RamFace: Race Adaptive Margin Based Face Recognition for Racial Bias Mitigation
2021 IEEE International Joint Conference on Biometrics (IJCB)(2021)
摘要
Recent studies show that there exist significant racial bias among state-of-the-art (SOTA) face recognition algorithms, i.e., the accuracy for Caucasian is consistently higher than that for other races like African and Asian. To mitigate racial bias, we propose the race adaptive margin based face recognition (RamFace) model, designed under the multi-task learning framework with the race classification as the auxiliary task. The experiments show that the race classification task can enforce the model to learn the racial features and thus improve the discriminability of the extracted feature representations. In addition, a racial bias robust loss function, i.e., race adaptive margin loss, is proposed such that different optimal margins can be automatically derived for different races in training the model, which further mitigates the racial bias. The experimental results show that on RFW dataset, our model not only achieves SOTA face recognition accuracy but also mitigates the racial bias problem. Besides, RamFace is also tested on several public face recognition evaluation benchmarks, i.e., LFW, CPLFW and CALFW, and achieves better performance than the commonly used face recognition methods, which justifies the generalization capability of RamFace.
更多查看译文
关键词
race adaptive margin based face recognition model,Asian race,African race,Caucasian race,CALFW,CPLFW,RFW dataset,state-of-the-art face recognition algorithms,public face recognition evaluation benchmarks,racial bias problem,SOTA face recognition accuracy,race adaptive margin loss,racial bias robust loss function,extracted feature representations,racial features,race classification task,multitask learning framework,racial bias mitigation,RamFace
AI 理解论文
溯源树
样例
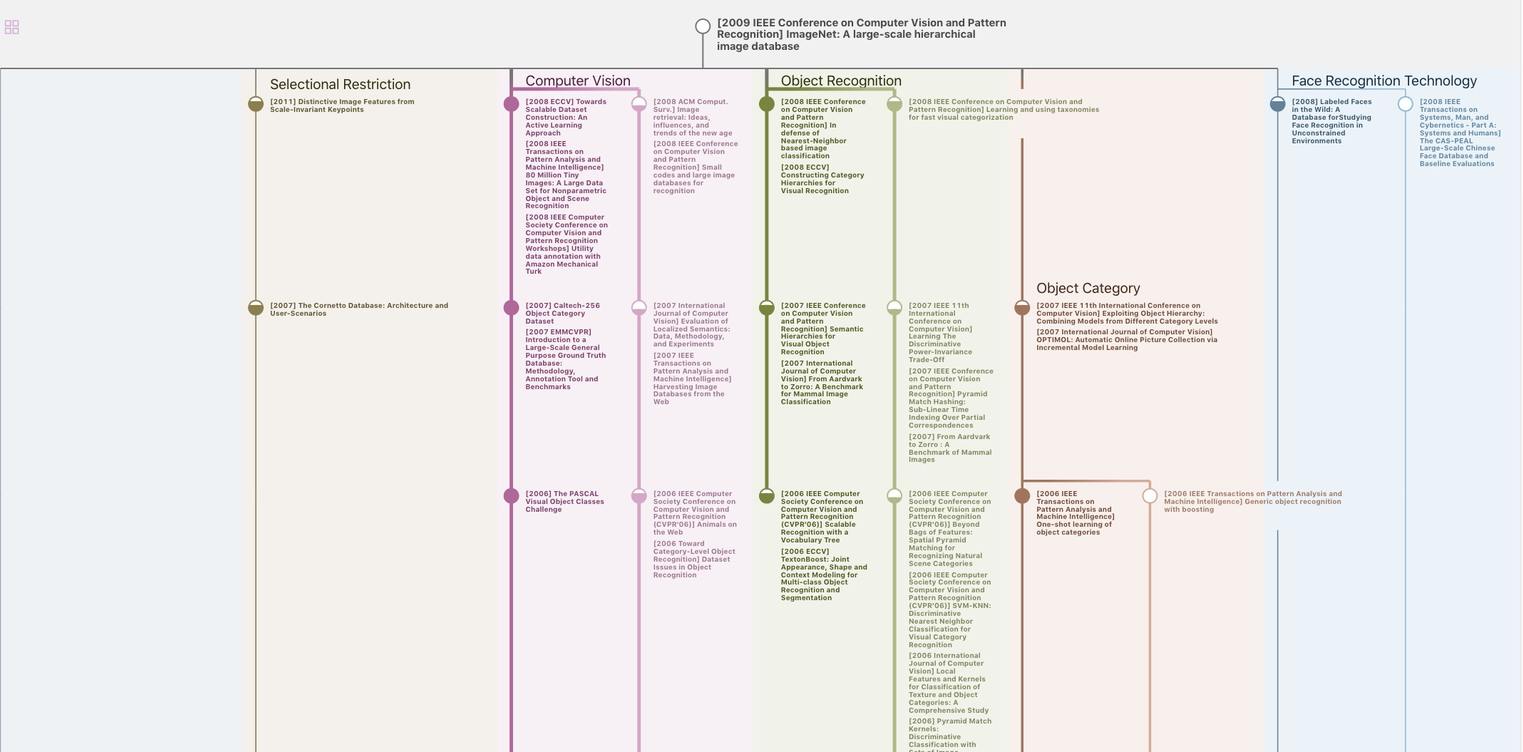
生成溯源树,研究论文发展脉络
Chat Paper
正在生成论文摘要