Predicting trajectory behaviour via machine-learned invariant manifolds
CHEMICAL PHYSICS LETTERS(2022)
摘要
In this paper, we use support vector machines (SVM) to develop a machine learning framework to discover phase space structures that distinguish between distinct reaction pathways. The SVM model is trained using data from trajectories of Hamilton's equations and works well even with relatively few trajectories. Moreover, this framework is specifically designed to require minimal a priori knowledge of the dynamics in a system. This makes our approach computationally better suited than existing methods for high-dimensional systems and systems where integrating trajectories is expensive. We benchmark our approach on Chesnavich's CH4+ Hamiltonian.
更多查看译文
关键词
Hamiltonian dynamics, Support vector machines, Phase space structures, Roaming, Ionmolecule reaction
AI 理解论文
溯源树
样例
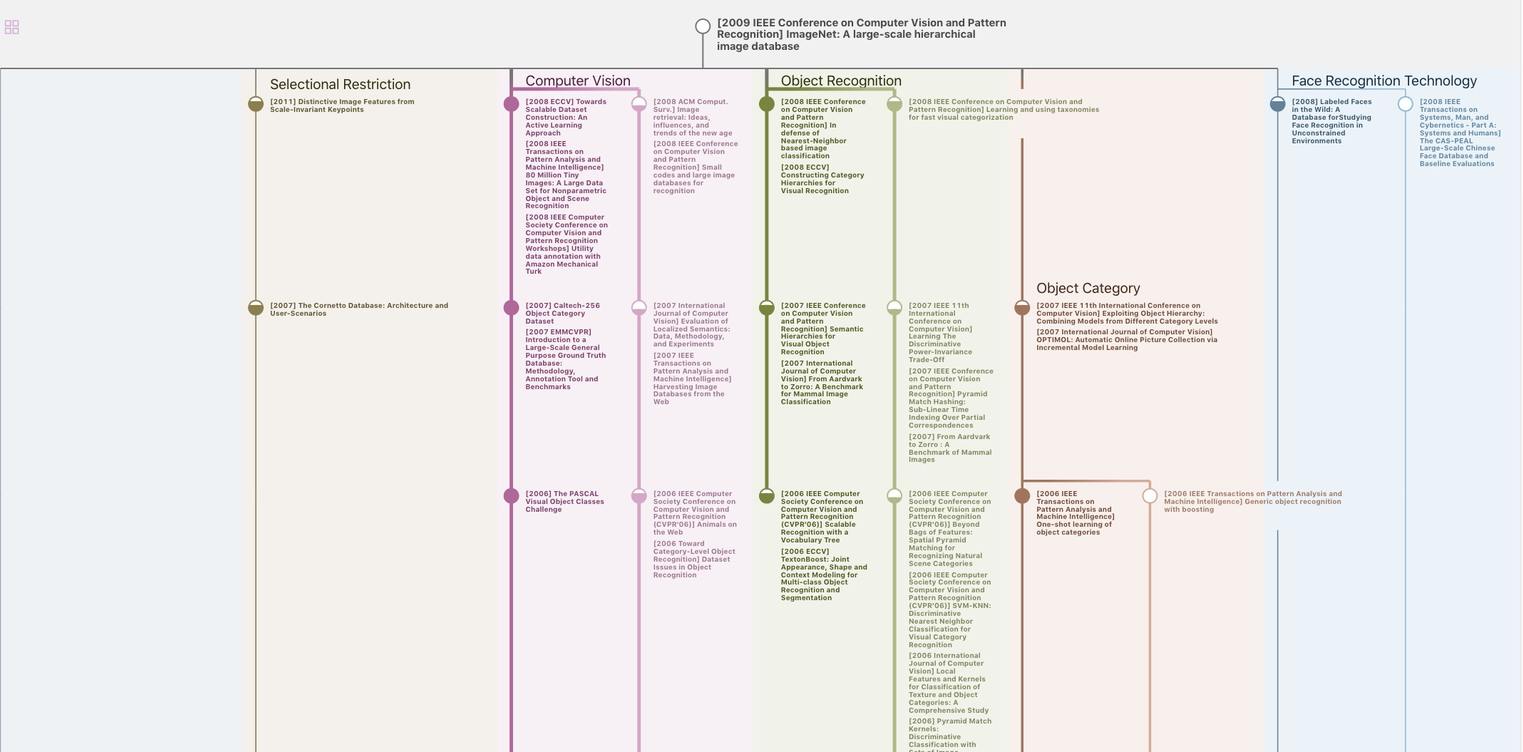
生成溯源树,研究论文发展脉络
Chat Paper
正在生成论文摘要