Resource Allocation for Low-Latency NOMA-V2X Networks Using Reinforcement Learning
IEEE CONFERENCE ON COMPUTER COMMUNICATIONS WORKSHOPS (IEEE INFOCOM WKSHPS 2021)(2021)
Abstract
With the development of the Internet of things (IoT), vehicle-to-everything (V2X) plays an essential role in wireless communication networks. Vehicular communications meet tremendous challenges in guaranteeing low-latency transmission for safety-critical information due to dynamic channels caused by high mobility. To handle the challenges, non-orthogonal multiple access (NOMA) has been considered as a promising candidate for future V2X networks. However, it is still an open issue on how to organize multiple transmission links with suitable resource allocation. In this paper, we investigate the problem of the resource allocation for the low-latency NOMA-integrated V2X (NOMA-V2X) communication networks. First, a cross-layer optimization problem is formulated to consider user scheduling and power allocation jointly while satisfying the quality-of-service (QoS) requirements, including the delay requirements, rate demands, and power constraints. To cope with the limited time-varying channel information, a machine learning based resource allocation algorithm is proposed to find solutions. Specifically, reinforcement learning is applied to learn the dynamic channel information for reducing the transmission delay. The numerical results indicate that our proposed algorithm can significantly reduce the system delay compared with other methods while satisfying the QoS requirements, so as to tackle the congestion issues for V2X communications.
MoreTranslated text
Key words
Machine Learning, NOMA, Reinforcement Learning, Resource Allocation, Vehicular Networks, V2X
AI Read Science
Must-Reading Tree
Example
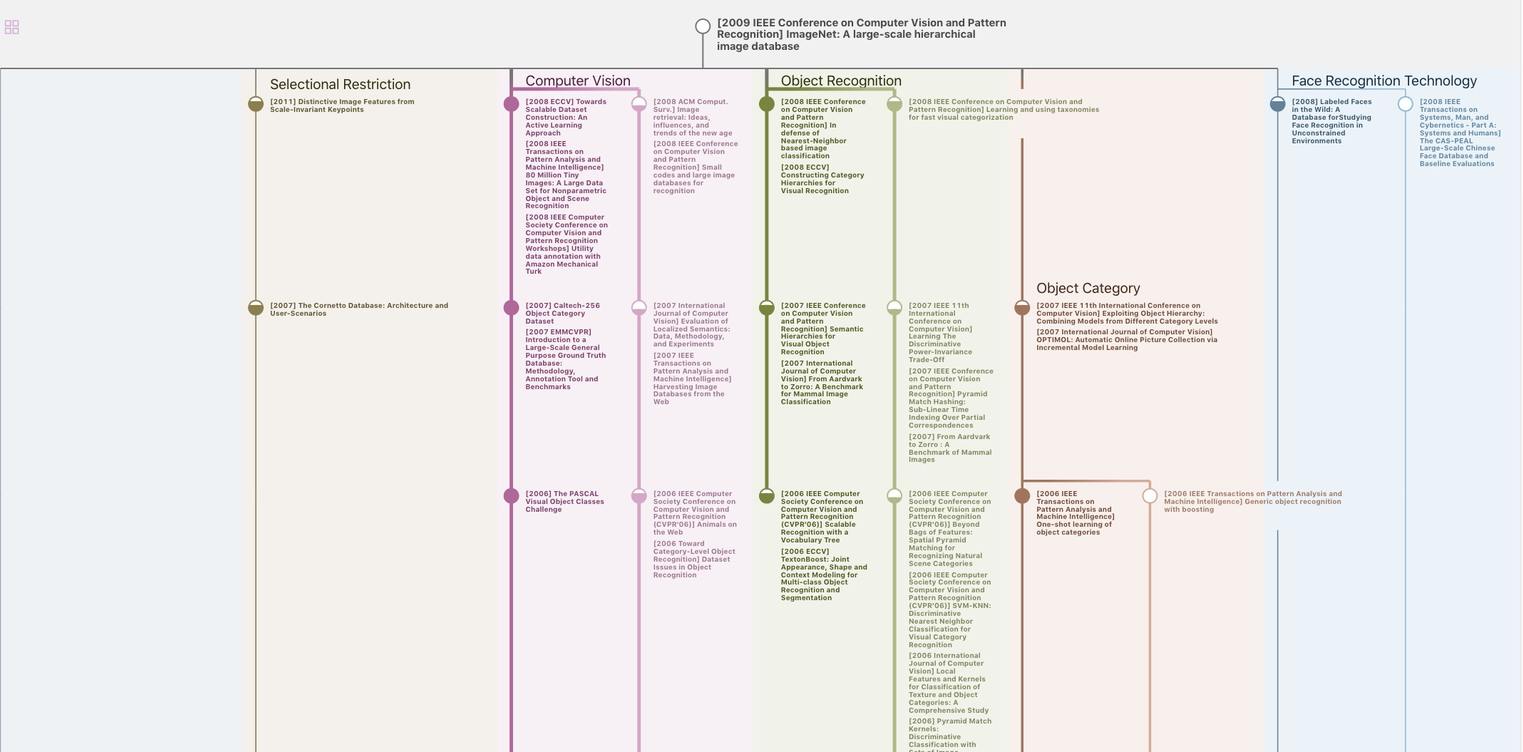
Generate MRT to find the research sequence of this paper
Chat Paper
Summary is being generated by the instructions you defined