An instance-dependent simulation framework for learning with label noise
arxiv(2022)
摘要
We propose a simulation framework for generating instance-dependent noisy labels via a pseudo-labeling paradigm. We show that the distribution of the synthetic noisy labels generated with our framework is closer to human labels compared to independent and class-conditional random flipping. Equipped with controllable label noise, we study the negative impact of noisy labels across a few practical settings to understand when label noise is more problematic. We also benchmark several existing algorithms for learning with noisy labels and compare their behavior on our synthetic datasets and on the datasets with independent random label noise. Additionally, with the availability of annotator information from our simulation framework, we propose a new technique, Label Quality Model (LQM), that leverages annotator features to predict and correct against noisy labels. We show that by adding LQM as a label correction step before applying existing noisy label techniques, we can further improve the models’ performance. The synthetic datasets that we generated in this work are released at https://github.com/deepmind/deepmind-research/tree/master/noisy_label .
更多查看译文
关键词
Noisy labels,Simulation,Datasets,Rater features
AI 理解论文
溯源树
样例
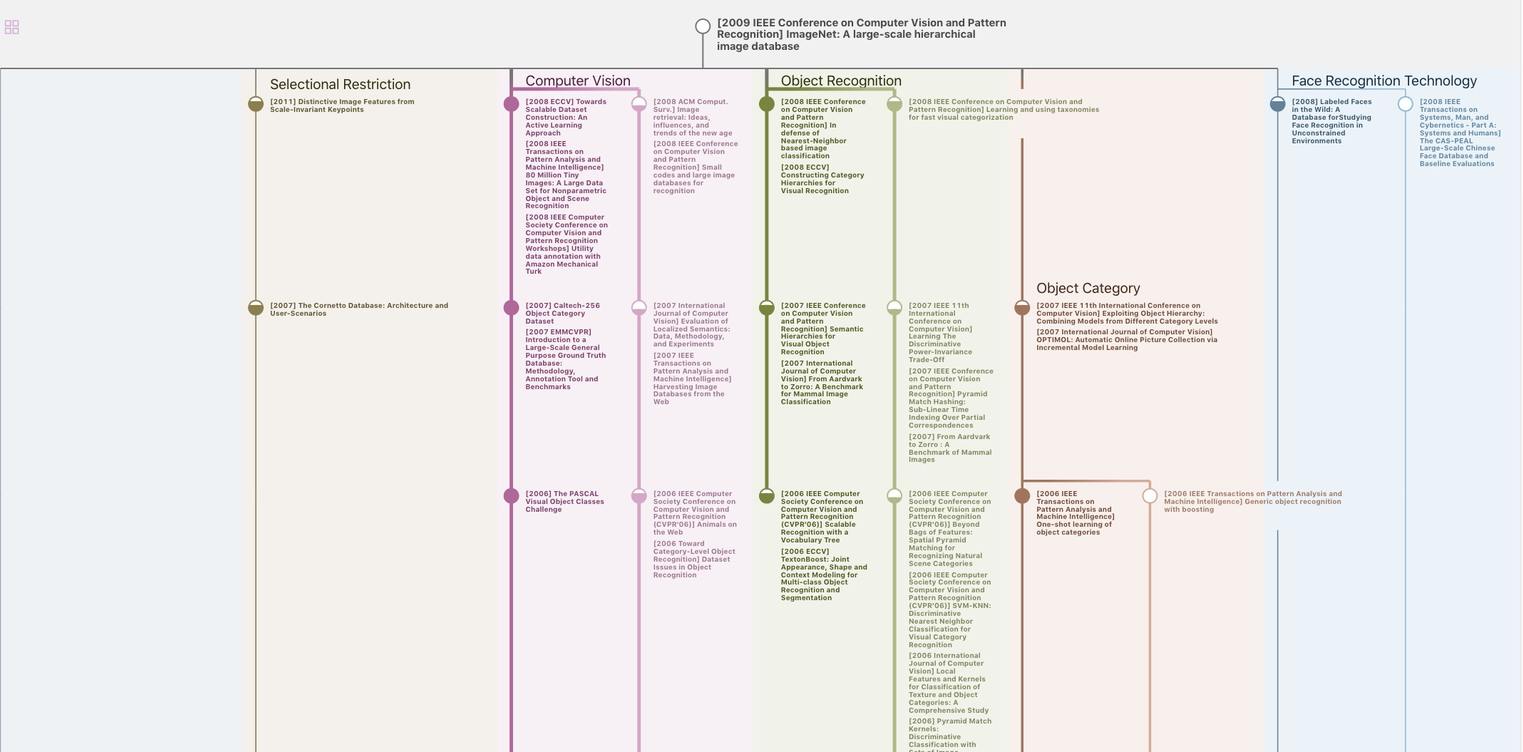
生成溯源树,研究论文发展脉络
Chat Paper
正在生成论文摘要