An Evaluation of the Layers of a Deep Network on the Optical Character Recognition Problem.
SIU(2021)
摘要
Various layers of Convolutional Neural Networks, one of the most common methods of deep learning, have been examined by many researchers, and methods that will increase performance and reduce the complexity of computing have been proposed in classification using this architecture. In this paper, we investigate, over the Optical Character Recognition problem, which layers does the deep architecture owe its high performance in classification. For this purpose, we evaluated the effectiveness of the first layers of deep architecture by classifying the features extracted from deep architecture with a Support Vector Machine. Then, we evaluated the effects of these methods in classification by applying the Fully Connected Layer and Global Average Pooling Layer methods in the last layers of the deep neural network. Experiments pointed out that the deep network owes its performance to all of its layers, but alternative solutions on the upper layers of the architecture can reduce the computational complexity without a significant change in the performance..
更多查看译文
关键词
convolutional netral network,global average pooling,optical character recognition
AI 理解论文
溯源树
样例
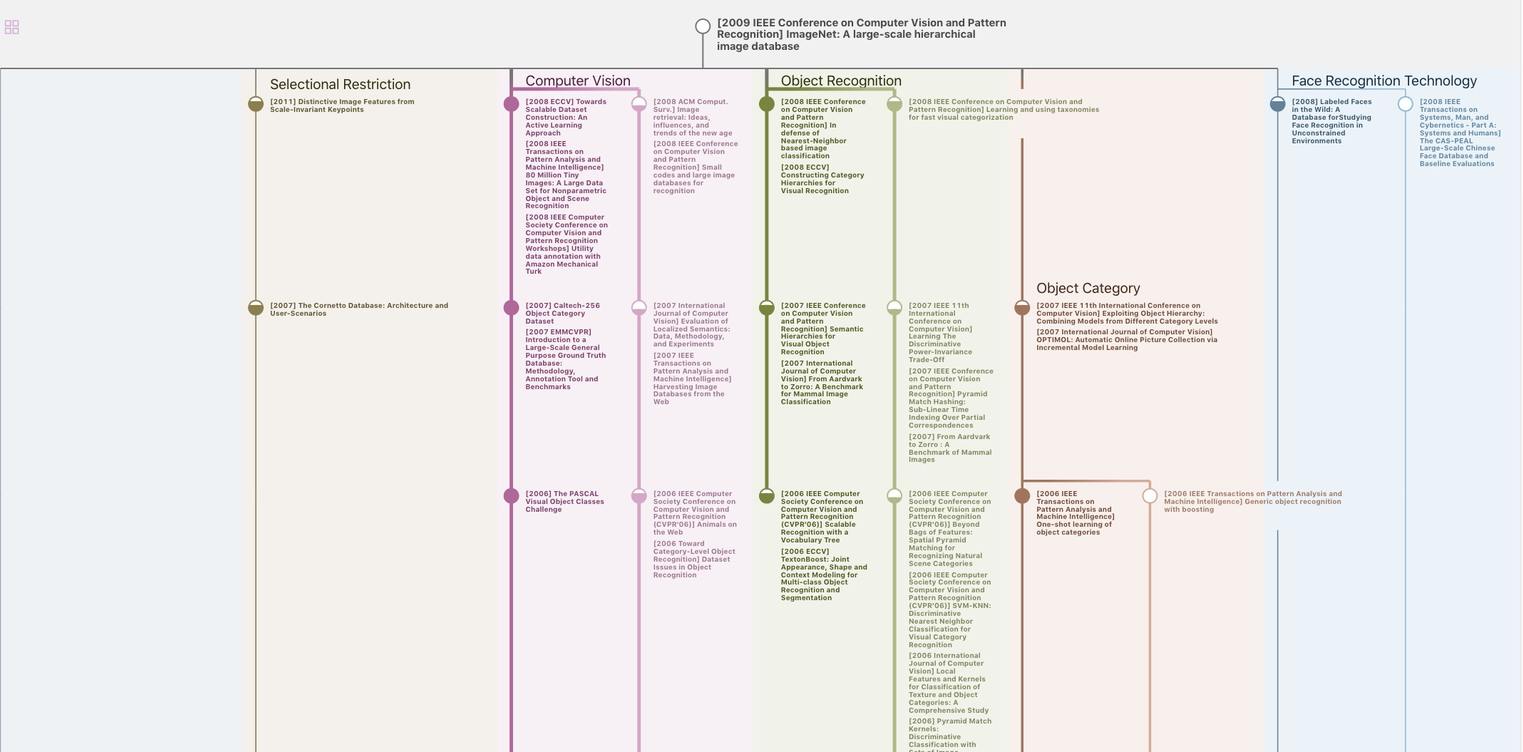
生成溯源树,研究论文发展脉络
Chat Paper
正在生成论文摘要