Destek Vektör Makinesi ile Yeni Oz Benzerlik İndeksi Kullanarak Epilepsi Tespiti Epilepsy Detection Using a Novel Self Similarity Index Combined with Support Vector Machine
SIU(2021)
Abstract
Epilepsy is a severe neurological disease that can result in life threatening situations. Therefore, accurate, reliable, and robust epilepsy diagnostic systems is of high importance. The design of such systems involves feature extraction and machine learning (ML) models. Thus, we introduce a novel Self-Similarity Index (SSI) feature based on Hjorth complexity and Katz fractal dimension. SSI is explored individually and in combination with other features extracted from both raw EEG signal and Wavelet Package Decomposition (WPD) coefficients. Several ML models are tested on the Bonn dataset to identify the most suitable model for classifying data into 3 classes - healthy, ictal, and inter-ictal. We show that the proposed SSI is superior to other features when used in a single-feature Support Vector Machine (SVM) classifier and yields 81.80% accuracy. This result is further improved to 99.00% when SSI is combined with 24 other commonly extracted features. Thus, SSI is a promising feature that allows for high classification accuracy with limited data and number of channels.
MoreTranslated text
Key words
Epilepsy,Fractal Dimension,Self Similarity Index,Support Vector Machine,Wavelet Package Decomposition
AI Read Science
Must-Reading Tree
Example
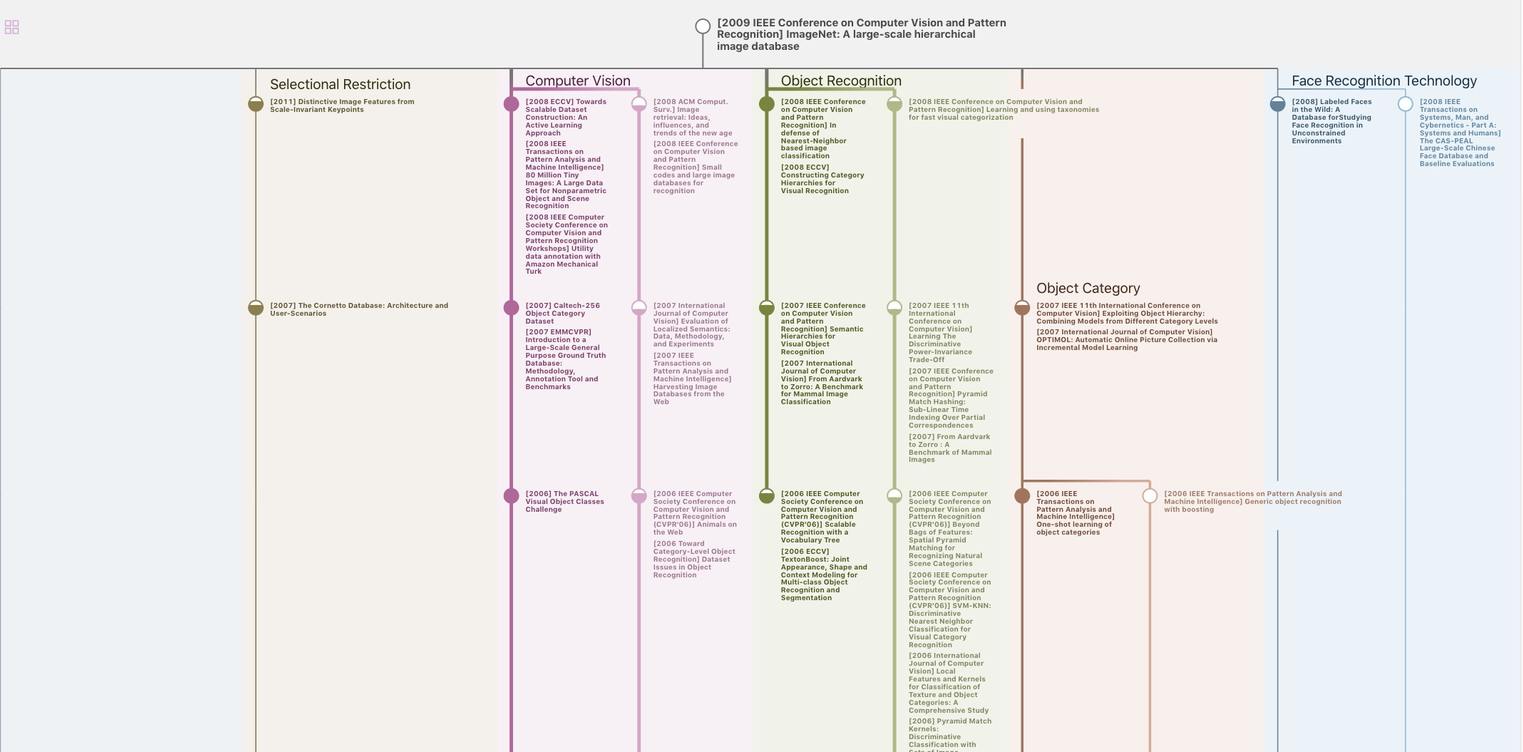
Generate MRT to find the research sequence of this paper
Chat Paper
Summary is being generated by the instructions you defined