based on a thorough literature analysis, we first propose an onion model comprising five layers, each of which corresponds to a category of music content we identified: signal, embedded metadata, expert-generated content, user-generated content, and derivative content. We provide a detailed characterization of each category along several dimensions. Second, we identify six overarching challenges, according to which we organize our main discussion: increasing recommendation diversity and novelty, providing transparency and explanations, accomplishing context-awareness, recommending sequences of music, improving scalability and efficiency, and alleviating cold start. Each article addresses one or more of these challenges and is categorized according to the content layers of our onion model, the article's goal(s), and main methodological choices. Furthermore, articles are discussed in temporal order to shed light on the evolution of content-driven music recommendation strategies. Finally, we provide our personal selection of the persisting grand challenges which are still waiting to be solved in future research endeavors.
更多查看译文
关键词
Music,Recommender Systems,Onion model
AI 理解论文
溯源树
样例
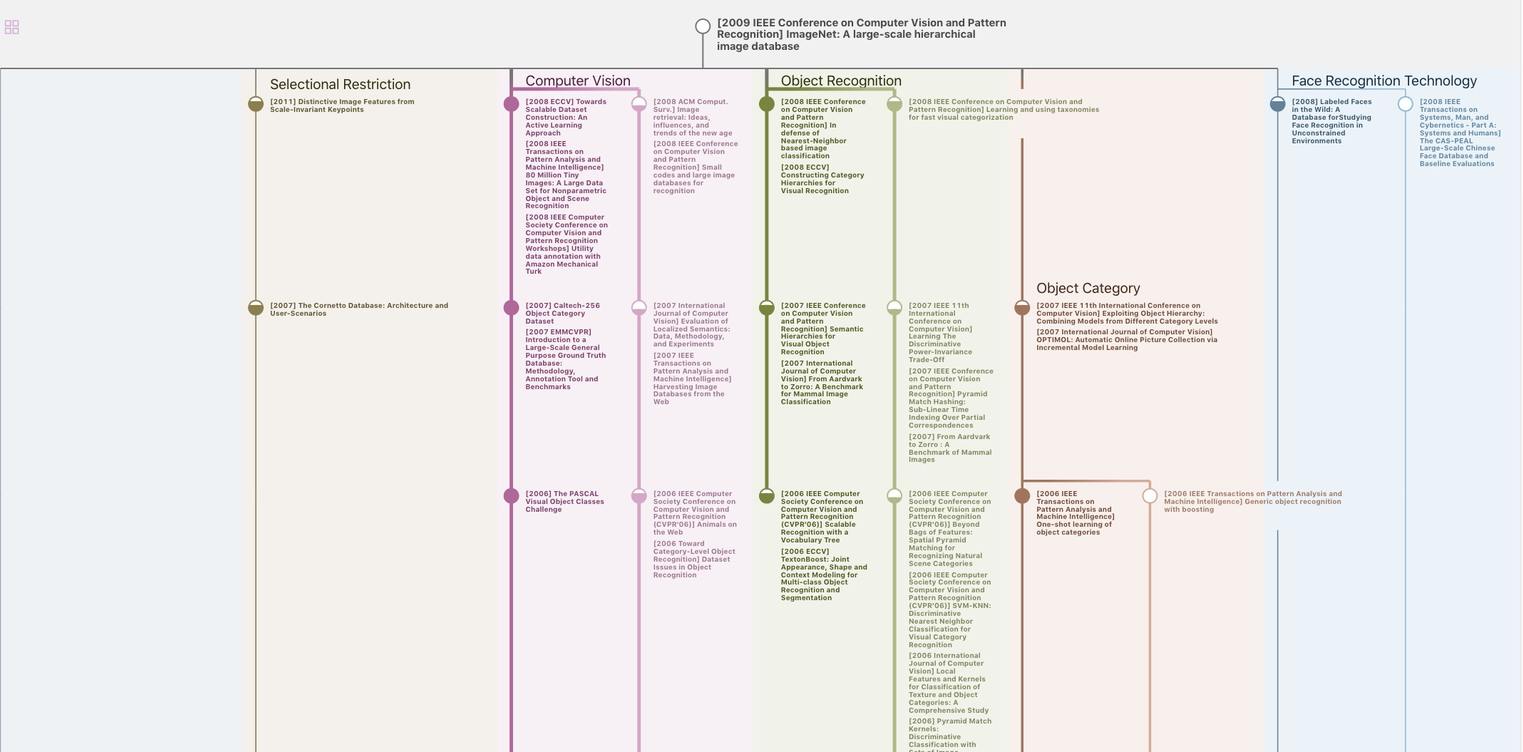
生成溯源树,研究论文发展脉络
Chat Paper
正在生成论文摘要