Learning Theorem Proving Components
AUTOMATED REASONING WITH ANALYTIC TABLEAUX AND RELATED METHODS, TABLEAUX 2021(2021)
摘要
Saturation-style automated theorem provers (ATPs) based on the given clause procedure are today the strongest general reasoners for classical first-order logic. The clause selection heuristics in such systems are, however, often evaluating clauses in isolation, ignoring other clauses. This has changed recently by equipping the E/ENIGMA system with a graph neural network (GNN) that chooses the next given clause based on its evaluation in the context of previously selected clauses. In this work, we describe several algorithms and experiments with ENIGMA, advancing the idea of contextual evaluation based on learning important components of the graph of clauses.
更多查看译文
关键词
Automated theorem proving, Machine learning, Neural networks, Decision trees, Saturation-style proving
AI 理解论文
溯源树
样例
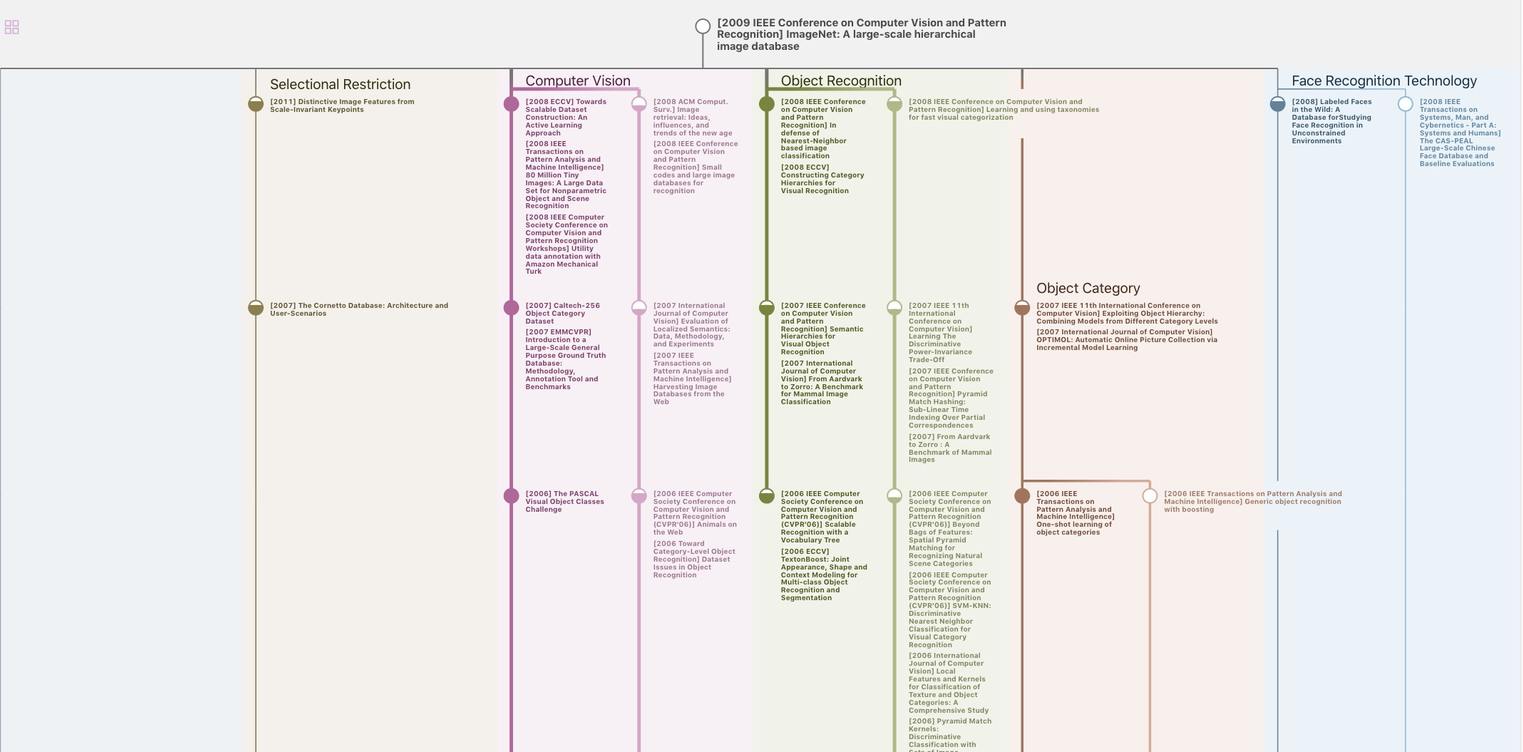
生成溯源树,研究论文发展脉络
Chat Paper
正在生成论文摘要