Multitask-based joint learning approach to robust ASR for radio communication speech
2021 Asia-Pacific Signal and Information Processing Association Annual Summit and Conference (APSIPA ASC)(2021)
摘要
To realize robust End-to-end Automatic Speech Recognition (E2E ASR) under radio communication condition, we propose a multitask-based method to jointly train a Speech Enhancement (SE) module as the front-end and an E2E ASR model as the back-end in this paper. One of the advantages of the proposed method is that the entire system can be trained from scratch. Different from prior works, either component here doesn't need to perform pre-training and fine-tuning processes separately. Through analysis, we found that the success of the proposed method lies in the following aspects. First, multitask learning is essential, that is, the SE network is not only learned to produce more intelligible speech, it is also aimed to generate speech that is beneficial to recognition. Secondly, we also found speech phase preserved from noisy speech is critical for an improved ASR performance. Thirdly, we propose a dual-channel data augmentation training method to obtain further improve-ment. Specifically, we combine the clean and enhanced speech to train the whole system. We evaluate the proposed method on the RATS English data set, achieving a relative WER reduction of 4.6% with the joint training method, and up to a relative WER reduction of 11.2% with the proposed data augmentation method.
更多查看译文
关键词
End-to-End,Speech Enhancement,Automatic Speech Recognition,Multitask Learning,Joint Training,Conformer
AI 理解论文
溯源树
样例
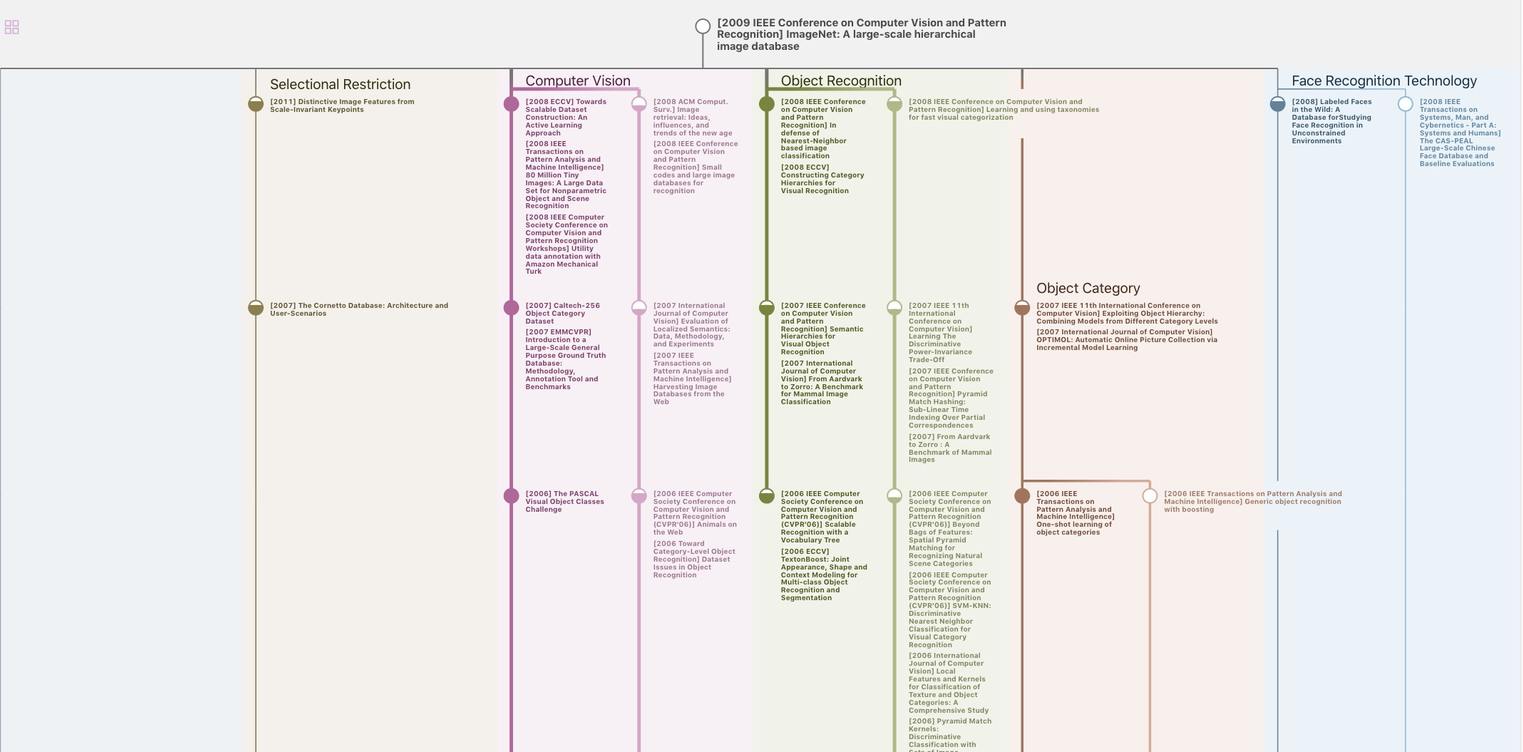
生成溯源树,研究论文发展脉络
Chat Paper
正在生成论文摘要