On the Distribution of ML Workloads to the Network Edge and Beyond
IEEE CONFERENCE ON COMPUTER COMMUNICATIONS WORKSHOPS (IEEE INFOCOM WKSHPS 2021)(2021)
摘要
The emerging paradigm of edge computing has revolutionized network applications, delivering computational power closer to the end-user. Consequently, Machine Learning (ML) tasks, typically performed in a data centre (Centralized Learning - CL), can now be offloaded to the edge (Edge Learning - EL) or mobile devices (Federated Learning - FL). While the inherent flexibility of such distributed schemes has drawn considerable attention, a thorough investigation on their resource consumption footprint is still missing. In our work, we consider a FL scheme and two EL variants, representing varying proximity to the end users (data sources) and corresponding levels of workload distribution across the network; namely Access Edge Learning (AEL), where edge nodes are essentially co-located with the base stations and Regional Edge Learning (REL), where they lie towards the network core. Based on real systems' measurements and user mobility traces, we devise a realistic simulation model to evaluate and compare the performance of the considered ML schemes under an image classification task. Our results indicate that FL and EL can act as viable alternatives to CL. Edge learning effectiveness is shaped by the configuration of edge nodes in the network with REL achieving the prominent combination of accuracy and bandwidth needs. Energy-wise, edge learning is shown to offer an attractive choice (for involved stakeholders) to offload centralised ML tasks.
更多查看译文
关键词
end users,data sources,workload distribution,Access Edge Learning,edge nodes,base stations,Regional Edge Learning,network core,considered ML schemes,image classification task,Edge learning effectiveness,ML tasks,ML workloads,network Edge,edge computing,network applications,computational power,end-user,Machine Learning tasks,data centre,Centralized Learning - CL,Edge Learning - EL,Federated Learning,inherent flexibility,distributed schemes,resource consumption footprint,FL scheme,EL variants
AI 理解论文
溯源树
样例
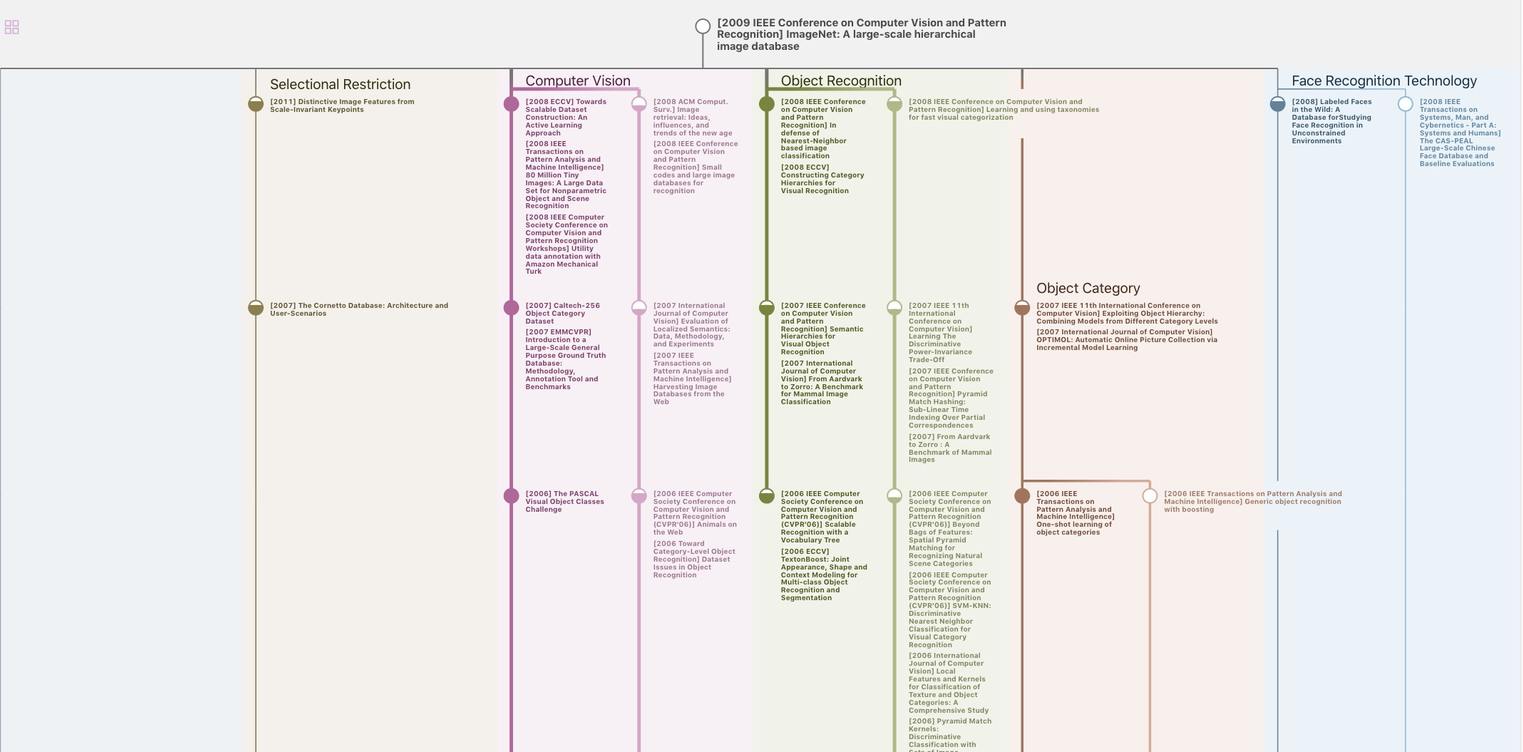
生成溯源树,研究论文发展脉络
Chat Paper
正在生成论文摘要