Machine Learning Emulation of Parameterized Gravity Wave Momentum Fluxes in an Atmospheric Global Climate Model
user-5fe1a78c4c775e6ec07359f9(2021)
摘要
<p>We present a novel, single-column gravity wave parameterization (GWP) that uses machine learning to emulate a physics-based GWP. An artificial neural network (ANN) is trained with output from an idealized atmospheric model and tested in an offline environment, illustrating that an ANN can learn the salient features of gravity wave momentum transport directly from resolved flow variables. We demonstrate that when trained on the westward phase of the Quasi-Biennial Oscillation, the ANN can skillfully generate the momentum fluxes associated with the eastward phase. We also show that the meridional and zonal wind components are the only flow variables necessary to predict horizontal momentum fluxes with a globally and temporally averaged R^2 value over 0.8. State-of-the-art GWPs are severely limited by computational constraints and a scarcity of observations for validation. This work constitutes a significant step towards obtaining observationally validated, computationally efficient GWPs in global climate models.</p>
更多查看译文
关键词
Momentum (technical analysis),Emulation,Gravity wave,Parameterized complexity,Mechanics,Physics,General Circulation Model
AI 理解论文
溯源树
样例
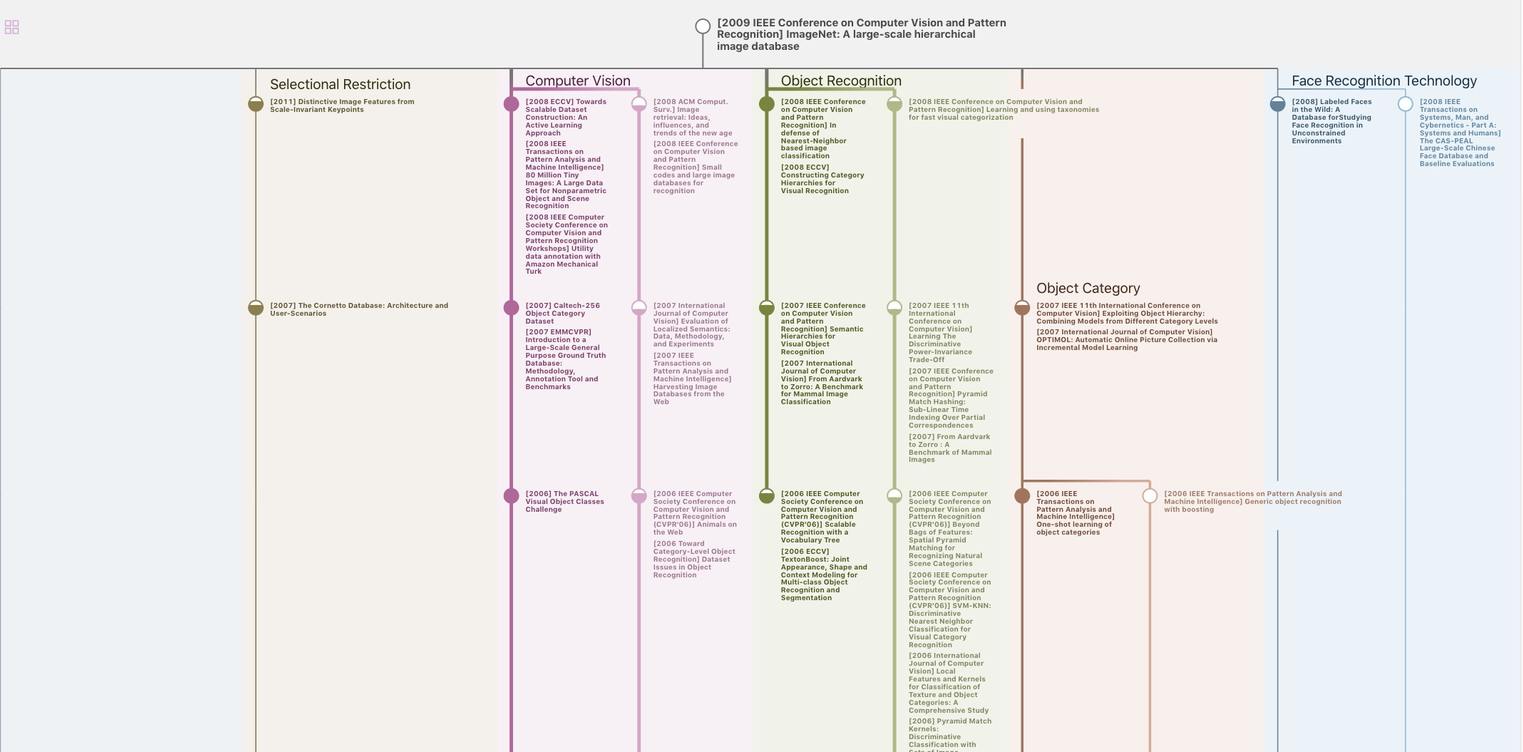
生成溯源树,研究论文发展脉络
Chat Paper
正在生成论文摘要