Online-Learning Deep Neuro-Adaptive Dynamic Inversion Controller for Model Free Control
arxiv(2021)
摘要
Adaptive methods are popular within the control literature due to the flexibility and forgiveness they offer in the area of modelling. Neural network adaptive control is favorable specifically for the powerful nature of the machine learning algorithm to approximate unknown functions and for the ability to relax certain constraints within traditional adaptive control. Deep neural networks are large framework networks with vastly superior approximation characteristics than their shallow counterparts. However, implementing a deep neural network can be difficult due to size specific complications such as vanishing/exploding gradients in training. In this paper, a neuro-adaptive controller is implemented featuring a deep neural network trained on a new weight update law that escapes the vanishing/exploding gradient problem by only incorporating the sign of the gradient. The type of controller designed is an adaptive dynamic inversion controller utilizing a modified state observer in a secondary estimation loop to train the network. The deep neural network learns the entire plant model on-line, creating a controller that is completely model free. The controller design is tested in simulation on a 2 link planar robot arm. The controller is able to learn the nonlinear plant quickly and displays good performance in the tracking control problem.
更多查看译文
关键词
model free controller,online-learning,neuro-adaptive
AI 理解论文
溯源树
样例
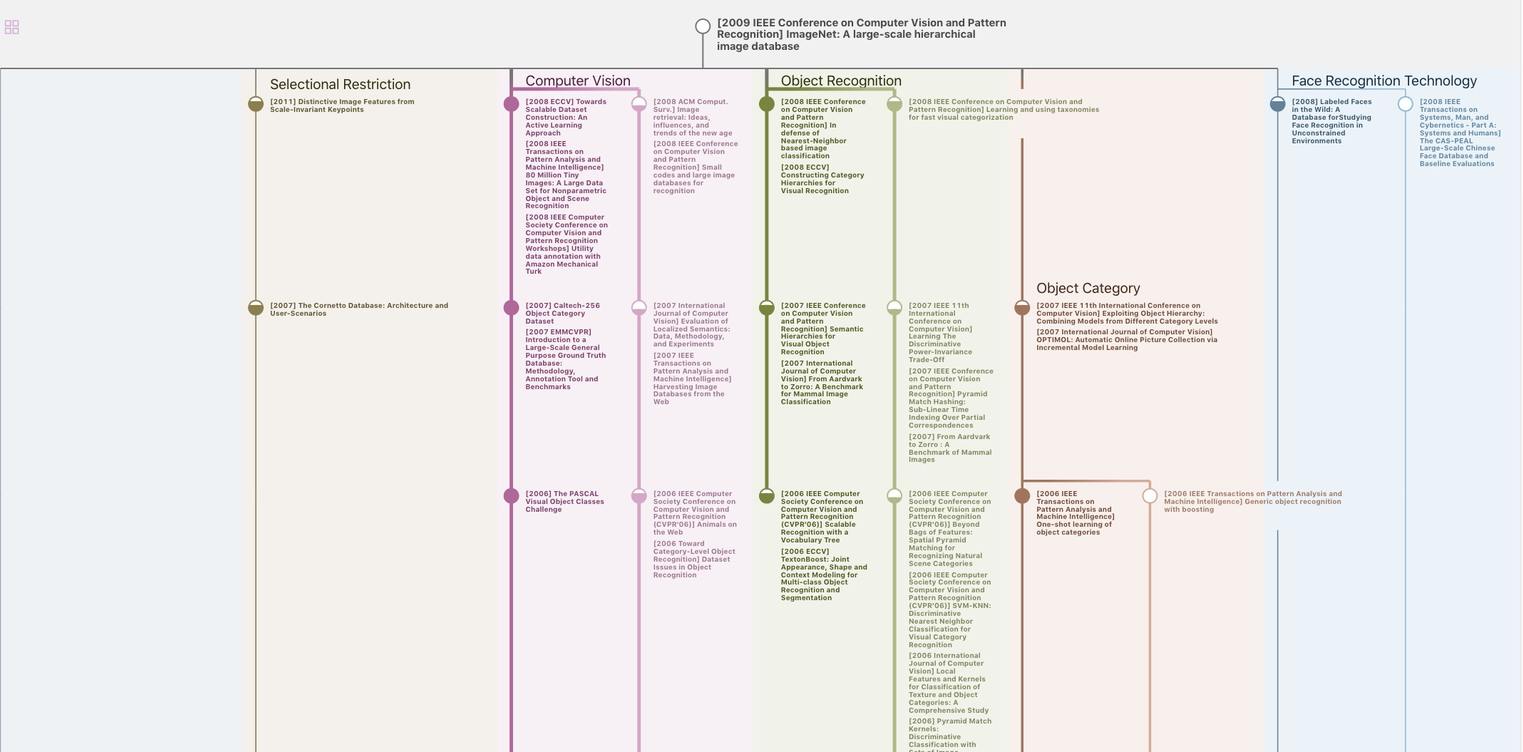
生成溯源树,研究论文发展脉络
Chat Paper
正在生成论文摘要